MAB-based Joint Optimization of Wireless LAN and Machine Learning for Communication-efficient Distributed Inference in Lossy Networks
2022 IEEE 95th Vehicular Technology Conference: (VTC2022-Spring)(2022)
摘要
Distributed inference is an emerging technology that enables inference with cutting-edge machine learning (ML) models such as deep neural networks (DNNs) on resource-constrained devices. However, narrow-band and lossy wireless networks easily create bottlenecks and increase the latency in distributed inference. This study proposes the joint optimization of an ML model and wireless communication parameters (such as transmission rate and retransmission limit) to reduce communication latency while maintaining the accuracy of inference. Our key idea is to utilize the packet-loss tolerance of ML inference that decreased the reliability but reduced communication latency. To this end, the proposed method based on the multi-armed bandit (MAB) algorithm, namely the upper confidence bound (UCB) algorithm, jointly optimizes (i) the wireless communication parameters that control the trade-off between reliability and latency in communications and (ii) the architecture of the ML model that controls the trade-off between accuracy and packet-loss reliance in ML inference. The results of computer simulations using ns3-ai show that the proposed method of joint optimization maintains the accuracy of inference as well as achieves a lower latency than when only the architecture of the ML model or communication parameters are optimized.
更多查看译文
关键词
Distributed inference,Joint control,Machine learning,Wireless LAN,Reinforcement Learning
AI 理解论文
溯源树
样例
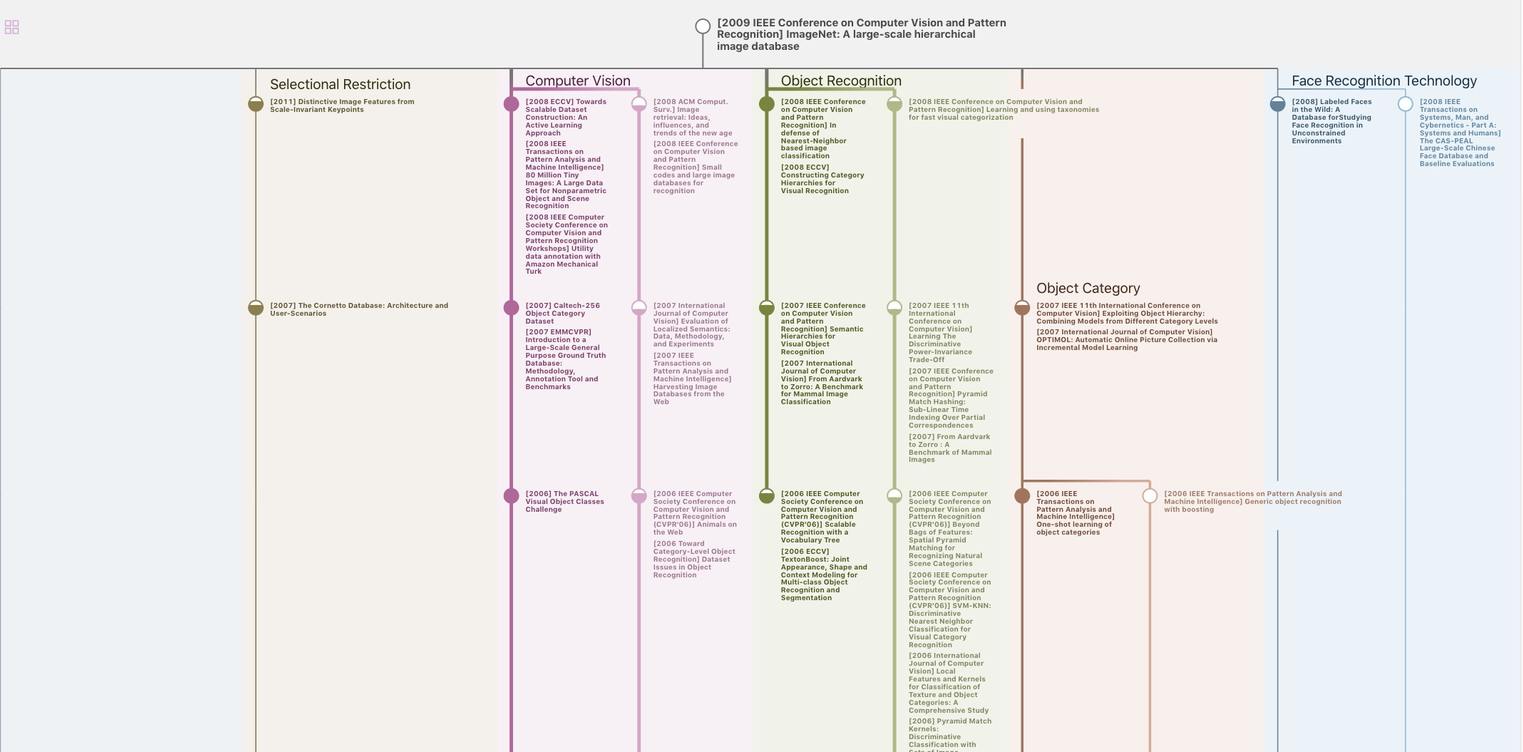
生成溯源树,研究论文发展脉络
Chat Paper
正在生成论文摘要