Ab-initio quantum chemistry with neural-network wavefunctions
arxiv(2022)
摘要
Machine learning and specifically deep-learning methods have outperformed human capabilities in many pattern recognition and data processing problems, in game playing, and now also play an increasingly important role in scientific discovery. A key application of machine learning in the molecular sciences is to learn potential energy surfaces or force fields from ab-initio solutions of the electronic Schr\"odinger equation using datasets obtained with density functional theory, coupled cluster, or other quantum chemistry methods. Here we review a recent and complementary approach: using machine learning to aid the direct solution of quantum chemistry problems from first principles. Specifically, we focus on quantum Monte Carlo (QMC) methods that use neural network ansatz functions in order to solve the electronic Schr\"odinger equation, both in first and second quantization, computing ground and excited states, and generalizing over multiple nuclear configurations. Compared to existing quantum chemistry methods, these new deep QMC methods have the potential to generate highly accurate solutions of the Schr\"odinger equation at relatively modest computational cost.
更多查看译文
关键词
quantum,ab-initio,neural-network
AI 理解论文
溯源树
样例
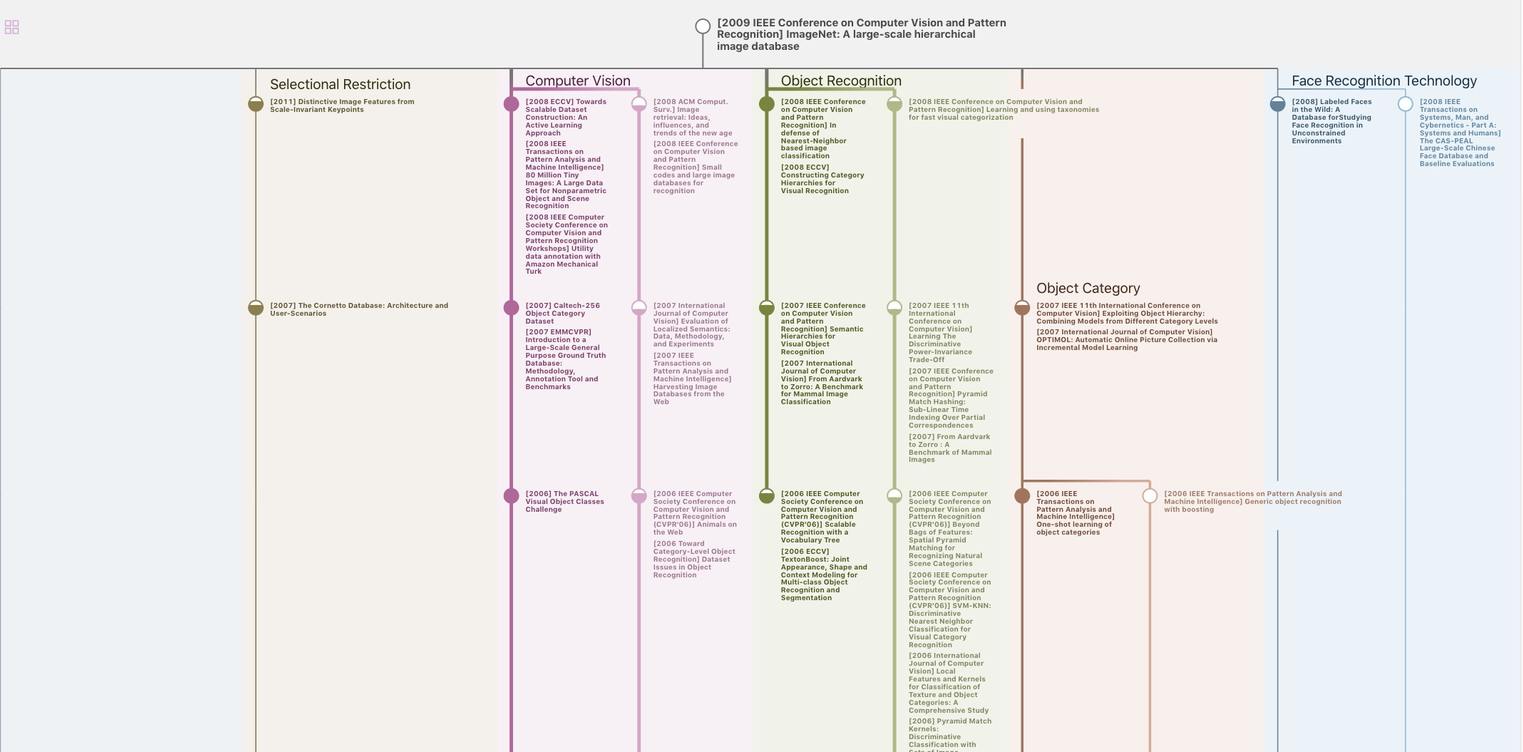
生成溯源树,研究论文发展脉络
Chat Paper
正在生成论文摘要