Deep learning approaches for anomalies detection of bladder ct contours in prostate cancer patients
Journal of Mechanics in Medicine and Biology(2022)
摘要
In radiation therapy, poor contour delineations of Region of Interests (ROI) may result in incorrect radiation dosing and increase the chance of radiation-induced toxicities. To better recognize these contours, we used a feature fusion-based 3-dimensional convolution neural network (3D-CNN), multi-view convolution neural network (MV-CNN), and 3D point cloud to retrospectively detect abnormal bladder contours among prostate cancer patients. We defined three patterns of abnormal bladder contours: (1) discontinuities: missing slices and disparate volumes, (2) surface aberrations: extended or contracted slices, (3) small volume: bladder volume < 150 ml. We created virtual samples for discontinuities and surface aberrations by randomly adding pixel to one or more slices outside the original contours and randomly extending or shrinking one or more slices. For both 3D-CNN and MV-CNN methods, we utilized the Local Binary Pattern (LBP) and Canny operators for feature extraction and compared several feature fusion-based approaches, including Original image + LBP processed image, Original image + Canny processed image, Original image + LBP processed image + Canny processed image. For the 3D point cloud, we applied the 3D point cloud segmentation method (Rand LA-Net) and the 3D point cloud classifying method (DGCNN). We had 344 samples available (219 real contours and 125 virtual contours) altogether. All the algorithms were trained on 200 bladder contours, validated on 100 bladders, then tested on 44 real annotated contours. Among all the approaches, the "Original + LBP"-based MV-CNN model achieved the highest accuracy: 100% for predicting discontinuity and small volume, 91.3% for predicting normal contours, and 90.9% for predicting surface aberrations. MV-CNN performed more accurately and efficiently across all the feature fusion methods. In particular, "Original + LBP"-based MV-CNN achieved the highest test accuracy. Our tools can detect anomalies of bladder contours in clinical settings and may improve the process of contour quality assurance (QA).
更多查看译文
关键词
Anomalies detection Bladder contours,ROI delineation,deep learning,feature fusion
AI 理解论文
溯源树
样例
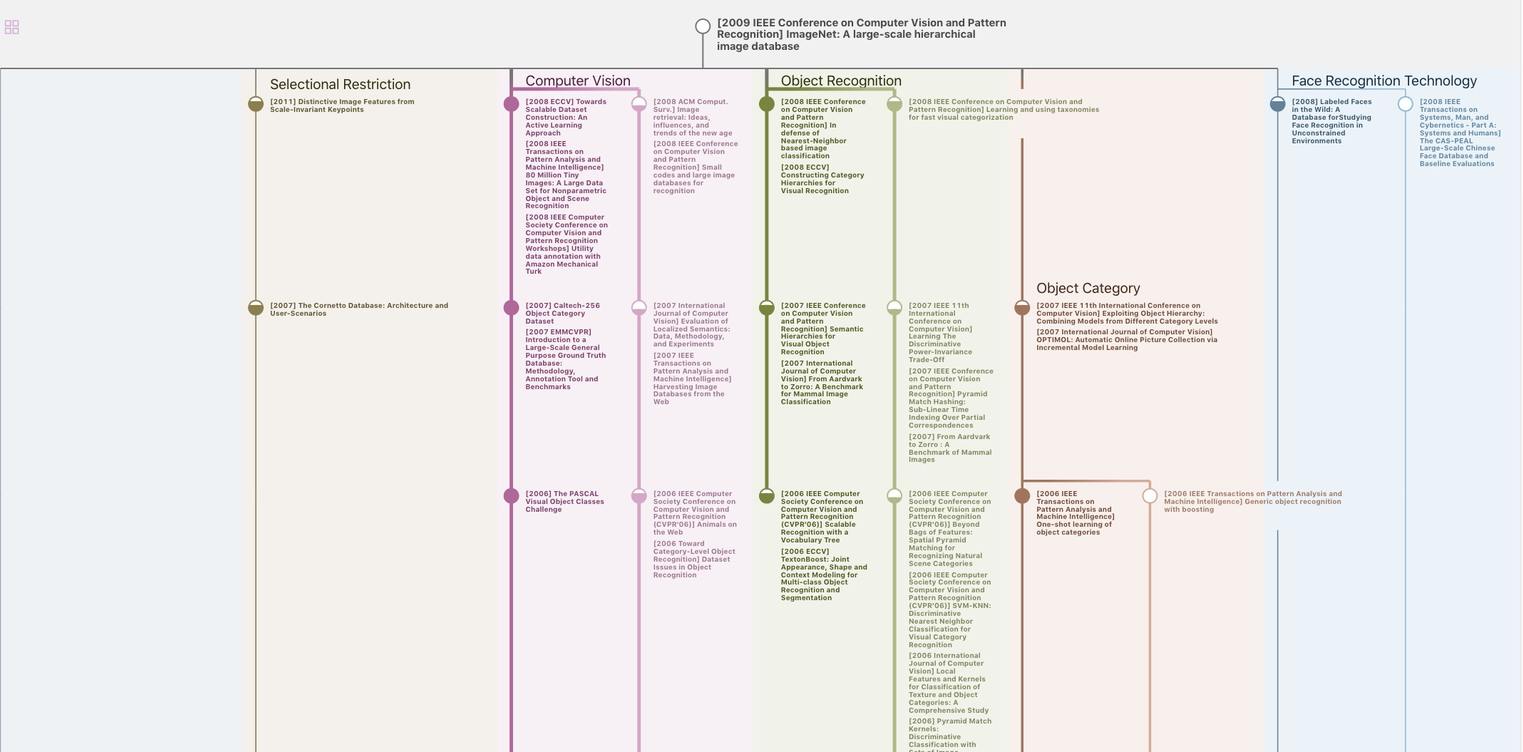
生成溯源树,研究论文发展脉络
Chat Paper
正在生成论文摘要