EAKF-based Parameter Optimization Using a Hybrid Adaptive Method
Monthly Weather Review(2022)
摘要
Abstract To effectively reduce model bias and improve assimilation quality, we adopt a hybrid 14 adaptive approach of ensemble adjustment Kalman filter (EAKF) and multigrid analysis 15 (MGA), called EAKF-MGA, to implement parameter optimization as follows. For each 16 assimilation cycle, observations are used to adjust the prior ensembles of both state variables 17 and parameters using the EAKF without inflation. Then, the MGA is adaptively triggered to 18 extract multiscale information from the observational residual to innovate the ensemble mean 19 of the state once again. Results of biased twin experiments consisting of a barotropic spectral 20 model and idealized observation systems show that the proposed EAKF-MGA is insensitive 21 to state variance inflation and localization during the parameter optimization process, 22 compared with the EAKF with adaptive inflation. We also find that computational efficiency 23 is another important advantage of the EAKF-MGA for both state estimation and parameter 24 estimation since extremely small ensemble size is allowed, while the EAKF with adaptive 25 inflation does not work anymore. In essence, the EAKF-MGA is designed to estimate and 26 correct systematic errors jointly with models state variables. Through alleviating biases, 27 including the model bias caused by the biased parameter and the analysis bias resulting from 28 the sampling noise given the limited ensemble size, it can be guaranteed that the analysis in 29 the EAKF-MGA will be proceeded onward with the standard assumption of the unbiased 30 model background field in modern data assimilation theory to be met.
更多查看译文
关键词
Data assimilation,Model errors,Optimization
AI 理解论文
溯源树
样例
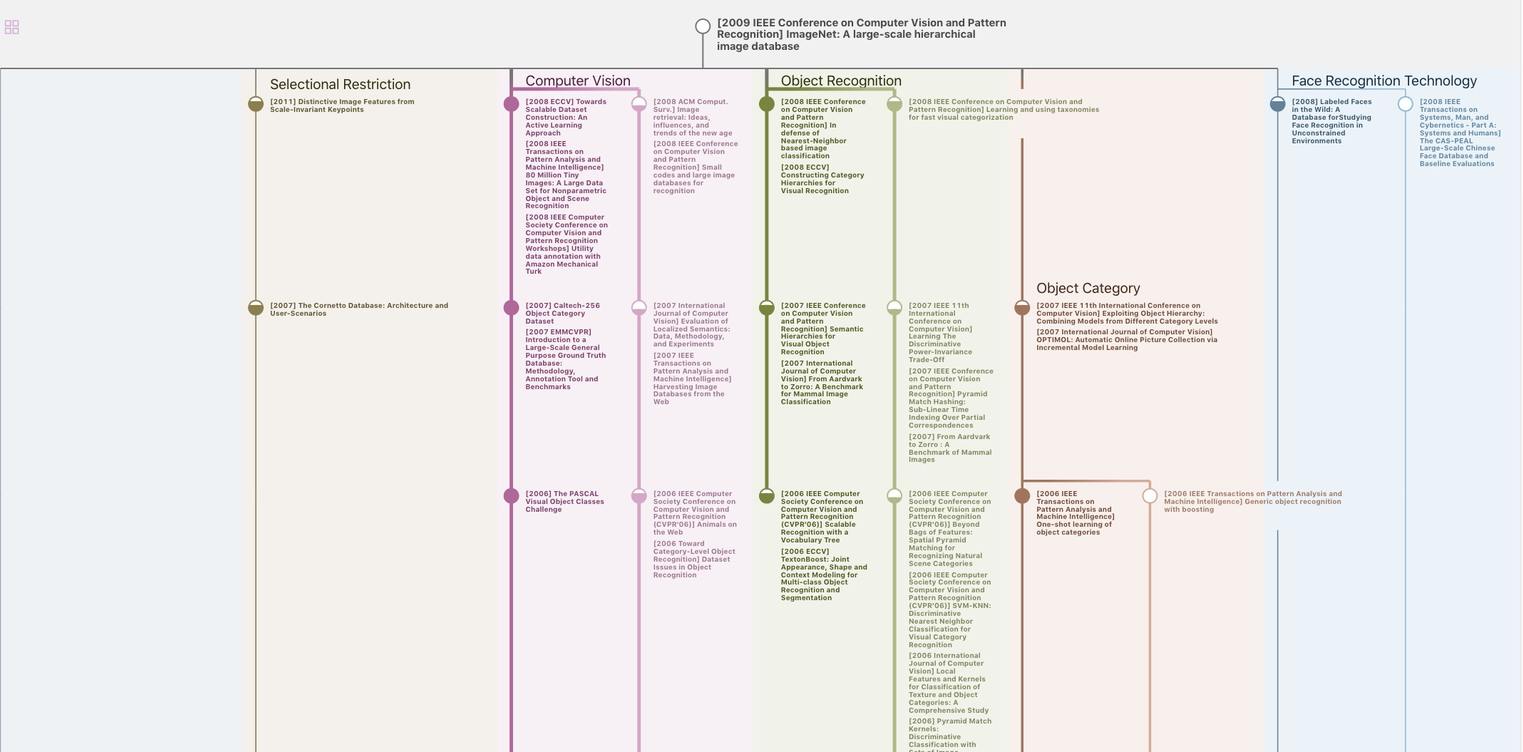
生成溯源树,研究论文发展脉络
Chat Paper
正在生成论文摘要