Utility of pre-treatment FDG PET/CT–derived machine learning models for outcome prediction in classical Hodgkin lymphoma
European Radiology(2022)
摘要
Objectives Relapse occurs in ~20% of patients with classical Hodgkin lymphoma (cHL) despite treatment adaption based on 2-deoxy-2-[ 18 F]fluoro- d -glucose positron emission tomography/computed tomography response. The objective was to evaluate pre-treatment FDG PET/CT–derived machine learning (ML) models for predicting outcome in patients with cHL. Methods All cHL patients undergoing pre-treatment PET/CT at our institution between 2008 and 2018 were retrospectively identified. A 1.5 × mean liver standardised uptake value (SUV) and a fixed 4.0 SUV threshold were used to segment PET/CT data. Feature extraction was performed using PyRadiomics with ComBat harmonisation. Training (80%) and test (20%) cohorts stratified around 2-year event-free survival (EFS), age, sex, ethnicity and disease stage were defined. Seven ML models were trained and hyperparameters tuned using stratified 5-fold cross-validation. Area under the curve (AUC) from receiver operator characteristic analysis was used to assess performance. Results A total of 289 patients (153 males), median age 36 (range 16–88 years), were included. There was no significant difference between training ( n = 231) and test cohorts ( n = 58) ( p value > 0.05). A ridge regression model using a 1.5 × mean liver SUV segmentation had the highest performance, with mean training, validation and test AUCs of 0.82 ± 0.002, 0.79 ± 0.01 and 0.81 ± 0.12. However, there was no significant difference between a logistic model derived from metabolic tumour volume and clinical features or the highest performing radiomic model. Conclusions Outcome prediction using pre-treatment FDG PET/CT–derived ML models is feasible in cHL patients. Further work is needed to determine optimum predictive thresholds for clinical use. Key points • A fixed threshold segmentation method led to more robust radiomic features. • A radiomic-based model for predicting 2-year event-free survival in classical Hodgkin lymphoma patients is feasible. • A predictive model based on ridge regression was the best performing model on our dataset.
更多查看译文
关键词
Hodgkin disease: positron emission tomography computed tomography,Machine learning, progression-free survival
AI 理解论文
溯源树
样例
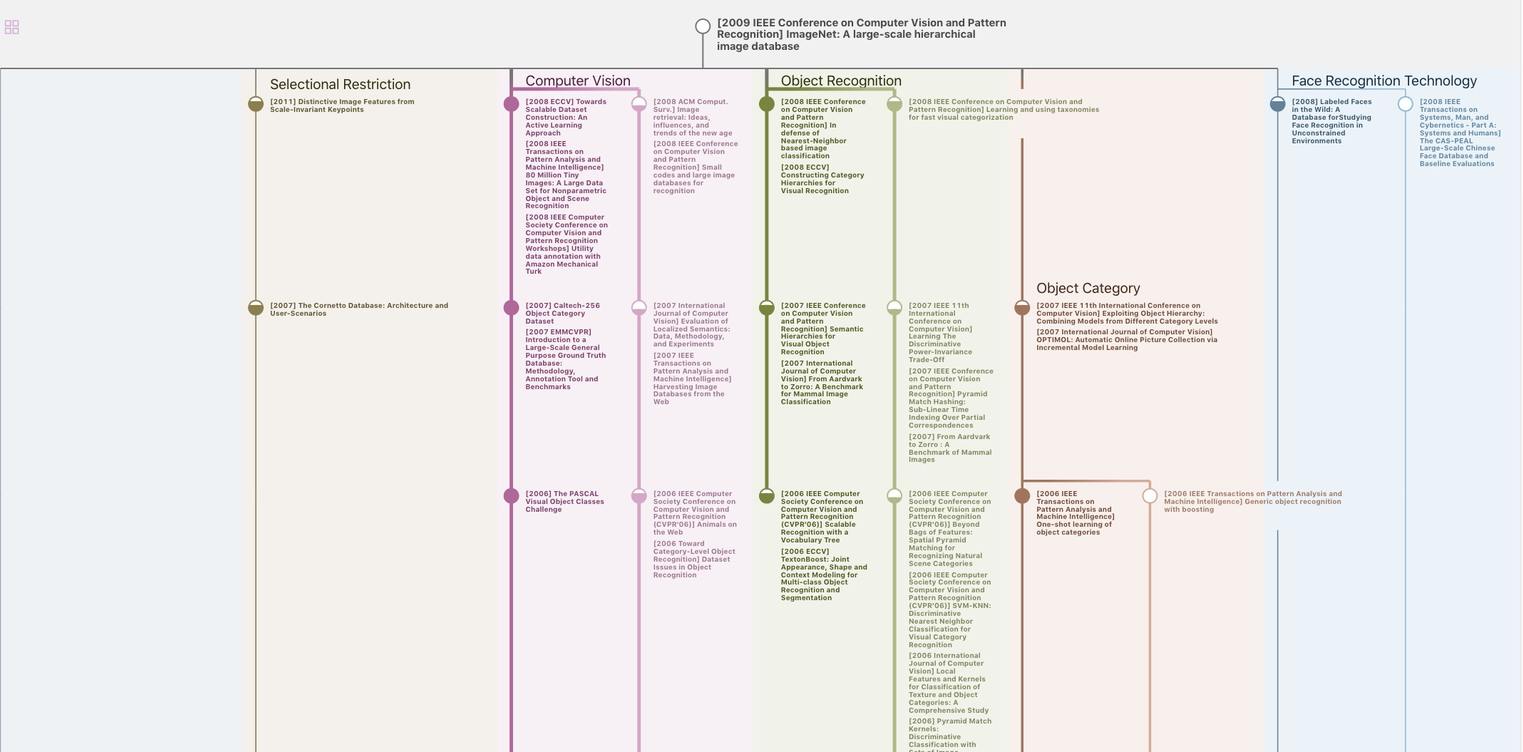
生成溯源树,研究论文发展脉络
Chat Paper
正在生成论文摘要