Removing InSAR Topography-Dependent Atmospheric Effect Based on Deep Learning
REMOTE SENSING(2022)
Abstract
Atmospheric effects are among the primary error sources affecting the accuracy of interferometric synthetic aperture radar (InSAR). The topography-dependent atmospheric effect is particularly noteworthy in reservoir areas for landslide monitoring utilizing InSAR, which must be effectively corrected to complete the InSAR high-accuracy measurement. This paper proposed a topography-dependent atmospheric correction method based on the Multi-Layer Perceptron (MLP) neural network model combined with topography and spatial data information. We used this proposed approach for the atmospheric correction of the interferometric pairs of Sentinel-1 images in the Baihetan dam. We contrasted the outcomes with those obtained using the generic atmospheric correction online service for InSAR (GACOS) correction and the traditional linear model correction. The results indicated that the MLP neural network model correction reduced the phase standard deviation of the Sentinel-1 interferogram by an average of 64% and nearly eliminated the phase-elevation correlation. Both comparisons outperformed the GACOS correction and the linear model correction. Through two real-world examples, we demonstrated how slopes with displacements, which were previously obscured by a significant topography-dependent atmospheric delay, could be successfully and clearly identified in the interferograms following the correction by the MLP neural network. The topography-dependent atmosphere can be better corrected using the MLP neural network model suggested in this paper. Unlike the previous model, this proposed approach could be adjusted to fit each interferogram, regardless of how much of the topography-dependent atmosphere was present. In order to improve the effectiveness of DInSAR and time-series InSAR solutions, it can be applied immediately to the interferogram to retrieve the effective displacement information that cannot be identified before the correction.
MoreTranslated text
Key words
InSAR,topography-dependent atmospheric correction,linear model,GACOS,MLP neural network
AI Read Science
Must-Reading Tree
Example
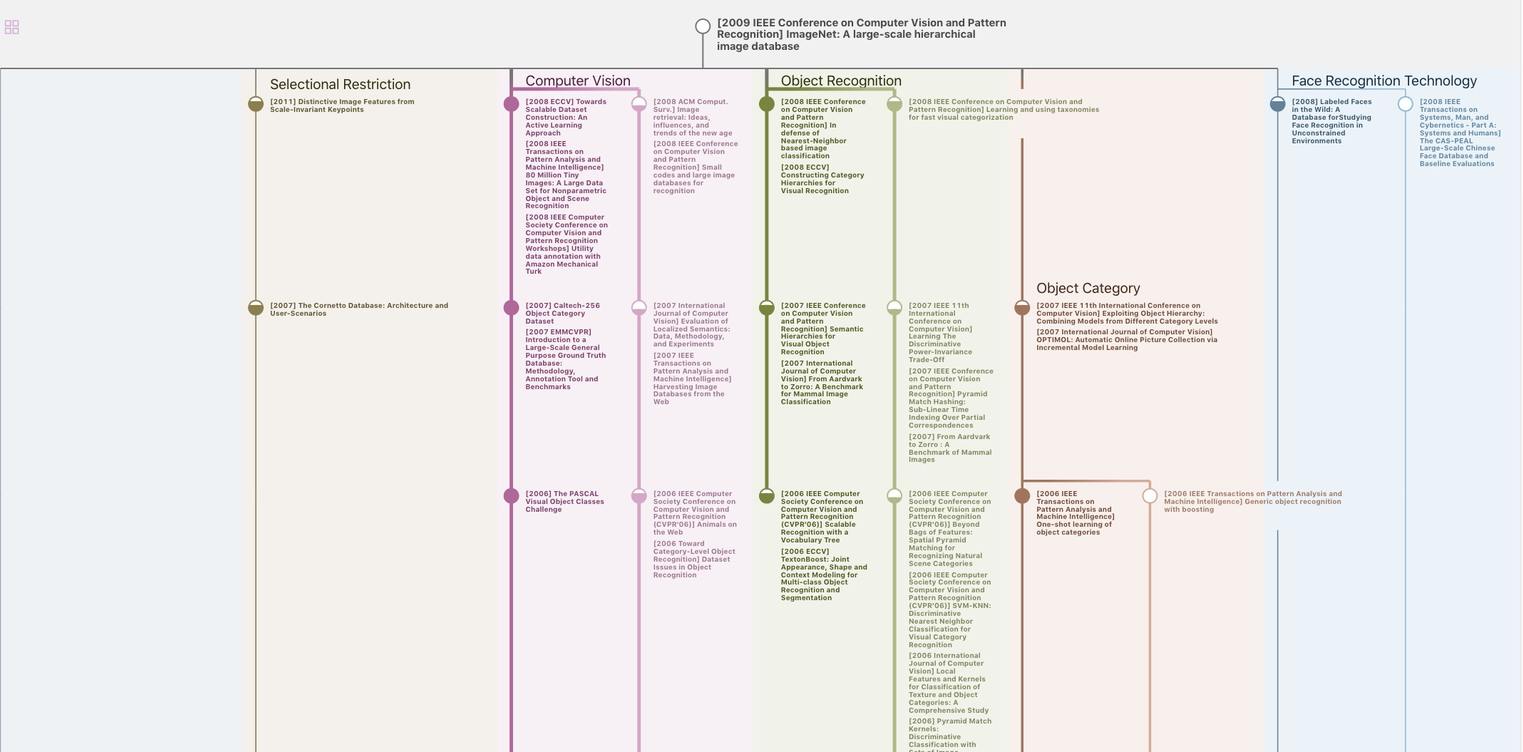
Generate MRT to find the research sequence of this paper
Chat Paper
Summary is being generated by the instructions you defined