Machine learning-based identification for the main influencing factors of alluvial fan development in the Lhasa River Basin, Qinghai-Tibet Plateau
Journal of Geographical Sciences(2022)
摘要
Alluvial fans are an important land resource in the Qinghai-Tibet Plateau with the expansion of human activities. However, the factors of alluvial fan development are poorly understood. According to our previous investigation and research, approximately 826 alluvial fans exist in the Lhasa River Basin (LRB). The main purpose of this work is to identify the main influencing factors by using machine learning. A development index (Di) of alluvial fan was created by combining its area, perimeter, height and gradient. The 72% of data, including Di, 11 types of environmental parameters of the matching catchment of alluvial fan and 10 commonly used machine learning algorithms were used to train and build models. The 18% of data were used to validate models. The remaining 10% of data were used to test the model accuracy. The feature importance of the model was used to illustrate the significance of the 11 types of environmental parameters to Di. The primary modelling results showed that the accuracy of the ensemble models, including Gradient Boost Decision Tree, Random Forest and XGBoost, are not less than 0.5 ( R 2 ). The accuracy of the Gradient Boost Decision Tree and XGBoost improved after grid research, and their R 2 values are 0.782 and 0.870, respectively. The XGBoost was selected as the final model due to its optimal accuracy and generalisation ability at the sites closest to the LRB. Morphology parameters are the main factors in alluvial fan development, with a cumulative value of relative feature importance of 74.60% in XGBoost. The final model will have better accuracy and generalisation ability after adding training samples in other regions.
更多查看译文
关键词
alluvial fan,machine learning,feature importance,XGBoost,Lhasa River Basin
AI 理解论文
溯源树
样例
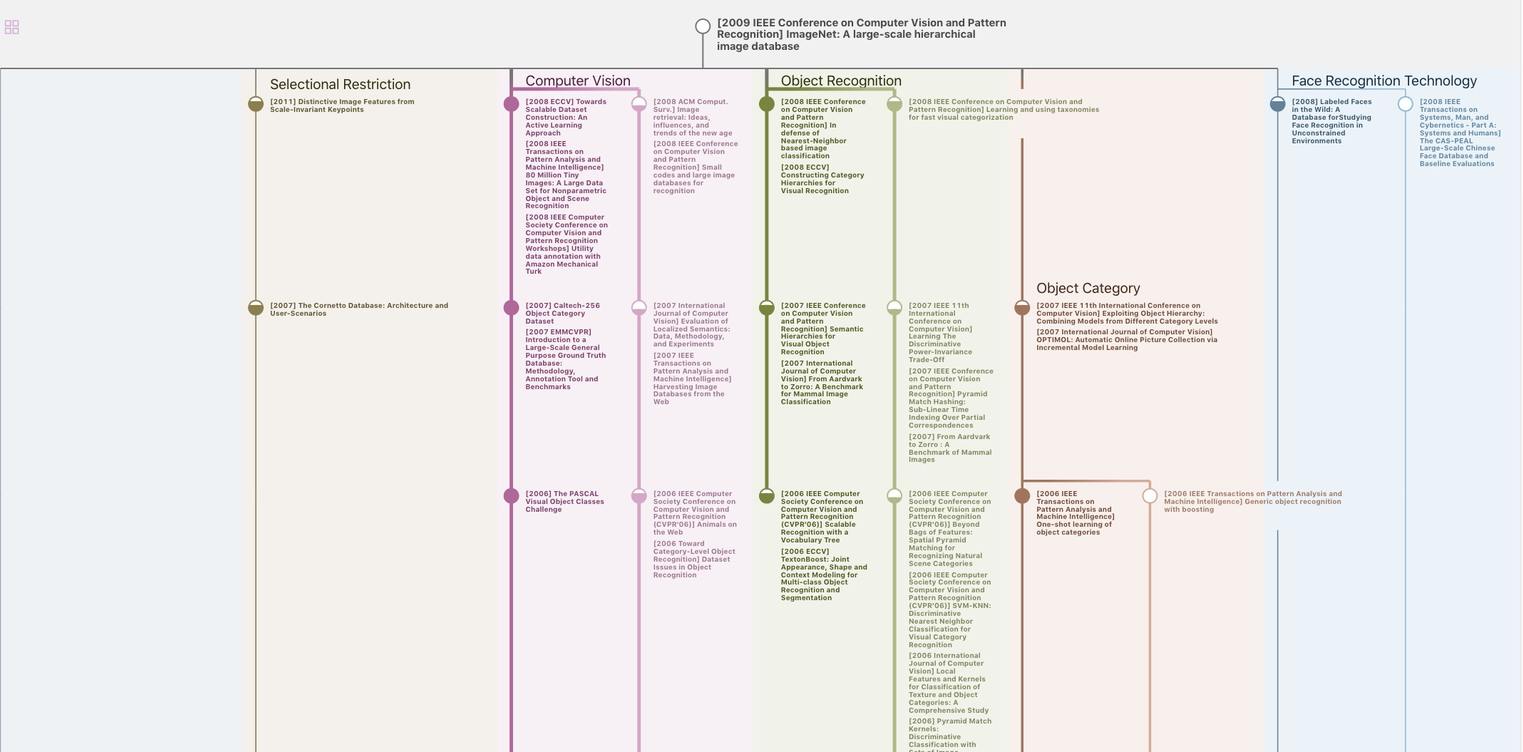
生成溯源树,研究论文发展脉络
Chat Paper
正在生成论文摘要