Mutual gain adaptive network for segmenting brain stroke lesions
Applied Soft Computing(2022)
摘要
Brain stroke, an acute vascular disease, can lead to brain damage. As the incidence rate continues to increase, it becomes urgent and yet significantly to develop automatic tools for segmenting brain stroke lesions, for helping radiologists to detect brain stroke both effectively and accurately. Deep learning models, though have advanced this task, they are still suffering from the huge size variation and ambiguous boundaries of stroke lesions, both substantially degrading the segmentation performance. In this paper, we proposed an effective and generic learning network, called mutual gain adaptive network, to enhance the network’s ability on dealing with size variation and distinguishing ambiguous boundaries, for advancing deep neural networks in brain stroke lesion segmentation. The main contribution is that 1) a mutual gain adaptive similarity (MGAS) block and 2) a global context-awareness (GCA) block. MGAS block aims at capturing both short- and long-range spatial dependencies, for better encoding global contexts by leveraging feature similarity, to enhance feature activation for segmenting stroke lesions with different sizes. GCA block is designed to supply global context for low-semantic features, for facilitating the restoration of pixel localization to distinguish ambiguous boundaries. Our network is assessed in a publicly available dataset, e.g. Anatomical Tracings of Lesions After Stroke (ATLAS). Extensive experimental results show that it is better than state-of-the-art methods.
更多查看译文
关键词
Brain stroke,Lesion segmentation,Deep learning,Ambiguous boundary distinguishing
AI 理解论文
溯源树
样例
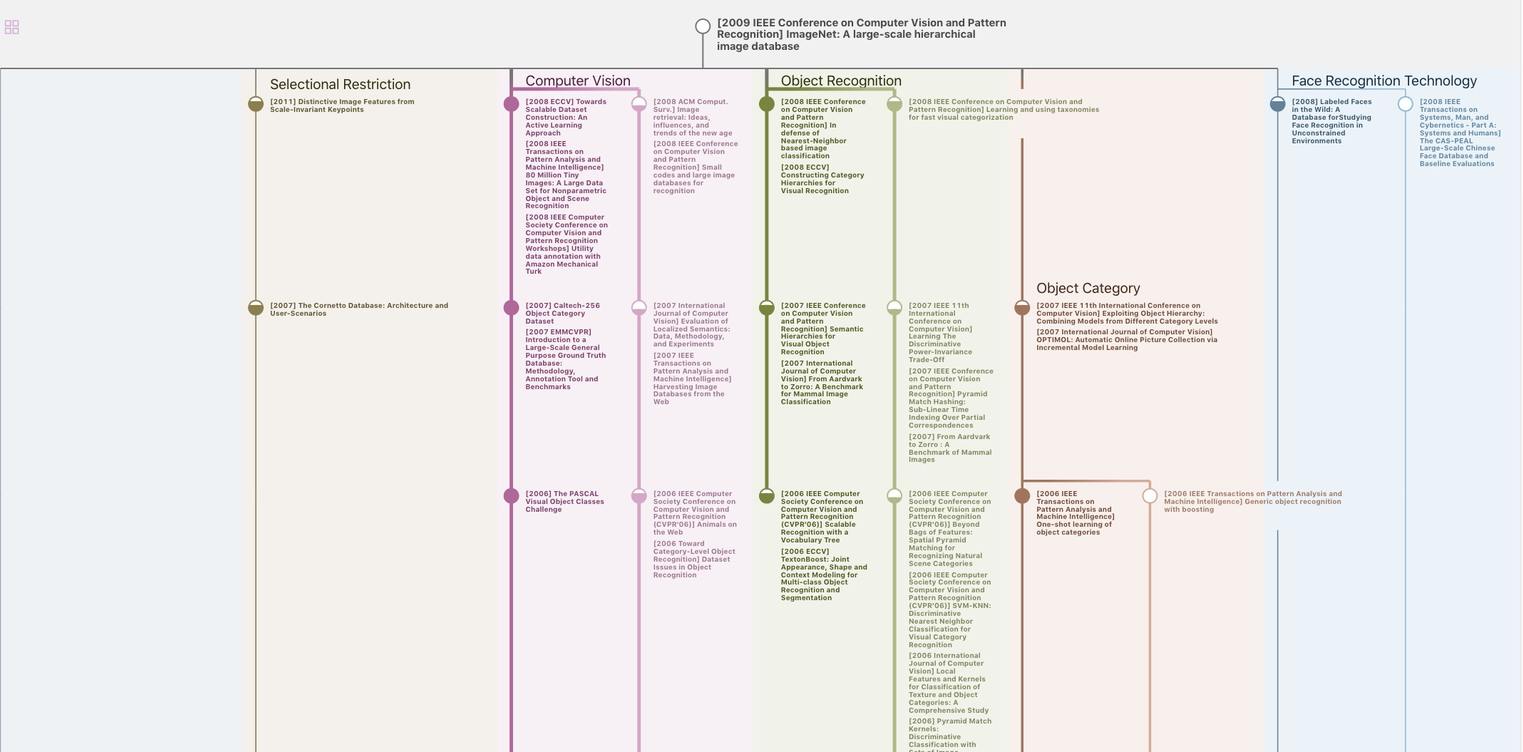
生成溯源树,研究论文发展脉络
Chat Paper
正在生成论文摘要