An invariances-preserving vector basis neural network for the closure of Reynolds-averaged Navier-Stokes equations by the divergence of the Reynolds stress tensor
Physics of Fluids(2022)
摘要
In the present paper, a new data-driven model is proposed to close and increase accuracy of Reynolds-averaged Navier-Stokes equations. Among the variety of turbulent quantities, it has been decided to predict the divergence of the Reynolds stress tensor (RST). Recent literature works highlighted the potential of this choice. The key novelty of this work is to obtain the divergence of the Reynolds stress tensor through a neural network (NN) whose architecture and input choice guarantee both Galilean and coordinates-frame rotation. The former derives from the input choice of the NN while the latter from the expansion of the divergence of the RST into a vector basis. This approach has been widely used for data-driven models for the RST anisotropy or the RST discrepancies but surprisingly not for the divergence of the RST. The present paper tries to fill this literature gap. Hence, a constitutive relation of the divergence of the RST from mean quantities is proposed to obtain such expansion. Moreover, once the proposed data-driven approach is trained, there is no need to run any classic turbulence model to close the equations. The well-known tests of flow in a square duct and over periodic hills are used to show advantages of the present method compared to the standard turbulence models.
更多查看译文
关键词
navier–stokes equations,neural network,invariances-preserving,reynolds-averaged
AI 理解论文
溯源树
样例
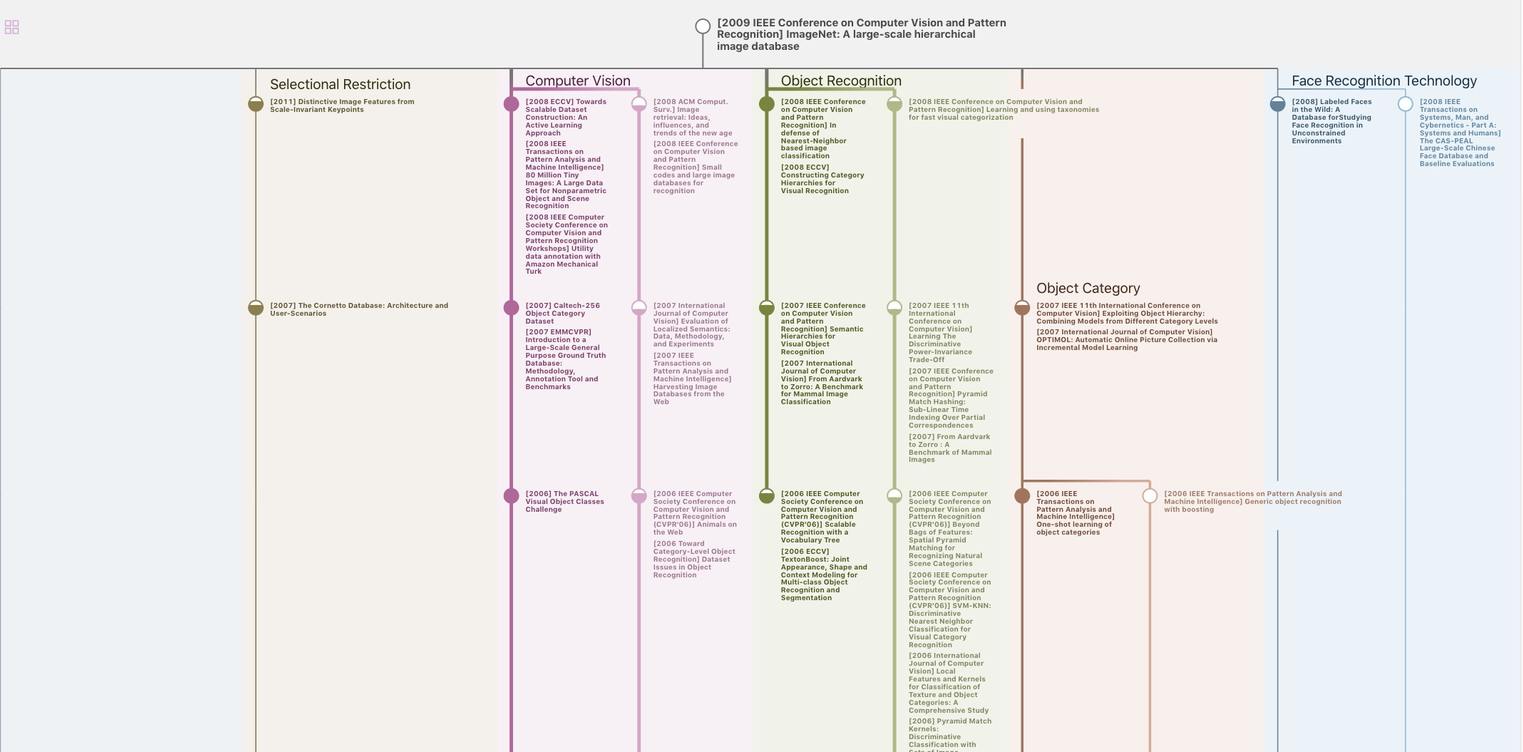
生成溯源树,研究论文发展脉络
Chat Paper
正在生成论文摘要