Exploring Skin Conductance Features for Cross-Subject Emotion Recognition
2022 IEEE Region 10 Symposium (TENSYMP)(2022)
Abstract
Human emotion recognition is an important research problem in various fields like human-computer interactions, learning, marketing etc. Physiological signals like galvanic skin response, heart rate, brain activation, respiration etc are being used for this. Simultaneous measurement of these signals is quite impractical in real-life scenarios. In this work, we have used Galvanic skin response (GSR) signal for emotion detection as there are many wearable devices available in the market that can be used to record GSR data with minimum obtrusion. We have used publicly available ASCERTAIN dataset containing physiological recordings from participants while watching affective movie clips. Variety of features have been used in literature for emotion recognition but deriving a feature set that works well across datasets is still an issue. We have used an exhaustive list of skin conductance features and used a two step feature selection method to identify most discriminating features for emotional arousal and valence classification. With proposed feature set, we achieved high f-scores of 0.7 for arousal and 0.8 for valence which are better compared to the performance of state-of-the-art emotion recognition approaches. Proposed feature sets were also used to classify valence and arousal levels of another publicly available CASE dataset. Average classification accuracy obtained on CASE dataset are 88% for arousal and 86% for valence which also outperforms state-of-the-art results. These results show that our recommended features perform well across datasets and hence can be used for cross-subject emotion classification.
MoreTranslated text
Key words
skin conductance features,emotion,recognition,cross-subject
AI Read Science
Must-Reading Tree
Example
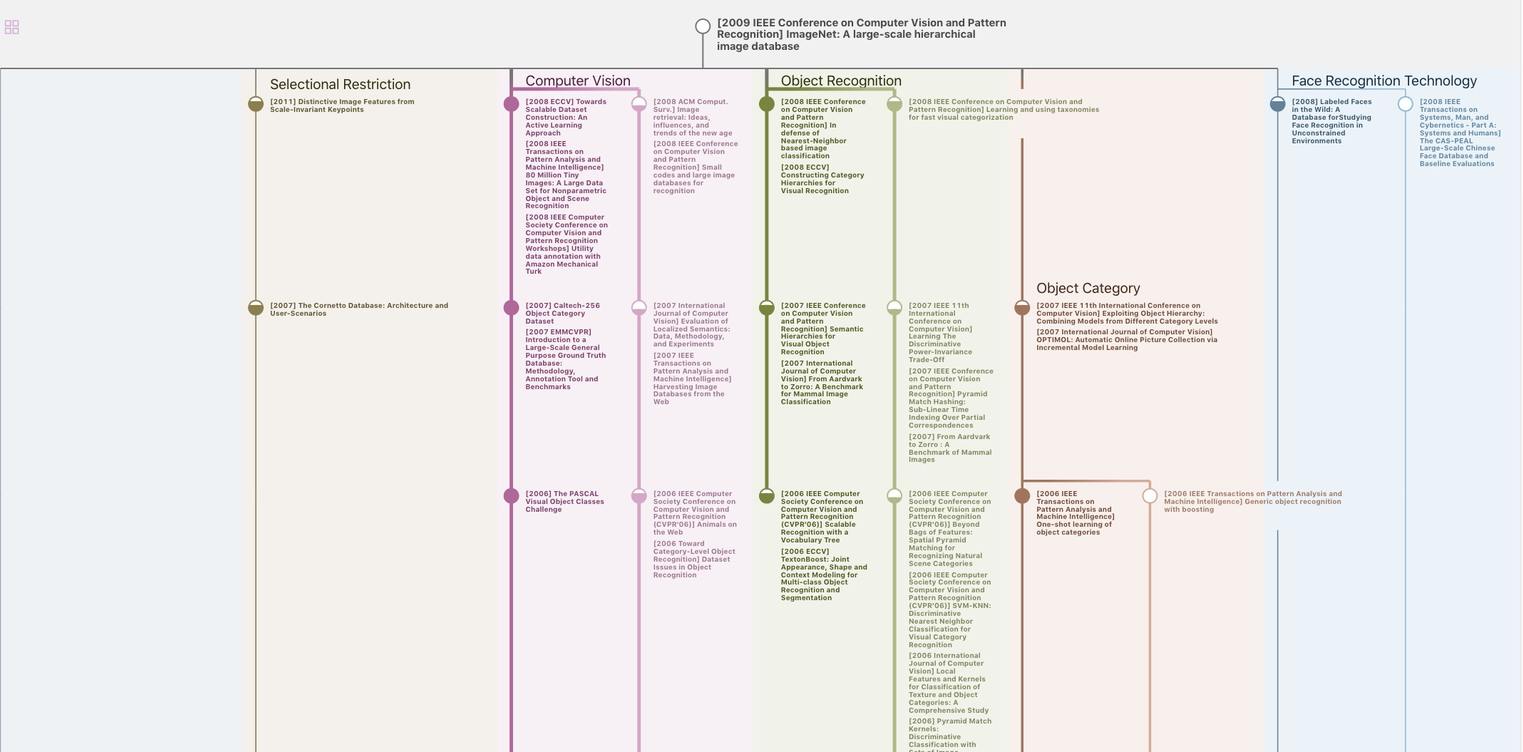
Generate MRT to find the research sequence of this paper
Chat Paper
Summary is being generated by the instructions you defined