Automated measurement of spine indices on axial MR images for lumbar spinal stenosis diagnosis using segmentation-guided regression network
Medical Physics(2023)
摘要
Purpose Automated measurement of spine indices on axial magnetic resonance (MR) images plays a significant role in lumbar spinal stenosis diagnosis. Existing direct spine indices measurement approaches fail to explicitly focus on the task-specific region or feature channel with the additional information for guiding. We aim to achieve accurate spine indices measurement by introducing the guidance of the segmentation task. Methods In this paper, we propose a segmentation-guided regression network (SGRNet) to achieve automated spine indices measurement. SGRNet consists of a segmentation path for generating the spine segmentation prediction and a regression path for producing spine indices estimation. The segmentation path is a U-Net-like network which includes a segmentation encoder and a decoder which generates multilevel segmentation features and segmentation prediction. The proposed segmentation-guided attention module (SGAM) in the regression encoder extracts the attention-aware regression feature under the guidance of the segmentation feature. Based on the attention-aware regression feature, a fully connected layer is utilized to output the accurate spine indices estimation. Results Experiments on the open-access Lumbar Spine MRI data set show that SGRNet achieves state-of-the-art performance with a mean absolute error of 0.49 mm and mean Pearson correlation coefficient of 0.956 for four indices estimation. Conclusions The proposed SGAM in SGRNet is capable of improving the performance of spine indices measurement by focusing on the task-specific region and feature channel under the guidance of the segmentation task.
更多查看译文
关键词
attention mechanism,lumbar spinal stenosis diagnosis,multitask learning,spine
AI 理解论文
溯源树
样例
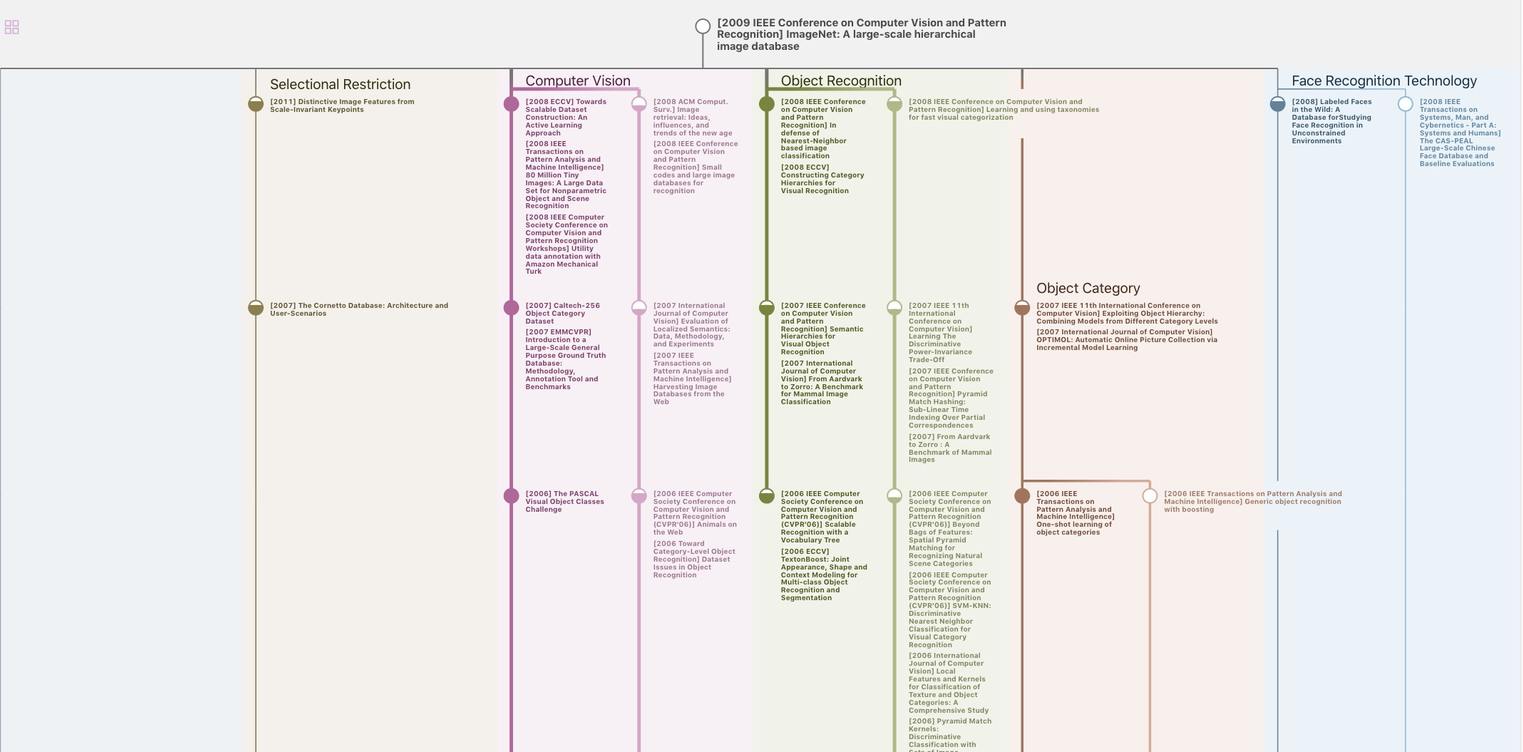
生成溯源树,研究论文发展脉络
Chat Paper
正在生成论文摘要