Feature Extraction of Sequence Data Based on LSTM and its Application to Fault Diagnosis of Industrial Process
2022 IEEE 11th Data Driven Control and Learning Systems Conference (DDCLS)(2022)
摘要
Data with sequential relationships such as text, speech, and sensor data, belong to sequence data. Many deep learning models are proposed to extract features and learn inherent information among the sequence data. We investigate the similarities and differences of the modeling methods of sequence data in various fields, and then use deep learning neural network to build a model for the fault diagnosis of sequence data in process industry. Long-short-term memory (LSTM) network and feature extraction of contrastive predictive coding (CPC) are used in this paper for building deep learning models. CPC can learn representations of data, and LSTM can learn the information contained in sequence data. For application to fault diagnosis, the whole model combining CPC and LSTM can appropriately distinguish normal data from various fault data. Experiments on the Tennessee-Eastman process show that the LSTM model combining with proper feature extraction methods can improve the performance of fault diagnosis.
更多查看译文
关键词
Feature Extraction,LSTM,Contrastive Predictive Coding,Fault Diagnosis
AI 理解论文
溯源树
样例
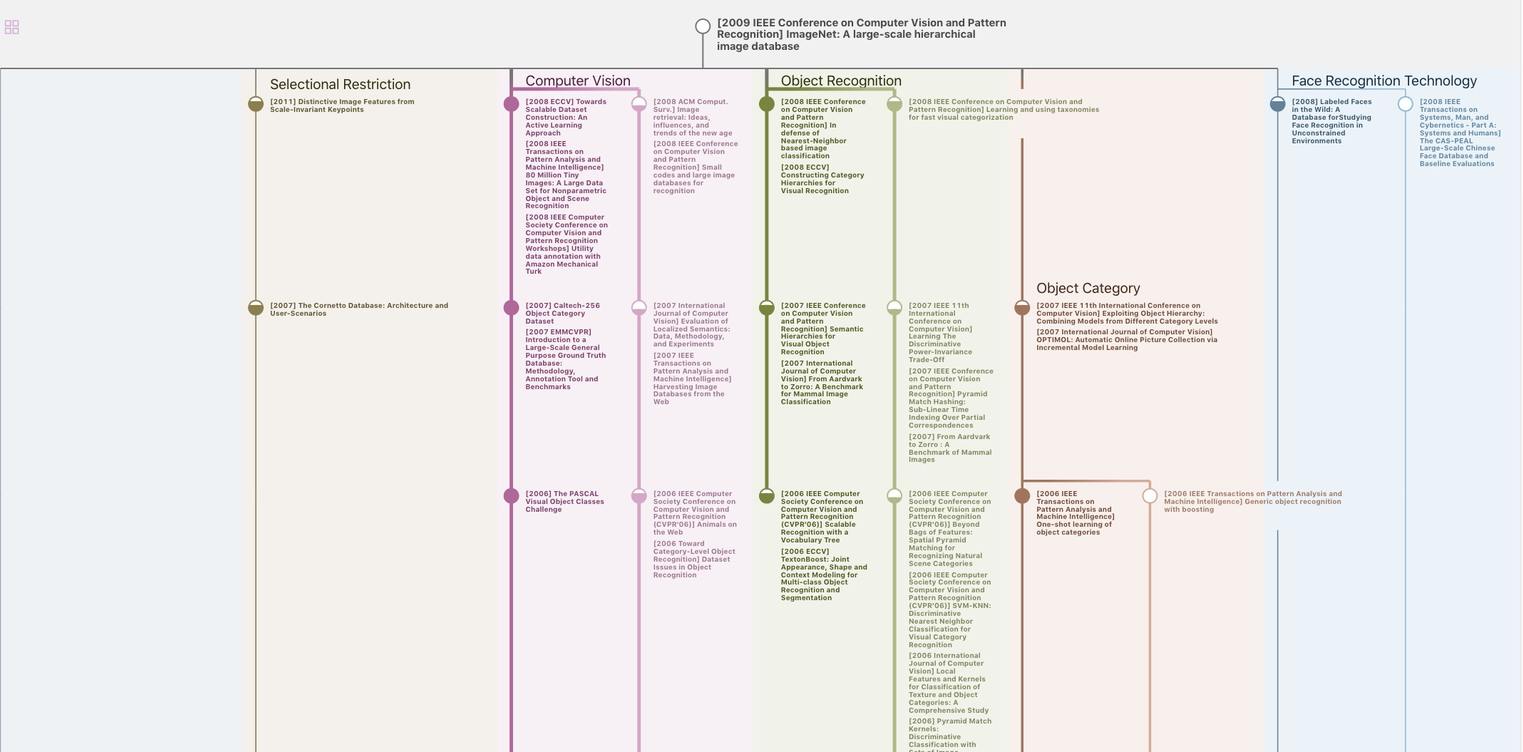
生成溯源树,研究论文发展脉络
Chat Paper
正在生成论文摘要