Infrastructure platform for privacy-preserving distributed machine learning development of computer-assisted theragnostics in cancer
JOURNAL OF BIOMEDICAL INFORMATICS(2022)
Abstract
Introduction: Emerging evidence suggests that data-driven support tools have found their way into clinical decision-making in a number of areas, including cancer care. Improving them and widening their scope of availability in various differing clinical scenarios, including for prognostic models derived from retrospective data, requires co-ordinated data sharing between clinical centres, secondary analyses of large multi-institutional clinical trial data, or distributed (federated) learning infrastructures. A systematic approach to utilizing routinely collected data across cancer care clinics remains a significant challenge due to privacy, administrative and po-litical barriers.Methods: An information technology infrastructure and web service software was developed and implemented which uses machine learning to construct clinical decision support systems in a privacy-preserving manner across datasets geographically distributed in different hospitals. The infrastructure was deployed in a network of Australian hospitals. A harmonized, international ontology-linked, set of lung cancer databases were built with the routine clinical and imaging data at each centre. The infrastructure was demonstrated with the development of logistic regression models to predict major cardiovascular events following radiation therapy.Results: The infrastructure implemented forms the basis of the Australian computer-assisted theragnostics (AusCAT) network for radiation oncology data extraction, reporting and distributed learning. Four radiation oncology departments (across seven hospitals) in New South Wales (NSW) participated in this demonstration study. Infrastructure was deployed at each centre and used to develop a model predicting for cardiovascular admission within a year of receiving curative radiotherapy for non-small cell lung cancer. A total of 10,417 lung cancer patients were identified with 802 being eligible for the model. Twenty features were chosen for analysis from the clinical record and linked registries. After selection, 8 features were included and a logistic regression model achieved an area under the receiver operating characteristic (AUROC) curve of 0.70 and C-index of 0.65 on out-of-sample data.Conclusion: The infrastructure developed was demonstrated to be usable in practice between clinical centres to harmonize routinely collected oncology data and develop models with federated learning. It provides a prom-ising approach to enable further research studies in radiation oncology using real world clinical data.
MoreTranslated text
Key words
Data mining,Decision support systems,Distributed learning,Federated learning,Machine learning,Radiation oncology
AI Read Science
Must-Reading Tree
Example
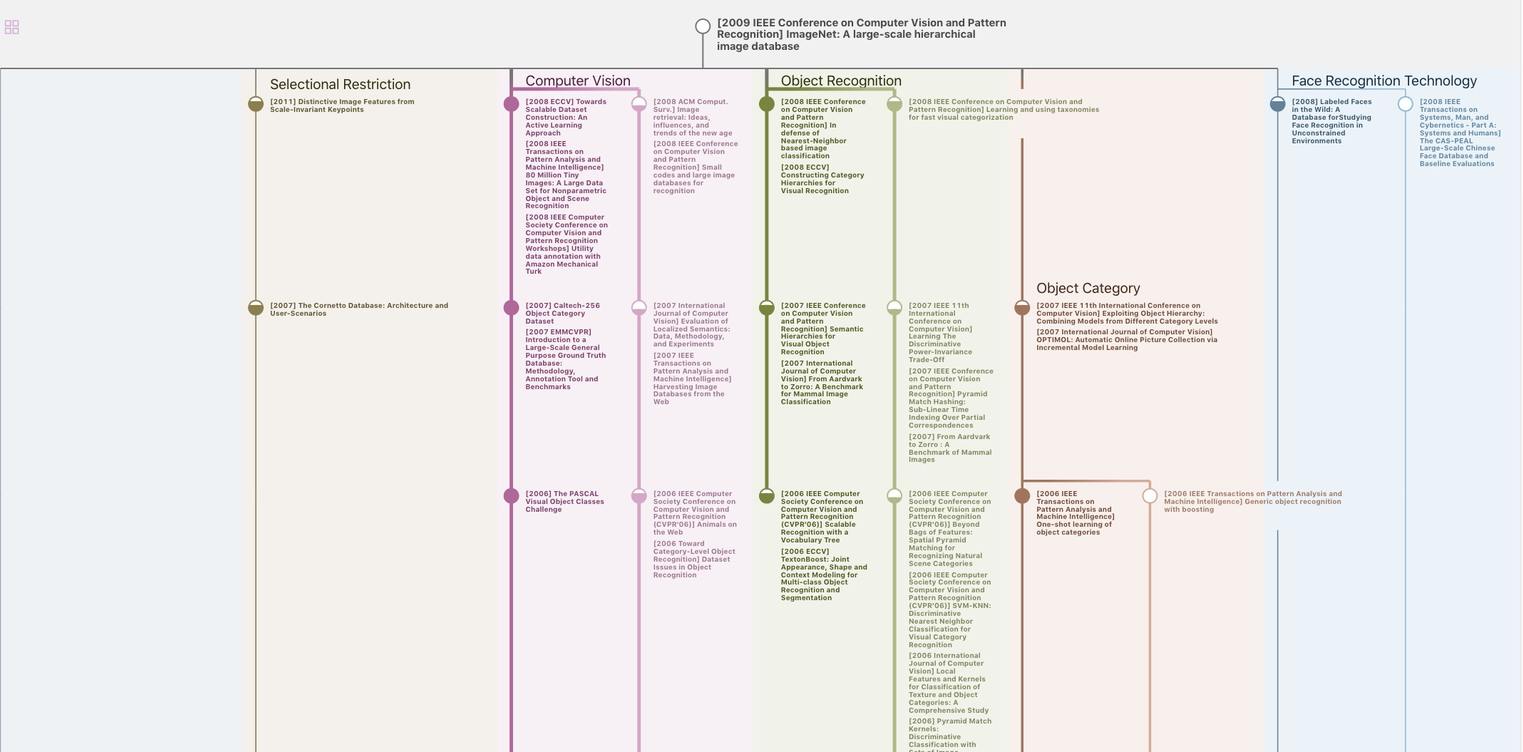
Generate MRT to find the research sequence of this paper
Chat Paper
Summary is being generated by the instructions you defined