MAPLE: Masked Pseudo-Labeling autoEncoder for Semi-supervised Point Cloud Action Recognition
PROCEEDINGS OF THE 30TH ACM INTERNATIONAL CONFERENCE ON MULTIMEDIA, MM 2022(2022)
摘要
Recognizing human actions from point cloud videos has attracted tremendous attention from both academia and industry due to its wide applications like automatic driving, robotics, and so on. However, current methods for point cloud action recognition usually require a huge amount of data with manual annotations and a complex backbone network with high computation cost, which makes it impractical for real-world applications. Therefore, this paper considers the task of semi-supervised point cloud action recognition. We propose a Masked Pseudo-Labeling autoEncoder (MAPLE) framework to learn effective representations with much fewer annotations for point cloud action recognition. In particular, we design a novel and efficient Decoupled spatial-temporal TransFormer (DestFormer) as the backbone of MAPLE. In DestFormer, the spatial and temporal dimensions of the 4D point cloud videos are decoupled to achieve an efficient self-attention for learning both long-term and short-term features. Moreover, to learn discriminative features from fewer annotations, we design a masked pseudo-labeling autoencoder structure to guide the DestFormer to reconstruct features of masked frames from the available frames. More importantly, for unlabeled data, we exploit the pseudo-labels from the classification head as the supervision signal for the reconstruction of features from the masked frames. Finally, comprehensive experiments demonstrate that MAPLE achieves superior results on three public benchmarks and outperforms the state-of-the-art method by 8.08% accuracy on the MSR-Action3D dataset. (1)
更多查看译文
关键词
Point Cloud Action Recognition,Semi-supervised Learning,Autoencoder,Vision Transformer
AI 理解论文
溯源树
样例
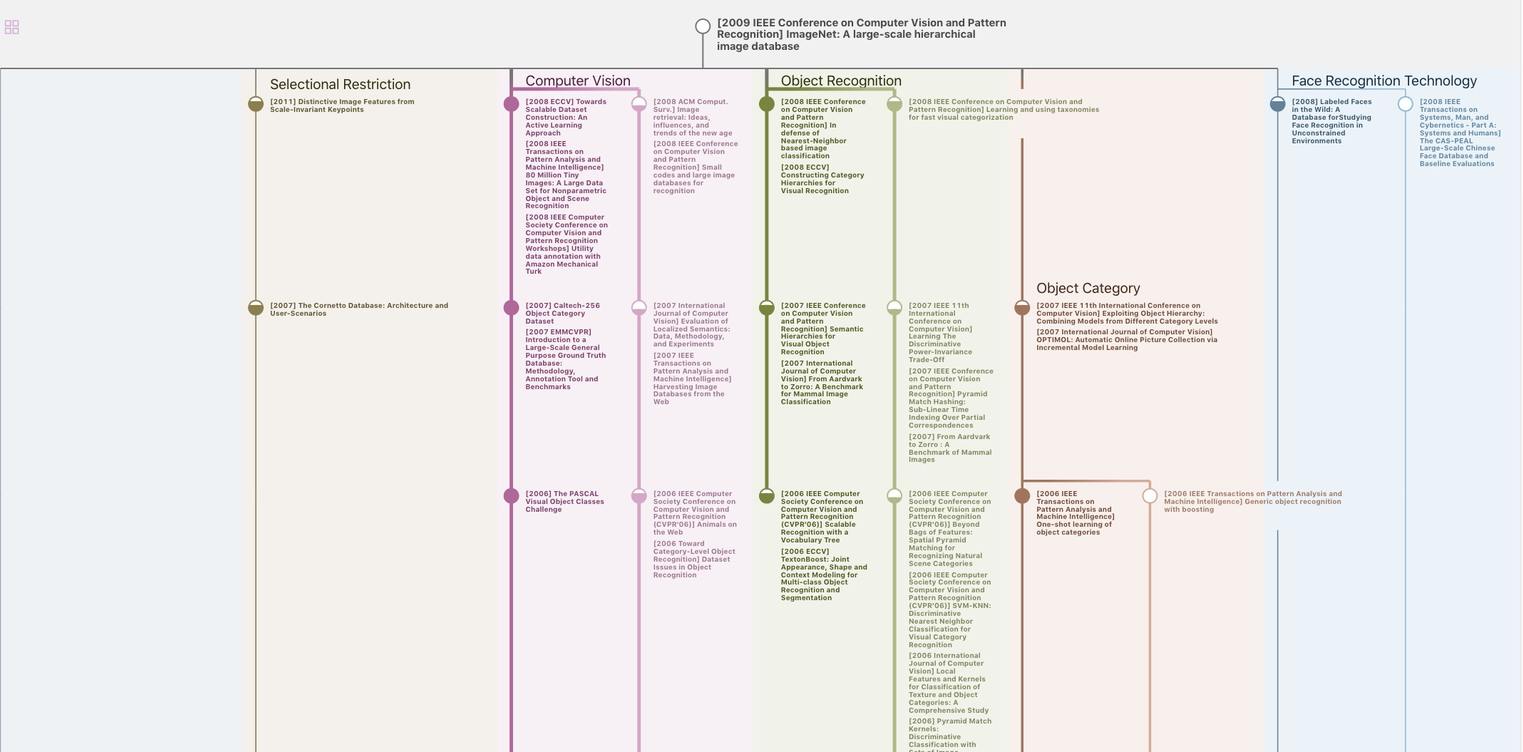
生成溯源树,研究论文发展脉络
Chat Paper
正在生成论文摘要