Extending machine learning beyond interatomic potentials for predicting molecular properties
Nature Reviews Chemistry(2022)
摘要
Machine learning (ML) is becoming a method of choice for modelling complex chemical processes and materials. ML provides a surrogate model trained on a reference dataset that can be used to establish a relationship between a molecular structure and its chemical properties. This Review highlights developments in the use of ML to evaluate chemical properties such as partial atomic charges, dipole moments, spin and electron densities, and chemical bonding, as well as to obtain a reduced quantum-mechanical description. We overview several modern neural network architectures, their predictive capabilities, generality and transferability, and illustrate their applicability to various chemical properties. We emphasize that learned molecular representations resemble quantum-mechanical analogues, demonstrating the ability of the models to capture the underlying physics. We also discuss how ML models can describe non-local quantum effects. Finally, we conclude by compiling a list of available ML toolboxes, summarizing the unresolved challenges and presenting an outlook for future development. The observed trends demonstrate that this field is evolving towards physics-based models augmented by ML, which is accompanied by the development of new methods and the rapid growth of user-friendly ML frameworks for chemistry.
更多查看译文
关键词
Chemistry/Food Science,general,Analytical Chemistry,Organic Chemistry,Physical Chemistry,Inorganic Chemistry,Biochemistry
AI 理解论文
溯源树
样例
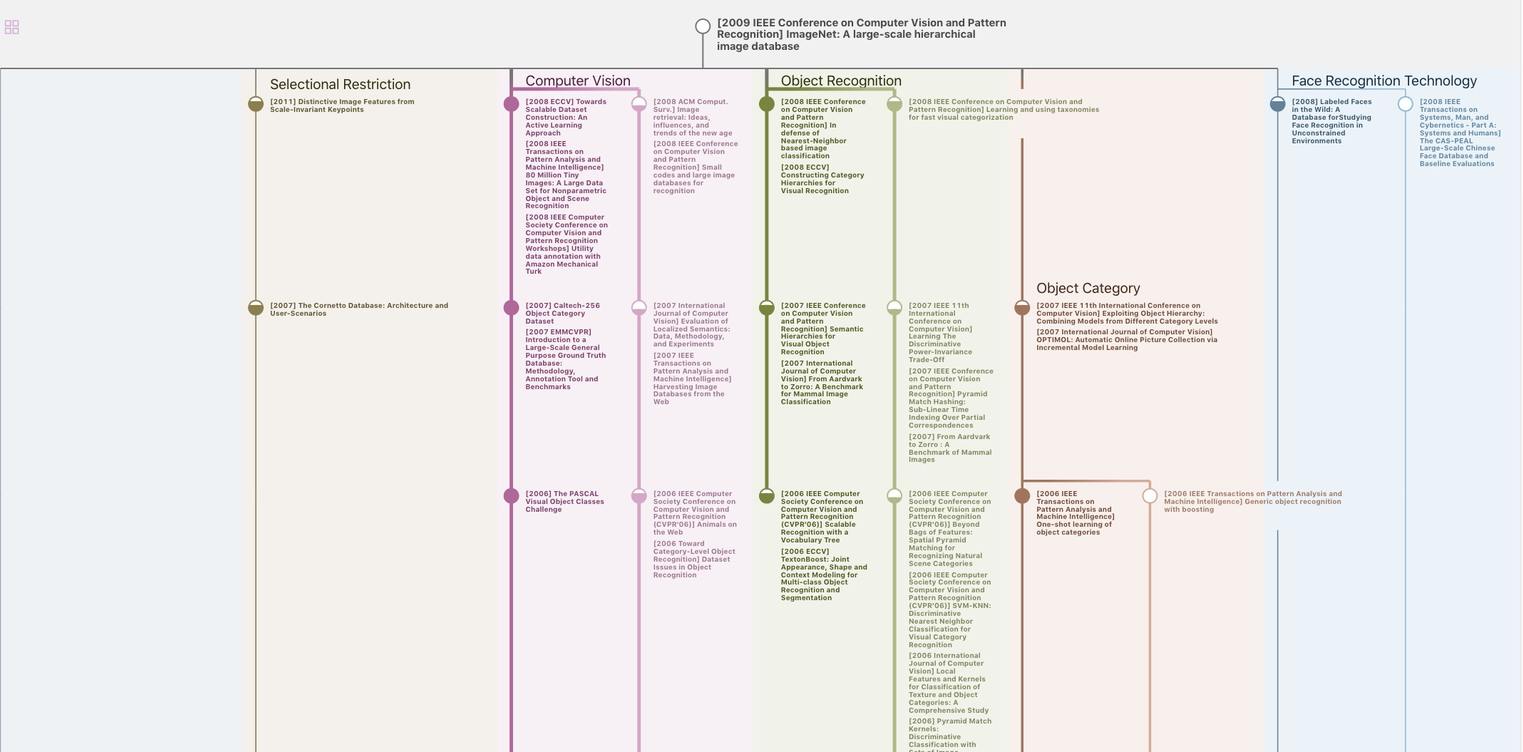
生成溯源树,研究论文发展脉络
Chat Paper
正在生成论文摘要