Deep Spatio-Temporal Attention-Based Recurrent Network From Dynamic Adaptive Functional Connectivity for MCI Identification
IEEE TRANSACTIONS ON NEURAL SYSTEMS AND REHABILITATION ENGINEERING(2022)
摘要
Most existing methods of constructing dynamic functional connectivity (dFC) network obtain the connectivity strength via the sliding window correlation (SWC) method, which estimates the connectivity strength at each time segment, rather than at each time point, and thus is difficult to produce accurate dFC network due to the influence of the window type and window width. Furthermore, the deep learning methods may not capture the discriminative spatio-temporal information that is closely related to disease, thus impacting the performance of mild cognitive impairment (MCI) identification. In this paper, a novel spatio-temporal attention-based bidirectional gated recurrent unit (STA-BiGRU) network is proposed to extract inherent spatio-temporal information from a dynamic adaptive functional connectivity (dAFC) network for MCI diagnosis. Specifically, we adopt a group lasso-based Kalman filter algorithm to obtain the dAFC network with more accurate connectivity strength at each time step. Then a spatial attention module with self-attention and a temporal attention module with multiple temporal attention vectors are incorporated into the BiGRU network to extract more discriminative disease-related spatio-temporal information. Finally, the spatio-temporal regularizations are employed to better guide the attention learning of STA-BiGRU network to enhance the robustness of the deep network. Experimental results show that the proposed framework achieves mean accuracies of 90.2%, 90.0%, and 81.5%, respectively, for three MCI classification tasks. This study provides a more effective deep spatio-temporal attention-based recurrent network and obtains good performance and interpretability of deep learning for psychiatry diagnosis research.
更多查看译文
关键词
Data mining,Time series analysis,Heuristic algorithms,Feature extraction,Deep learning,Adaptive systems,Diseases,Dynamic adaptive functional connectivity (dAFC),computer aided analysis,attention,spatio-temporal information,functional MRI
AI 理解论文
溯源树
样例
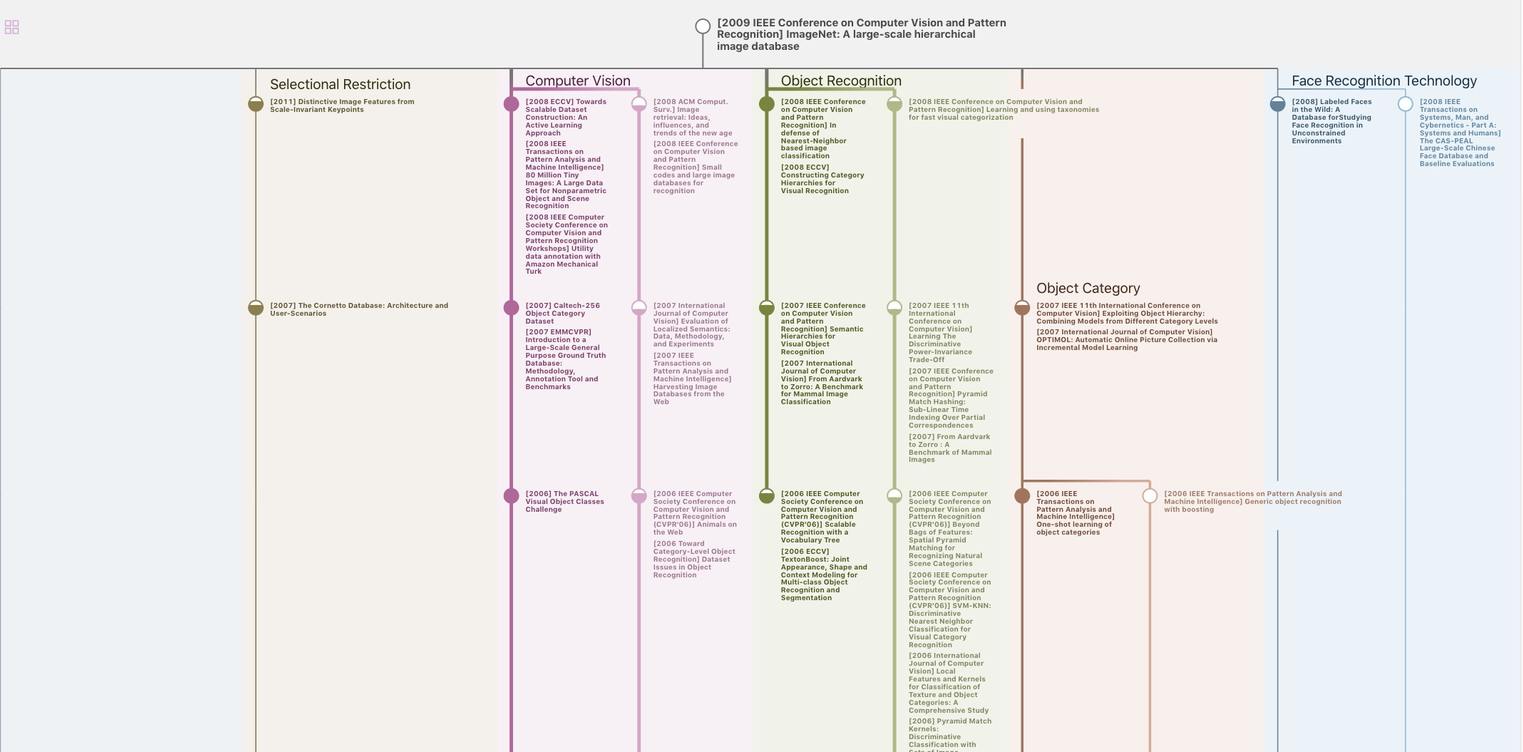
生成溯源树,研究论文发展脉络
Chat Paper
正在生成论文摘要