Reliable Self-Supervised Information Mining for Deep Subspace Clustering
2022 IEEE International Conference on Multimedia and Expo (ICME)(2022)
摘要
Deep subspace clustering has achieved remarkable performance in unsupervised clustering tasks. The self-supervised approach is further introduced to learn more discriminative representation for enhancing clustering performance. Despite the significant improvement of clustering performance by exploiting self-supervision information, these approaches heavily depend on the high quality of pseudo-label from the current clustering result and this will inevitably degrade the clustering performance when the obtained pseudo-labels are incorrect. To solve this issue, we develop a robust self-supervised deep subspace clustering approach by exploiting the reliable self-supervised information during training. The proposed method is involved in two key steps: a diffusion processing step is developed to improve self-expressiveness matrix such that more accurate clustering result (pseudo-labels) can be obtained. More importantly, we further propose to estimate and exploit the reliability of the assigned pseudo-label for each sample to alleviate the negative impact of incorrect pseudo-labels, such that the unreliable self-supervision can be further alleviated. Experimental studies on several benchmark datasets validate the effectiveness of our approach in terms of refining the self-supervised information. The source code of the proposed method is available at the https://github.com/stuljj/RSDSC.git.
更多查看译文
关键词
Deep subspace clustering,Self-supervised training
AI 理解论文
溯源树
样例
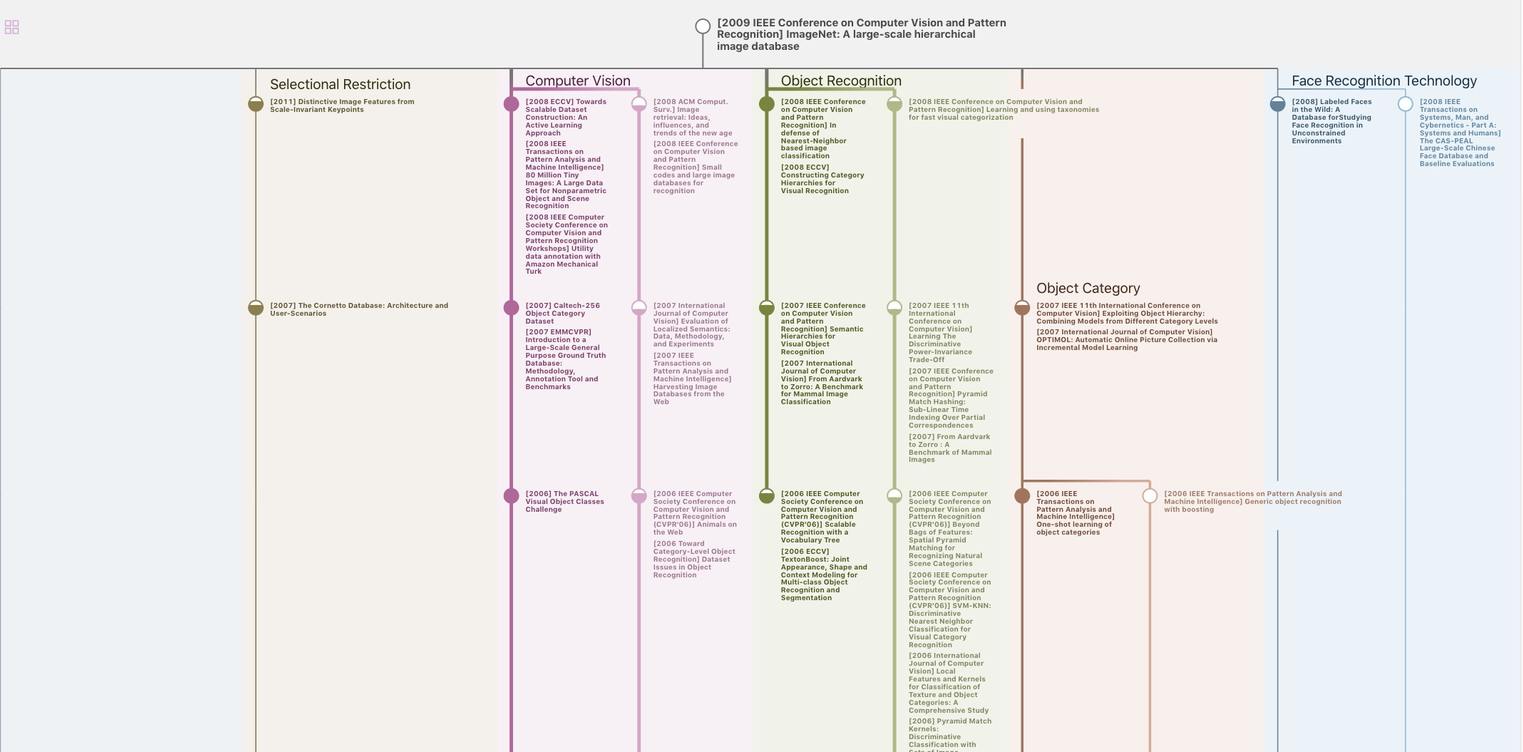
生成溯源树,研究论文发展脉络
Chat Paper
正在生成论文摘要