The Geometry and Calculus of Losses
JOURNAL OF MACHINE LEARNING RESEARCH(2023)
摘要
Statistical decision problems lie at the heart of statistical machine learning. The simplest problems are multiclass classification and class probability estimation. Central to their definition is the choice of loss function, which is the means by which the quality of a solution is evaluated. In this paper we systematically develop the theory of loss functions for such problems from a novel perspective whose basic ingredients are convex sets with a particular structure. The loss function is defined as the subgradient of the support function of the convex set. It is consequently automatically proper (calibrated for probability estimation). This perspective provides three novel opportunities. It enables the development of a fundamental relationship between losses and (anti)-norms that appears to have not been noticed before. Second, it enables the development of a calculus of losses induced by the calculus of convex sets which allows the interpolation between different losses, and thus is a potential useful design tool for tailoring losses to particular problems. In doing this we build upon, and considerably extend, existing results on M-sums of convex sets. Third, the perspective leads to a natural theory of "polar" loss functions, which are derived from the polar dual of the convex set defining the loss, and which form a natural universal substitution function for Vovk's aggregating algorithm.
更多查看译文
关键词
convex sets,support functions,gauges,polars,concave duality,proper loss functions,M-sums,distorted probabilities,polar losses,Shephard duality,anti-norms,Bregman divergences,semi inner products,Finsler geometry,aggregating algorithm,substitution functions,direct and inverse addition
AI 理解论文
溯源树
样例
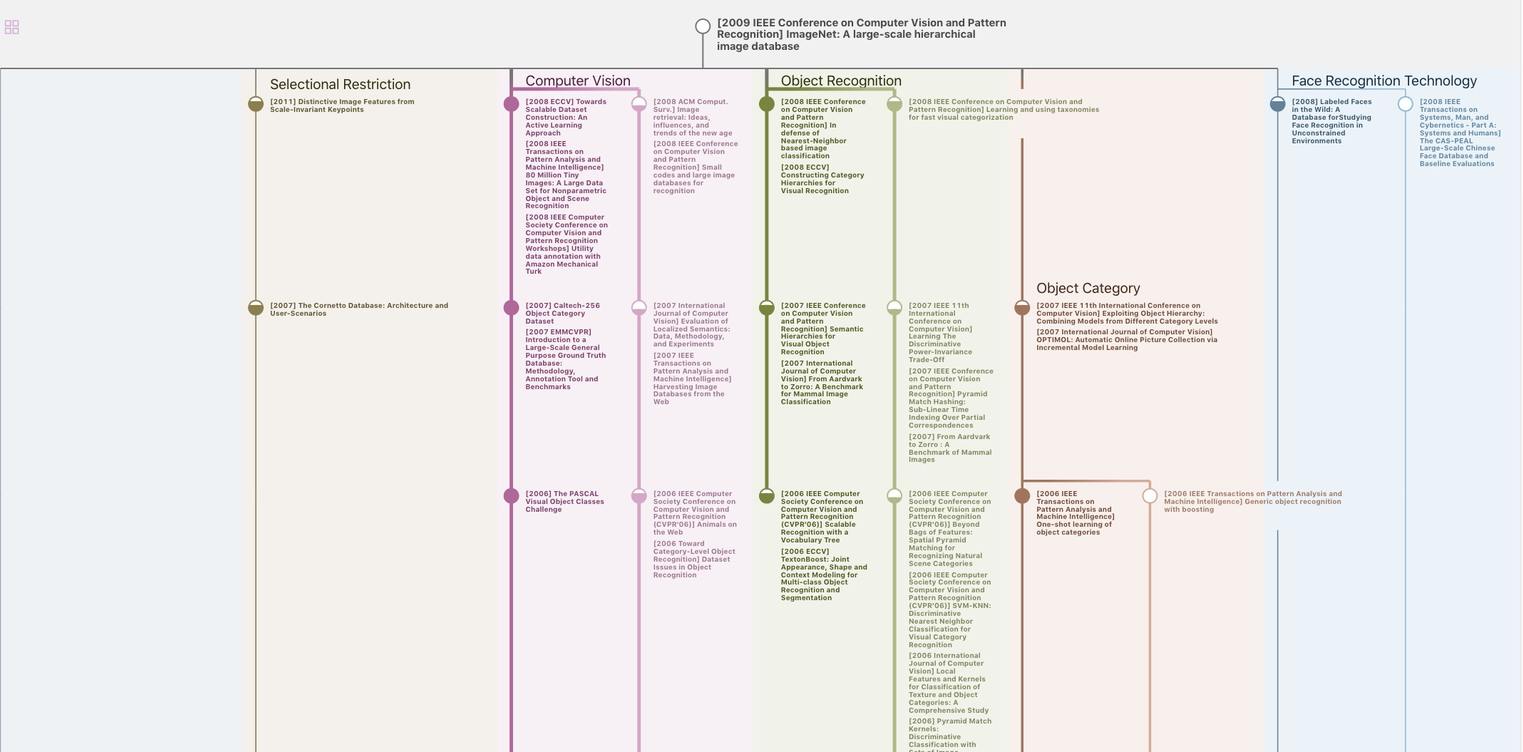
生成溯源树,研究论文发展脉络
Chat Paper
正在生成论文摘要