Boosting Night-Time Scene Parsing With Learnable Frequency
IEEE transactions on image processing : a publication of the IEEE Signal Processing Society(2023)
摘要
Night-Time Scene Parsing (NTSP) is essential to many vision applications, especially for autonomous driving. Most of the existing methods are proposed for day-time scene parsing. They rely on modeling pixel intensity-based spatial contextual cues under even illumination. Hence, these methods do not perform well in night-time scenes as such spatial contextual cues are buried in the over-/under-exposed regions in night-time scenes. In this paper, we first conduct an image frequency-based statistical experiment to interpret the day-time and night-time scene discrepancies. We find that image frequency distributions differ significantly between day-time and night-time scenes, and understanding such frequency distributions is critical to NTSP problem. Based on this, we propose to exploit the image frequency distributions for night-time scene parsing. First, we propose a Learnable Frequency Encoder (LFE) to model the relationship between different frequency coefficients to measure all frequency components dynamically. Second, we propose a Spatial Frequency Fusion module (SFF) that fuses both spatial and frequency information to guide the extraction of spatial context features. Extensive experiments show that our method performs favorably against the state-of-the-art methods on the NightCity, NightCity+ and BDD100K-night datasets. In addition, we demonstrate that our method can be applied to existing day-time scene parsing methods and boost their performance on night-time scenes. The code is available at
https://github.com/wangsen99/FDLNet
.
更多查看译文
关键词
Night-time vision,scene parsing,frequency analysis
AI 理解论文
溯源树
样例
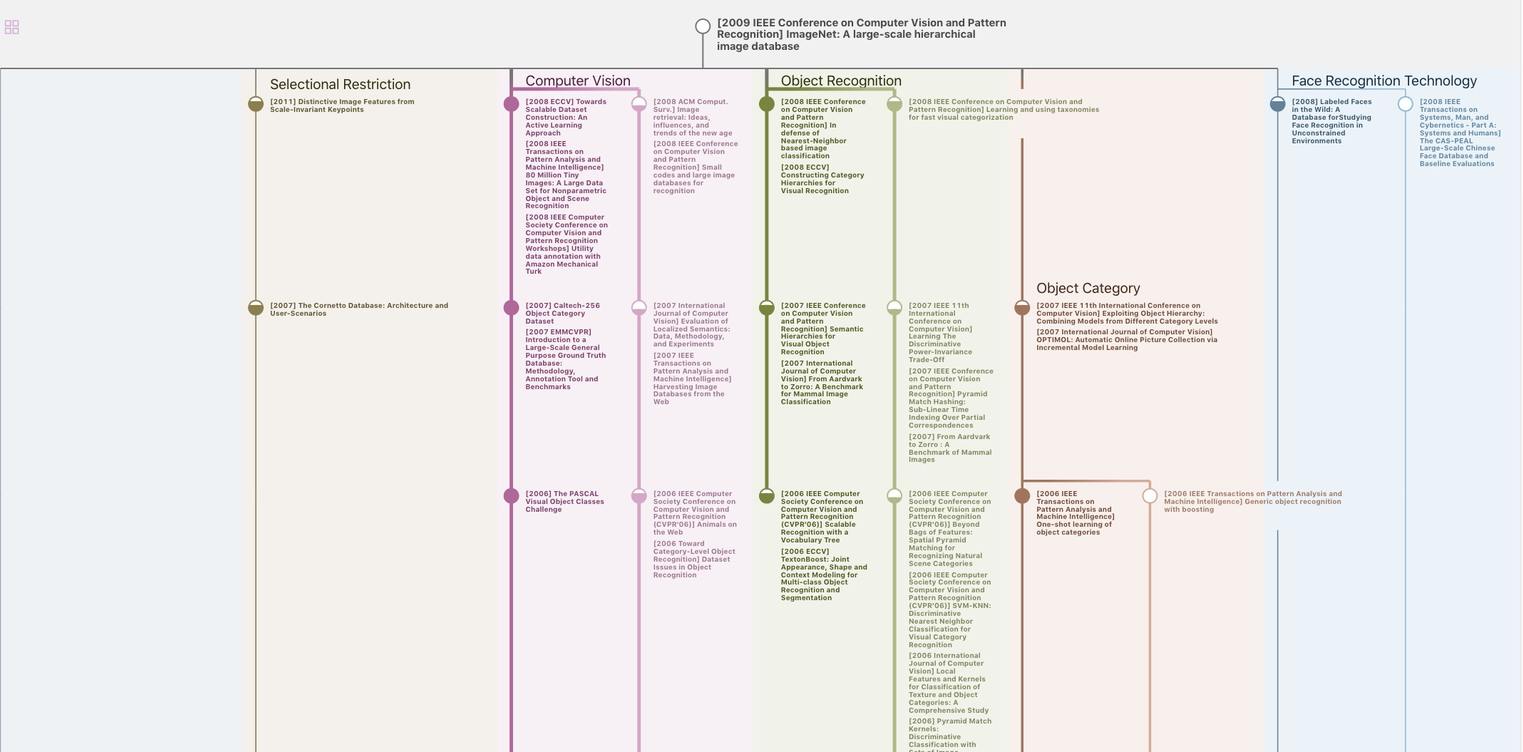
生成溯源树,研究论文发展脉络
Chat Paper
正在生成论文摘要