Semantic Correlation Transfer for Heterogeneous Domain Adaptation.
IEEE transactions on neural networks and learning systems(2022)
摘要
Heterogeneous domain adaptation (HDA) is expected to achieve effective knowledge transfer from a label-rich source domain to a heterogeneous target domain with scarce labeled data. Most prior HDA methods strive to align the cross-domain feature distributions by learning domain invariant representations without considering the intrinsic semantic correlations among categories, which inevitably results in the suboptimal adaptation performance across domains. Therefore, to address this issue, we propose a novel semantic correlation transfer (SCT) method for HDA, which not only matches the marginal and conditional distributions between domains to mitigate the large domain discrepancy, but also transfers the category correlation knowledge underlying the source domain to target by maximizing the pairwise class similarity across source and target. Technically, the domainwise and classwise centroids (prototypes) are first computed and aligned according to the feature embeddings. Then, based on the derived classwise prototypes, we leverage the cosine similarity of each two classes in both domains to transfer the supervised source semantic correlation knowledge among different categories to target effectively. As a result, the feature transferability and category discriminability can be simultaneously improved during the adaptation process. Comprehensive experiments and ablation studies on standard HDA tasks, such as text-to-image, image-to-image, and text-to-text, have demonstrated the superiority of our proposed SCT against several state-of-the-art HDA methods.
更多查看译文
关键词
Semantics,Correlation,Prototypes,Training,Task analysis,Electronic mail,Transfer learning,Cross modal learning,feature fusion,heterogeneous domain adaptation (HDA),semantic correlation transfer (SCT)
AI 理解论文
溯源树
样例
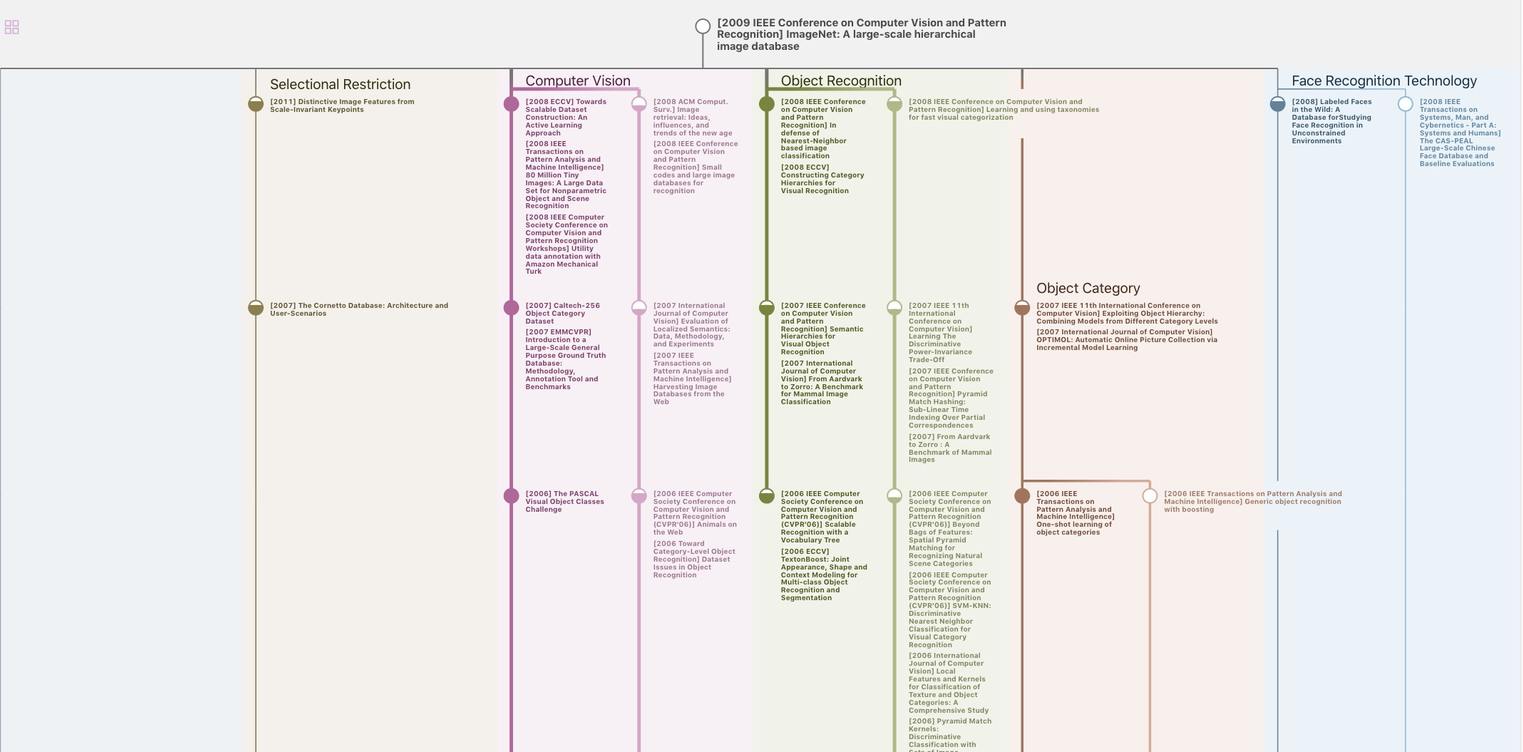
生成溯源树,研究论文发展脉络
Chat Paper
正在生成论文摘要