BITS: Bi-level Imitation for Traffic Simulation
2023 IEEE INTERNATIONAL CONFERENCE ON ROBOTICS AND AUTOMATION, ICRA(2023)
摘要
Simulation is the key to scaling up validation and verification for robotic systems such as autonomous vehicles. Despite advances in high-fidelity physics and sensor simulation, a critical gap remains in simulating realistic behaviors of road users. This is because devising first principle models for human-like behaviors is generally infeasible. In this work, we take a data-driven approach to generate traffic behaviors from real-world driving logs. The method achieves high sample efficiency and behavior diversity by exploiting the bi-level hierarchy of high-level intent inference and low-level driving behavior imitation. The method also incorporates a planning module to obtain stable long-horizon behaviors. We empirically validate our method with scenarios from two large-scale driving datasets and show our method achieves balanced traffic simulation performance in realism, diversity, and long-horizon stability. We also explore ways to evaluate behavior realism and introduce a suite of evaluation metrics for traffic simulation. Finally, as part of our core contributions, we develop and open source a software tool that unifies data formats across different driving datasets and converts scenes from existing datasets into interactive simulation environments. For video results and code release, see https://bit.ly/3L9uzj3.
更多查看译文
AI 理解论文
溯源树
样例
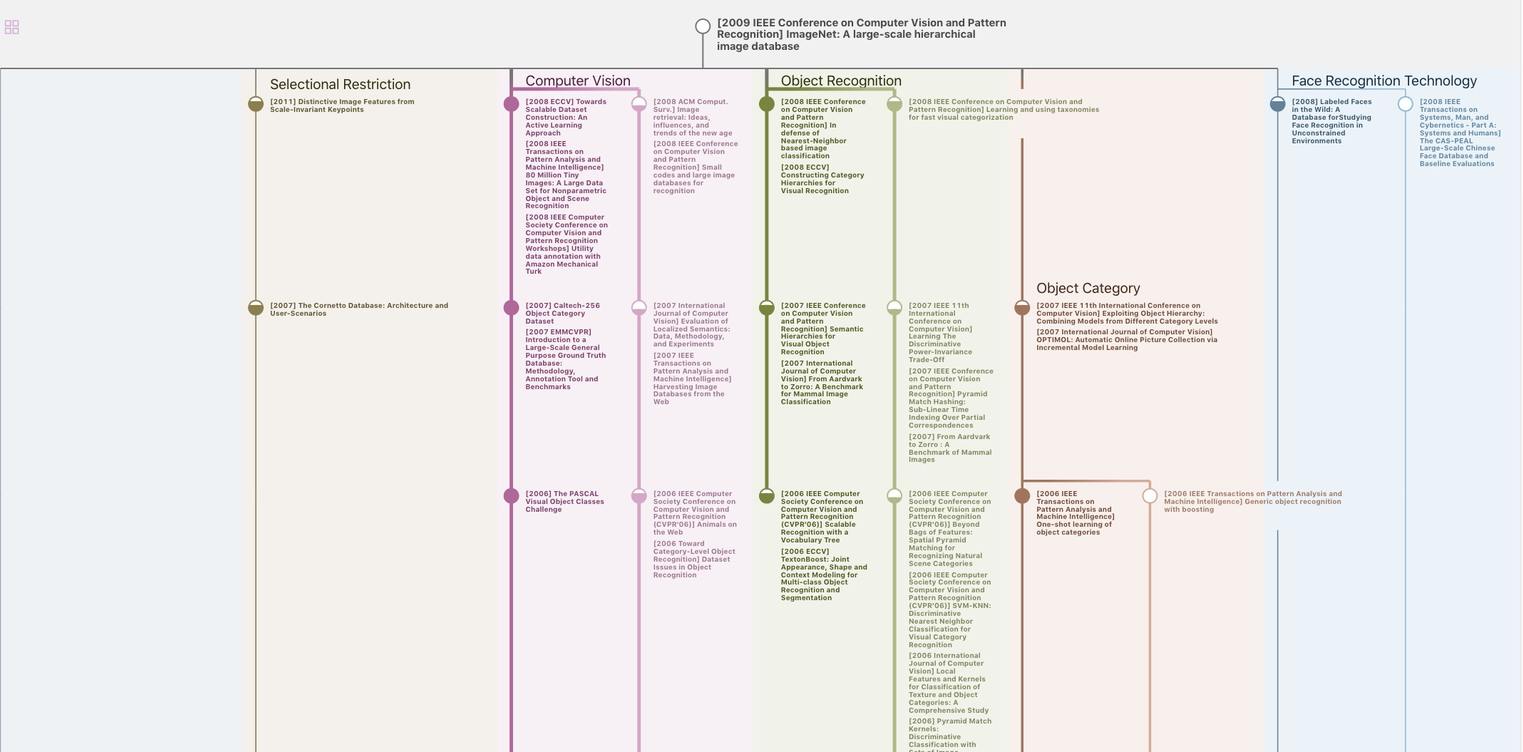
生成溯源树,研究论文发展脉络
Chat Paper
正在生成论文摘要