TokenCut: Segmenting Objects in Images and Videos With Self-Supervised Transformer and Normalized Cut
IEEE TRANSACTIONS ON PATTERN ANALYSIS AND MACHINE INTELLIGENCE(2023)
摘要
In this paper, we describe a graph-based algorithm that uses the features obtained by a self-supervised transformer to detect and segment salient objects in images and videos. With this approach, the image patches that compose an image or video are organised into a fully connected graph, in which the edge between each pair of patches is labeled with a similarity score based on the features learned by the transformer. Detection and segmentation of salient objects can then be formulated as a graph-cut problem and solved using the classical Normalized Cut algorithm. Despite the simplicity of this approach, it achieves state-of-the-art results on several common image and video detection and segmentation tasks. For unsupervised object discovery, this approach outperforms the competing approaches by a margin of 6.1%, 5.7%, and 2.6% when tested with the VOC07, VOC12, and COCO20 K datasets. For the unsupervised saliency detection task in images, this method improves the score for Intersection over Union (IoU) by 4.4%, 5.6% and 5.2%. When tested with the ECSSD, DUTS, and DUT-OMRON datasets. This method also achieves competitive results for unsupervised video object segmentation tasks with the DAVIS, SegTV2, and FBMS datasets.
更多查看译文
关键词
Unsupervised object discovery,unsupervised saliency detection,unsupervised video object segmentation,self-supervised transformer,normalized cut
AI 理解论文
溯源树
样例
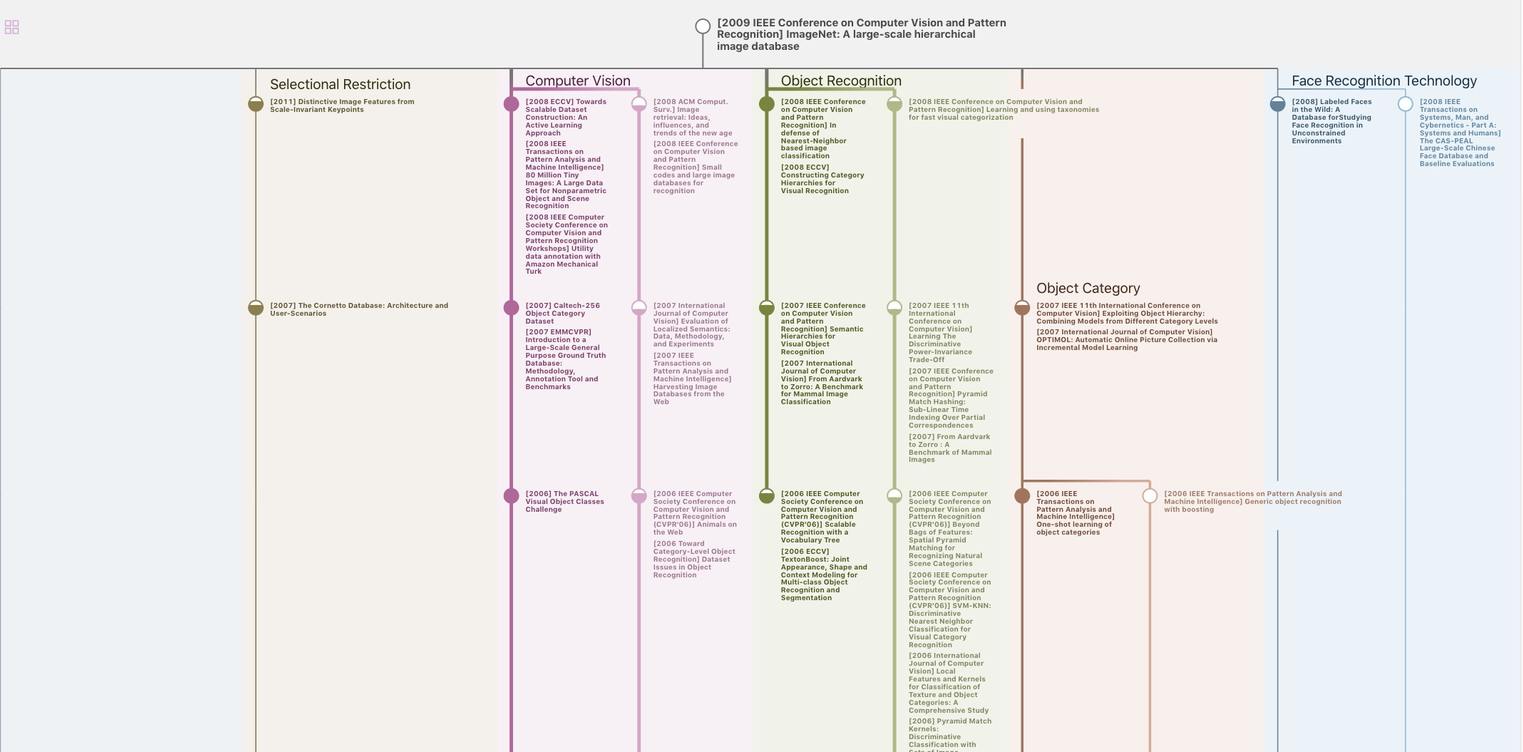
生成溯源树,研究论文发展脉络
Chat Paper
正在生成论文摘要