Uncertain and biased facial expression recognition based on depthwise separable convolutional neural network with embedded attention mechanism
Journal of Electronic Imaging(2022)
摘要
Datasets play a crucial role in the development of facial expression recognition (FER), but most of the datasets suffer from obvious uncertainties and biases caused by different cultures and collection conditions. To look deeper into these issues, this paper first conducts two sets of experiments, face detection, and facial expression (FE) classification. They are based on three datasets (CK+, FER2013, and RAF-DB), which are collected from lab and wild environments. This paper proposes a network, depthwise separable convolutional neural network (CNN) with an embedded attention mechanism (DSA-CNN) for expression recognition. First, at the preprocessing stage, we obtain the maximum expression range clipping, which is calculated from 81 facial landmark points to filter nonface interferences. Then, we use DSA-CNN, which is based on a coordinate squeeze-and-excitation (CSE) attention for feature extraction. Finally, to further deal with imbalanced class biases and uncertainties issues, this paper proposes a class-weighted cross-entropy loss (CCE-loss) to alleviate the imbalance among seven emotional classes. Then, we combine CCE-loss with ranking regularization loss (RR-loss) and self-importance weighting cross-entropy loss (SCE-loss) at label amend stage, to jointly guide the training of the network. Extensive experiments on three FER datasets demonstrate that our proposed method outperforms most of the state-of-the-art methods eventually.
更多查看译文
关键词
facial expressions recognition, maximum expression range clipping, coordinate squeeze-and-excitation attention, class-weighted cross-entropy loss, dataset biases
AI 理解论文
溯源树
样例
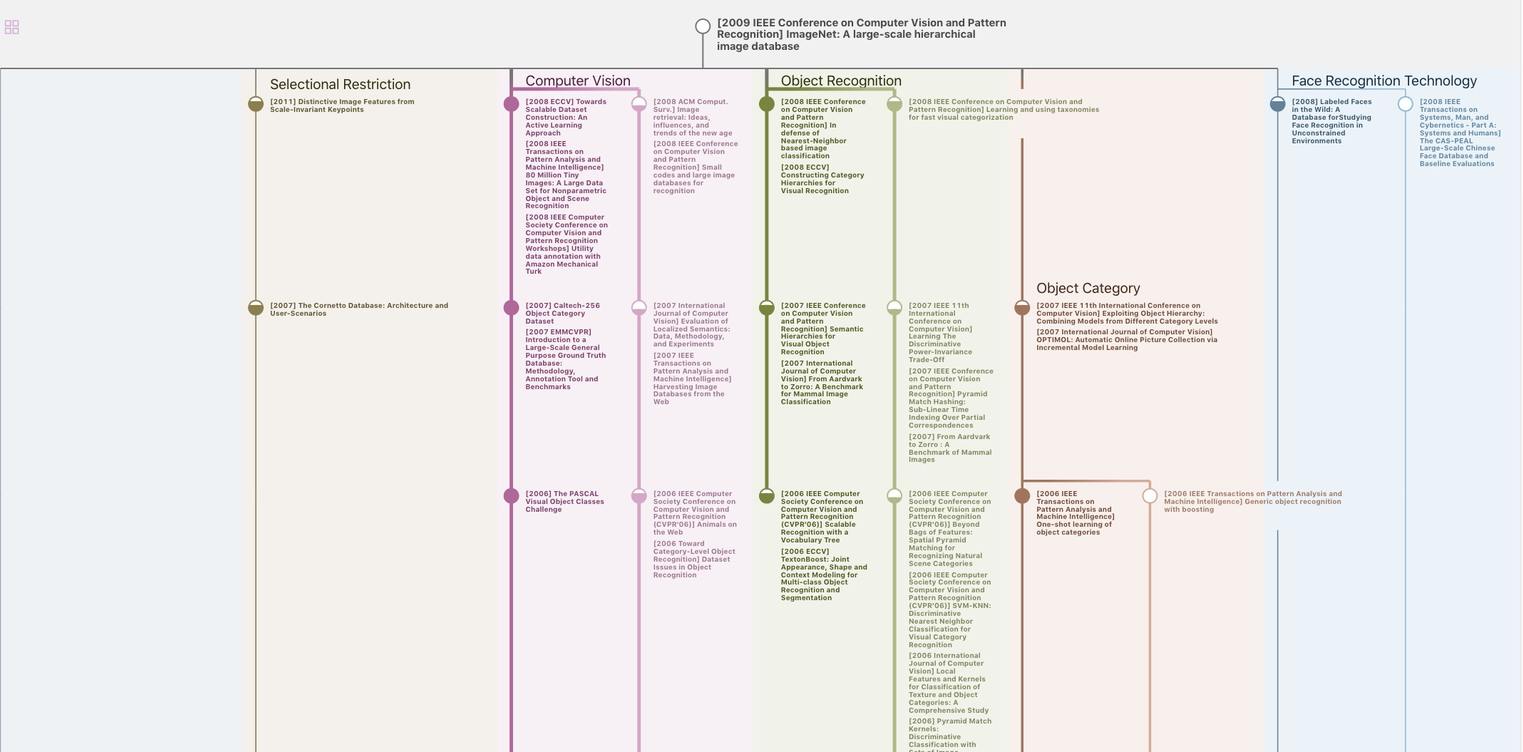
生成溯源树,研究论文发展脉络
Chat Paper
正在生成论文摘要