Automating Optical Network Fault Management with Machine Learning
IEEE Communications Magazine(2022)
摘要
Effective fault management is essential for quality of service assurance in optical networks. Conventional fault management designs for optical networks mainly rely on threshold-based rules, which can hardly characterize the complex fault patterns therein. This article discusses the application of machine learning (ML) in actuating an automated optical network fault management architecture. The architecture is built on advanced optical performance monitoring (OPM) techniques and software-defined-networking-enabled programmable network control and management. With the viability of abundant OPM data, the architecture employs various ML models to automatically learn fault patterns and thereby realize data-driven cognitive fault management. We review the state of the art on fault detection, identification, and localization based on such an architecture, focusing specifically on soft failures. To overcome the applicability and scalability issues encountered by existing soft failure detection designs, we present a hybrid learning solution that combines the merits of supervised, unsupervised, and cooperative ML. In particular, we present a self-taught mechanism that self-learns fault patterns with unsupervised learning and then trains supervised learning classifiers with the learned patterns for online detection. We further introduce a broker-plane-aided federated learning framework to enable collaborative training of classifiers from multiple network domains while complying with domain privacy constraints. Performance evaluations show that the hybrid learning design can achieve high fault detection rates with negligible false alarms using less than 10 abnormal data samples (~ 0.1 percent of the scale of normal samples) for training.
更多查看译文
AI 理解论文
溯源树
样例
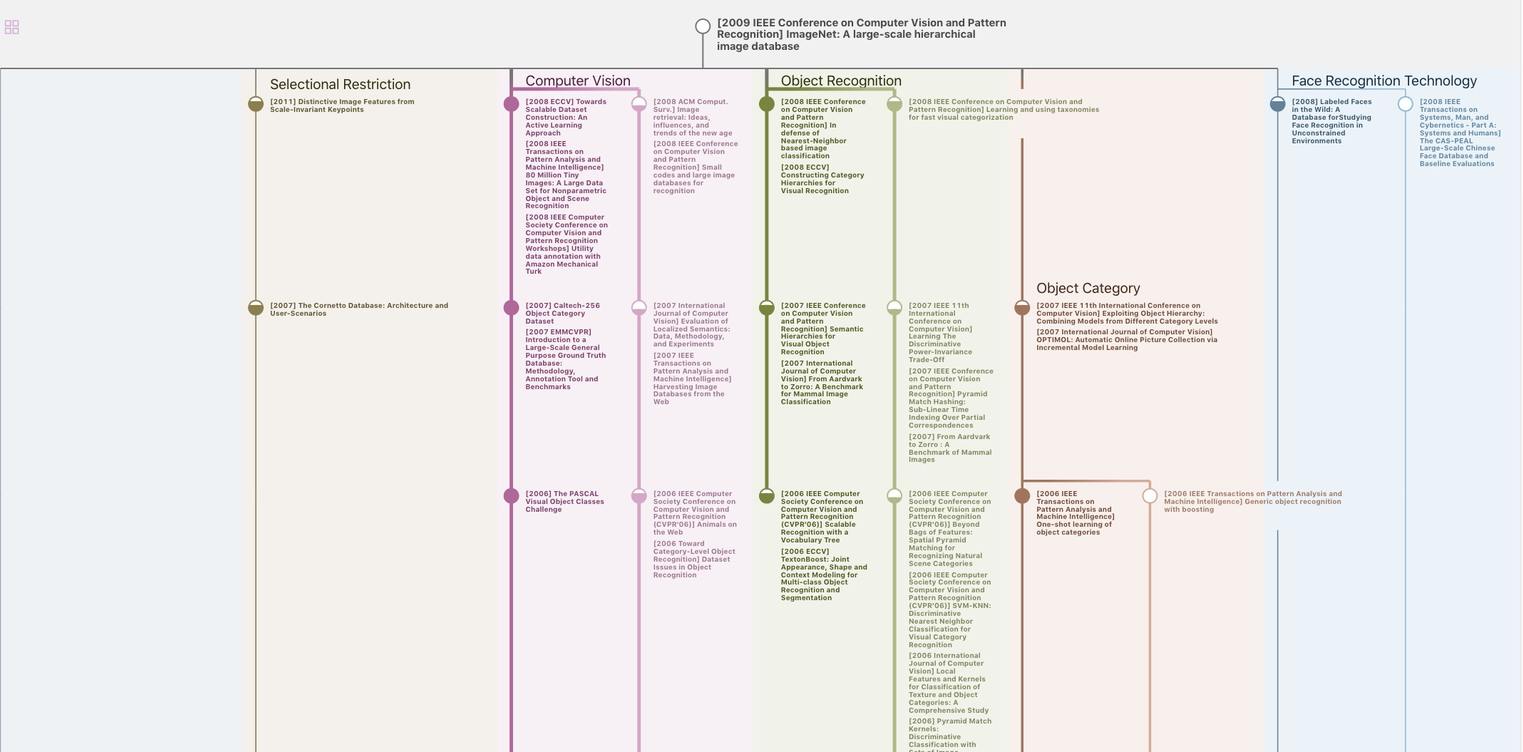
生成溯源树,研究论文发展脉络
Chat Paper
正在生成论文摘要