Injecting Image Details into CLIP's Feature Space
ICLR 2023(2022)
摘要
Although CLIP-like Visual Language Models provide a functional joint feature space for image and text, due to the limitation of the CILP-like model's image input size (e.g., 224), subtle details are lost in the feature representation if we input high-resolution images (e.g., 2240). In this work, we introduce an efficient framework that can produce a single feature representation for a high-resolution image that injects image details and shares the same semantic space as the original CLIP. In the framework, we train a feature fusing model based on CLIP features extracted from a carefully designed image patch method that can cover objects of any scale, weakly supervised by image-agnostic class prompted queries. We validate our framework by retrieving images from class prompted queries on the real world and synthetic datasets, showing significant performance improvement on these tasks. Furthermore, to fully demonstrate our framework's detail retrieval ability, we construct a CLEVR-like synthetic dataset called CLVER-DS, which is fully annotated and has a controllable object scale.
更多查看译文
关键词
Text-Based Information Retrieval,Fine-Detail,CLIP,Single Feature,Detail Compression,Complete Cover,Feature Space Alignment,Self-Supervised
AI 理解论文
溯源树
样例
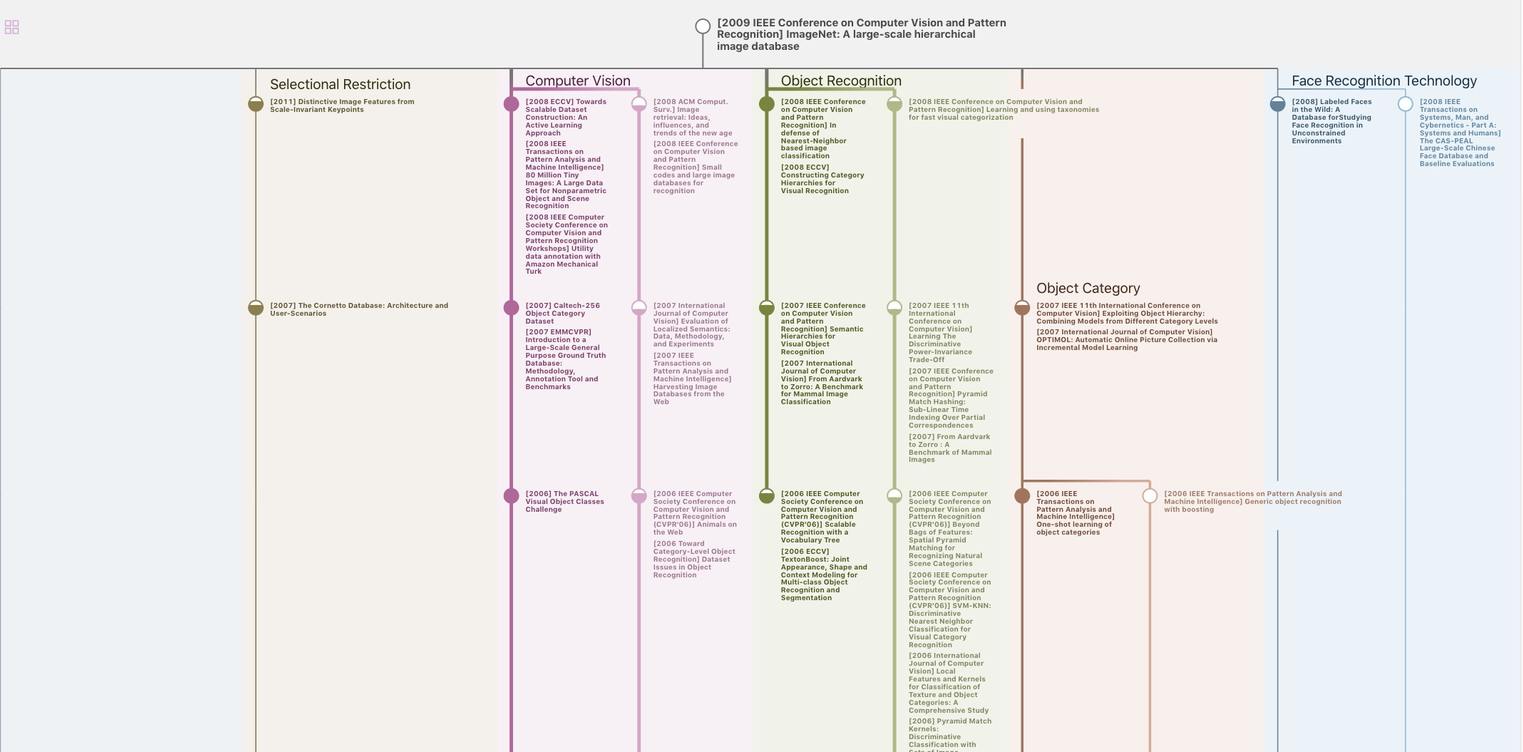
生成溯源树,研究论文发展脉络
Chat Paper
正在生成论文摘要