Global Search and Analysis for the Nonconvex Two-Level l 1 Penalty.
IEEE transactions on neural networks and learning systems(2022)
摘要
Imposing suitably designed nonconvex regularization is effective to enhance sparsity, but the corresponding global search algorithm has not been well established. In this article, we propose a global search algorithm for the nonconvex two-level l penalty based on its piecewise linear property and apply it to machine learning tasks. With the search capability, the optimization performance of the proposed algorithm could be improved, resulting in better sparsity and accuracy than most state-of-the-art global and local algorithms. Besides, we also provide an approximation analysis to demonstrate the effectiveness of our global search algorithm in sparse quantile regression.
更多查看译文
关键词
Compressive sensing,global search algorithm,kernel-based quantile regression,nonconvex optimization,two-level l(1) penalty
AI 理解论文
溯源树
样例
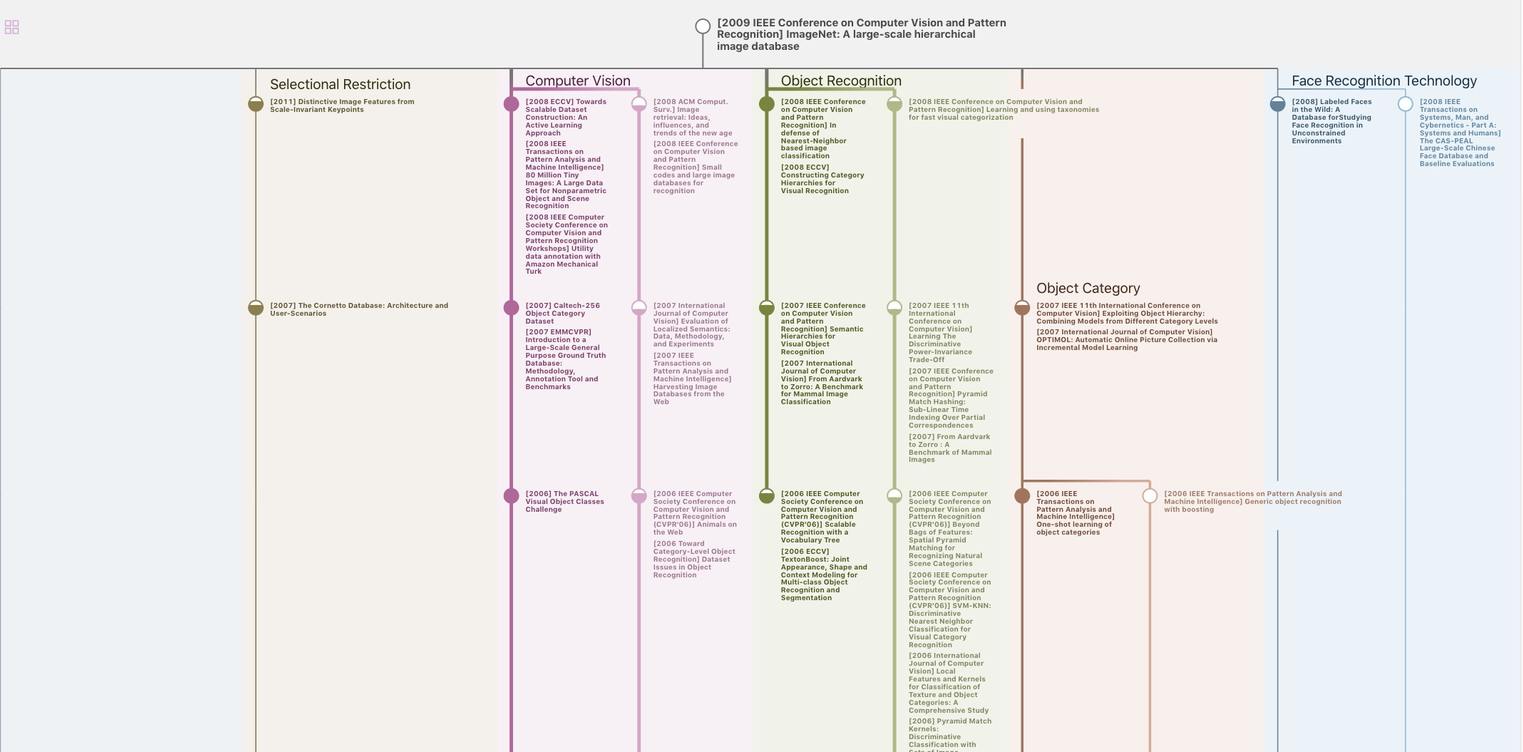
生成溯源树,研究论文发展脉络
Chat Paper
正在生成论文摘要