Hybrid model for short-term wind power forecasting based on singular spectrum analysis and a temporal convolutional attention network with an adaptive receptive field
Energy Conversion and Management(2022)
Abstract
Accurate and robust short-term wind power forecasting (WPF) is of great significance to enhance the rate of renewable energy utilization in power systems and to promote low-carbon energy transformation. However, the high randomness and complex volatility of wind power bring great challenges when designing reliable and accurate forecasting models. In this paper, a novel hybrid model based on singular spectrum analysis (SSA) and a temporal convolutional attention network with an adaptive receptive field (ARFTCAN) is proposed. Specifically, to ensure the sufficiency and completeness of feature decomposition and reconstruction, we develop an SSAbased component partitioning mechanism to decompose complex original wind power sequences and determine their trend, period and noise components. Moreover, a self-attention mechanism and the adaptive receptive field (ARF) algorithm are integrated into a temporal convolutional network (TCN) to ensure the automatic extraction of multiple critical frequency-domain features within the complete fluctuation period. Furthermore, the forecasting results obtained with different feature components are integrated into the final model to realize identification, reconstruction and extrapolation from a multifrequency-domain perspective. The results demonstrate that the proposed model effectively supports the adaptability of short-term WPF in four seasons. Especially in scenarios with high-frequency wind power fluctuations, the mean absolute percentage error (MAPE) of the proposed model is reduced by more than 52% relative to those of the state-of-the-art decomposition-forecasting models. Moreover, compared to the classic SSA-based deep learning models, the proposed model achieves an MAPE reduction of over 13% in a scenario with low power output.
MoreTranslated text
Key words
Wind power forecasting,Temporal convolutional attention network,Singular spectrum analysis,Adaptive receptive field algorithm
AI Read Science
Must-Reading Tree
Example
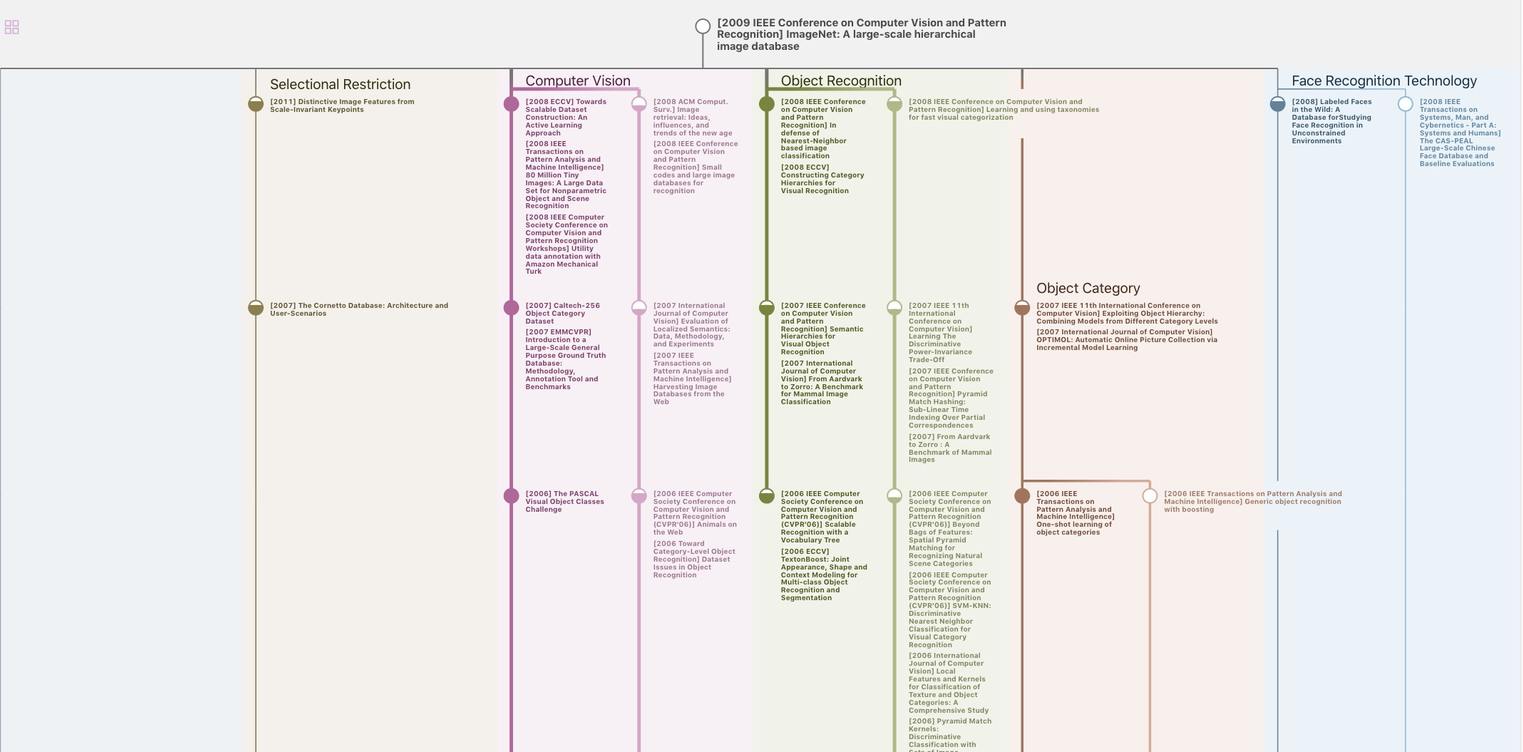
Generate MRT to find the research sequence of this paper
Chat Paper
Summary is being generated by the instructions you defined