RAGUEL: Recourse-Aware Group Unfairness Elimination
Conference on Information and Knowledge Management(2022)
摘要
ABSTRACTWhile machine learning and ranking-based systems are in widespread use for sensitive decision-making processes (e.g., determining job candidates, assigning credit scores), they are rife with concerns over unintended biases in their outcomes, which makes algorithmic fairness (e.g., demographic parity, equal opportunity) an objective of interest. 'Algorithmic recourse' offers feasible recovery actions to change unwanted outcomes through the modification of attributes. We introduce the notion of ranked group-level recourse fairness, and develop a 'recourse-aware ranking' solution that satisfies ranked recourse fairness constraints while minimizing the cost of suggested modifications. Our solution suggests interventions that can reorder the ranked list of database records and mitigate group-level unfairness; specifically, disproportionate representation of sub-groups and recourse cost imbalance. This re-ranking identifies the minimum modifications to data points, with these attribute modifications weighted according to their ease of recourse. We then present an efficient block-based extension that enables re-ranking at any granularity (e.g., multiple brackets of bank loan interest rates, multiple pages of search engine results). Evaluation on real datasets shows that, while existing methods may even exacerbate recourse unfairness, our solution – RAGUEL – significantly improves recourse-aware fairness. RAGUEL outperforms alternatives at improving recourse fairness, through a combined process of counterfactual generation and re-ranking, whilst remaining efficient for large-scale datasets.
更多查看译文
关键词
Fairness, Ranking, Algorithmic Recourse, Recourse-Aware Ranking, Classification, Machine Learning
AI 理解论文
溯源树
样例
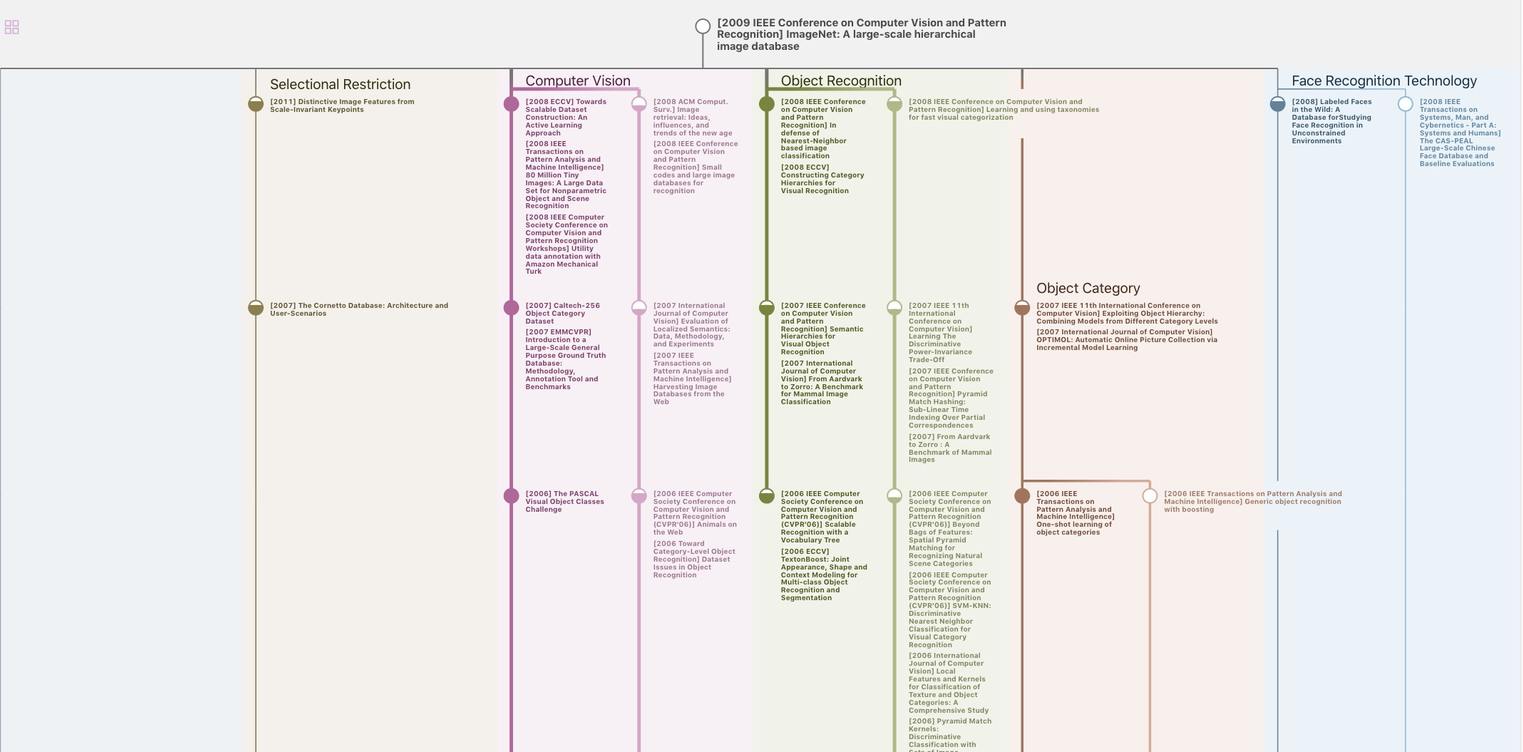
生成溯源树,研究论文发展脉络
Chat Paper
正在生成论文摘要