Variable selection in sparse multivariate GLARMA models: Application to germination control by environment
HAL (Le Centre pour la Communication Scientifique Directe)(2022)
Abstract
We propose a novel and efficient iterative two-stage variable selection approach for multivariate sparse GLARMA models, which can be used for modelling multivariate discrete-valued time series. Our approach consists in iteratively combining two steps: the estimation of the autoregressive moving average (ARMA) coefficients of multivariate GLARMA models and the variable selection in the coefficients of the Generalized Linear Model (GLM) part of the model performed by regularized methods. We explain how to implement our approach efficiently. Then we assess the performance of our methodology using synthetic data and compare it with alternative methods. Finally, we illustrate it on RNA-Seq data resulting from polyribosome profiling to determine translational status for all mRNAs in germinating seeds. Our approach, which is implemented in the MultiGlarmaVarSel R package and available on the CRAN, is very attractive since it benefits from a low computational load and is able to outperform the other methods for recovering the null and non-null coefficients.
MoreTranslated text
Key words
germination control by environment,glarma models,variable selection
AI Read Science
Must-Reading Tree
Example
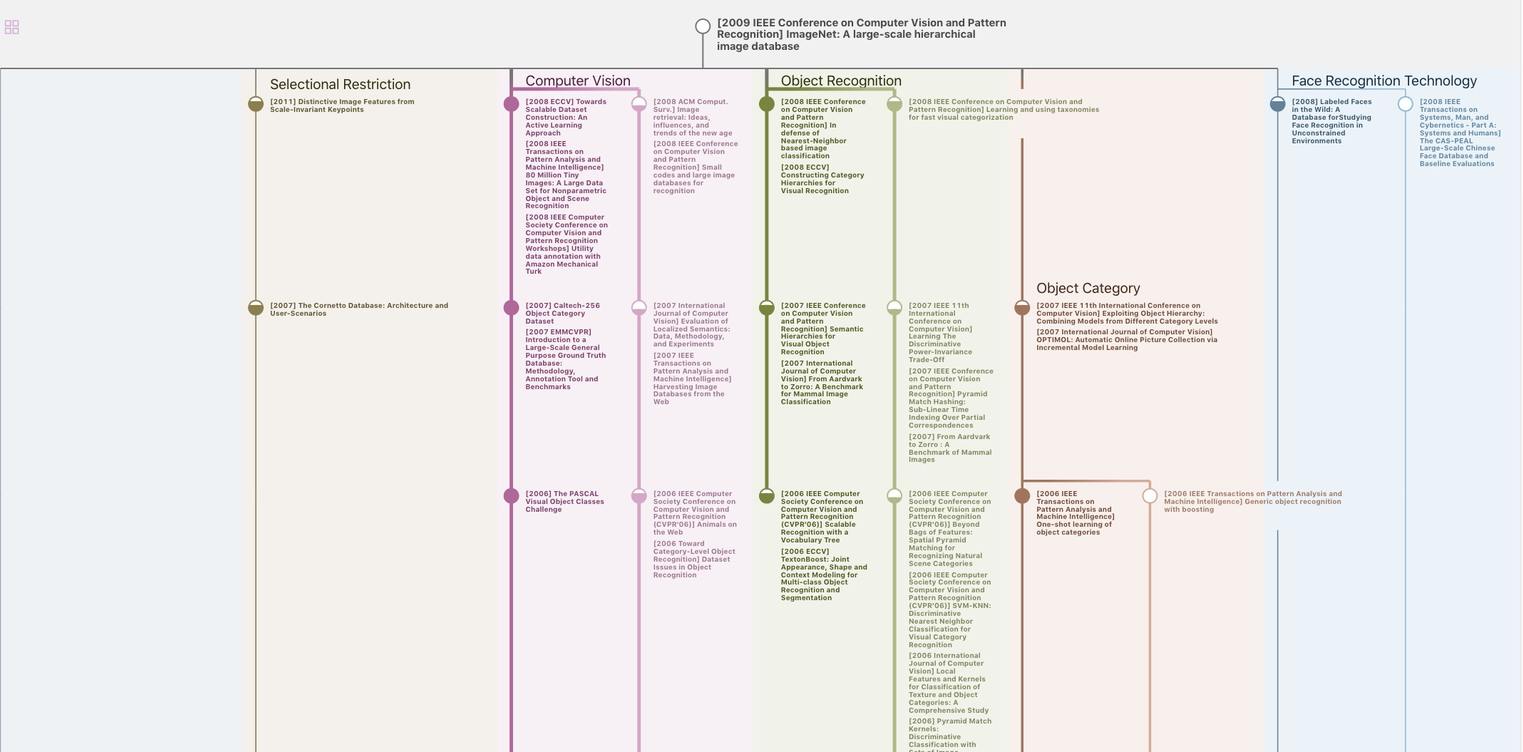
Generate MRT to find the research sequence of this paper
Chat Paper
Summary is being generated by the instructions you defined