Estimating Probability Distributions of Travel Times by Fitting a Markovian Velocity Model
IEEE Transactions on Intelligent Transportation Systems(2022)
摘要
To improve the routing decisions of individual drivers and the management policies designed by traffic operators, one needs reliable estimates of travel time distributions. Since congestion caused by both recurrent patterns (e.g., rush hours) and non-recurrent events (e.g., traffic incidents) leads to potentially substantial delays in highway travel times, we focus on a framework capable of incorporating both effects. To this end, we propose to work with the Markovian Velocity model (MVM), based on an environmental background process that tracks both random and (semi-)predictable events affecting the vehicle speeds in a highway network. We show how to operationalize this flexible data-driven model in order to obtain the travel time distribution for a vehicle departing at a known day and time to traverse a given path. Specifically, we detail how to structure the background process and set the speed levels corresponding to the different states of this process. First, for the inclusion of non-recurrent events, we study incident data to describe the random durations of the incident and inter-incident times. Both of them depend on the time of day, but we identify periods in which they can be considered time-independent. Second, for an estimation of the speed patterns in both incident and inter-incident regime, loop detector data for each of the identified periods is studied. In numerical examples that use road network detector data of the Dutch highway network, we obtain the travel time distribution estimates that arise under different traffic regimes, and illustrate the advantages compared to traditional travel-time prediction methods.
更多查看译文
关键词
Travel time distribution,Markovian background process,incident duration,recurrent congestion,loop detector data
AI 理解论文
溯源树
样例
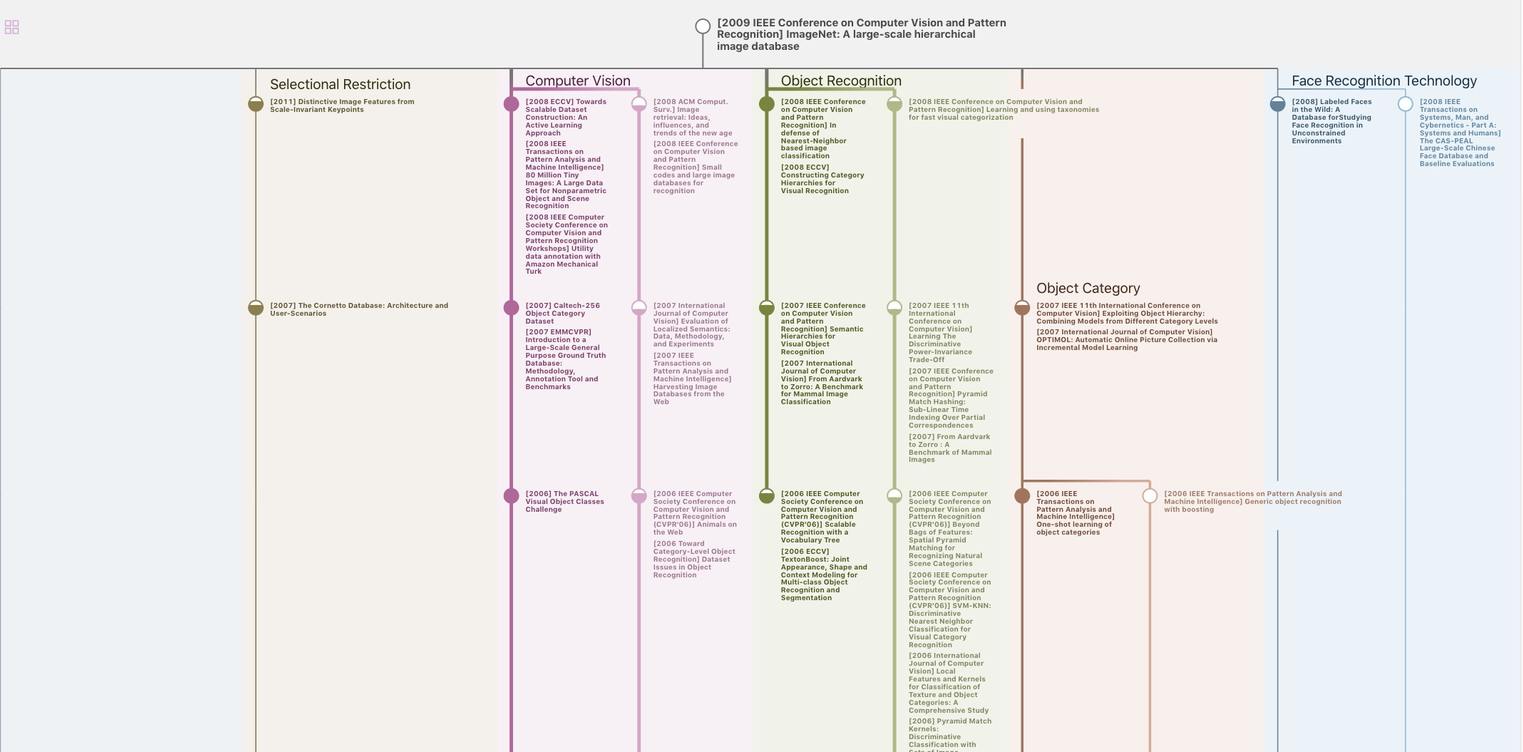
生成溯源树,研究论文发展脉络
Chat Paper
正在生成论文摘要