Surrogate tree ensemble model representing 2D population doses over complex terrain in the event of a radiological release into the air
Progress in Nuclear Energy(2023)
Abstract
Atmospheric dispersion models predict the dispersion of harmful substances in case of accidents at industrial facilities and nuclear power plants (NPPs). However, high computation time limits their usage in an emergency or long-term analyses. This paper reduces the computation time by designing a surrogate data-driven model using a grid of tree ensemble models as a surrogate for the physical model and meteorological station measurements as model regressors. Regression tree modelling provided information for selecting the most important variables for prediction, while model ensembles improved the prediction accuracy. The approach is tested for an NPP in complex terrain to predict spatial (2D) maps of population doses for 24 h after a radiological release. The average performance of 2D maps against the physical model is SMSE (Standardized Mean Square Error) < 0.5 and FMS (Figure of Merit in Space) > 0.5. The designed model performs very well in predicting the long-term mean and 95th percentile of population doses. The main shortcoming is the underestimation of very high doses. Performance is expected to be further improved by selecting training data using pattern selection techniques and potentially by alternative machine learning algorithms or interconnected models, which we intend to apply in future work.
MoreTranslated text
Key words
Atmospheric dispersion,Radiological release,Surrogate model,Tree ensembles,Complex terrain
AI Read Science
Must-Reading Tree
Example
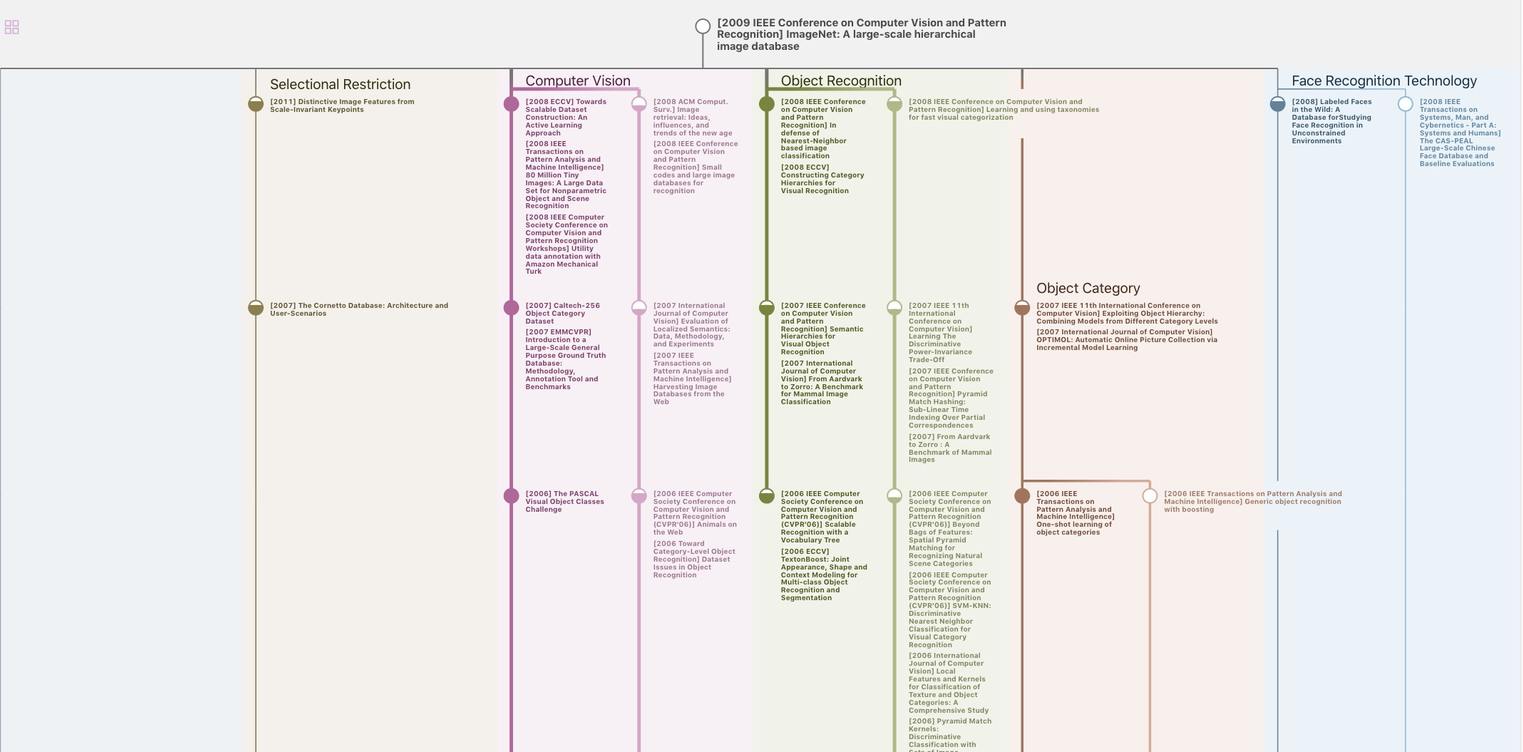
Generate MRT to find the research sequence of this paper
Chat Paper
Summary is being generated by the instructions you defined