Deterministic Feature Decoupling By Surfing Invariance Manifolds
2020 IEEE INTERNATIONAL CONFERENCE ON ACOUSTICS, SPEECH, AND SIGNAL PROCESSING(2020)
摘要
We introduce a formalism that justifies and extends a heuristic method for algebraically decoupling deterministic features that recently proved useful for improving feature-based classification. Our new formalism is based on defining transformations inside manifolds, by following trajectories along the features' gradients. Through these transformations we define a normalization that, we demonstrate, allows for decoupling differentiable features. By applying this to sampling moments, we obtain a quasi-analytic solution for the orthokurtosis, a modification of the kurtosis that is not just decoupled from mean and variance, but also from skewness. After theoretically motivating feature decoupling for random data distributions, we illustrate with a regression problem example how decoupled features may perform significantly better than coupled ones.
更多查看译文
关键词
nonlinear orthogonal features, sample statistics, regression, manifolds, decoupled features, orthokurtosis
AI 理解论文
溯源树
样例
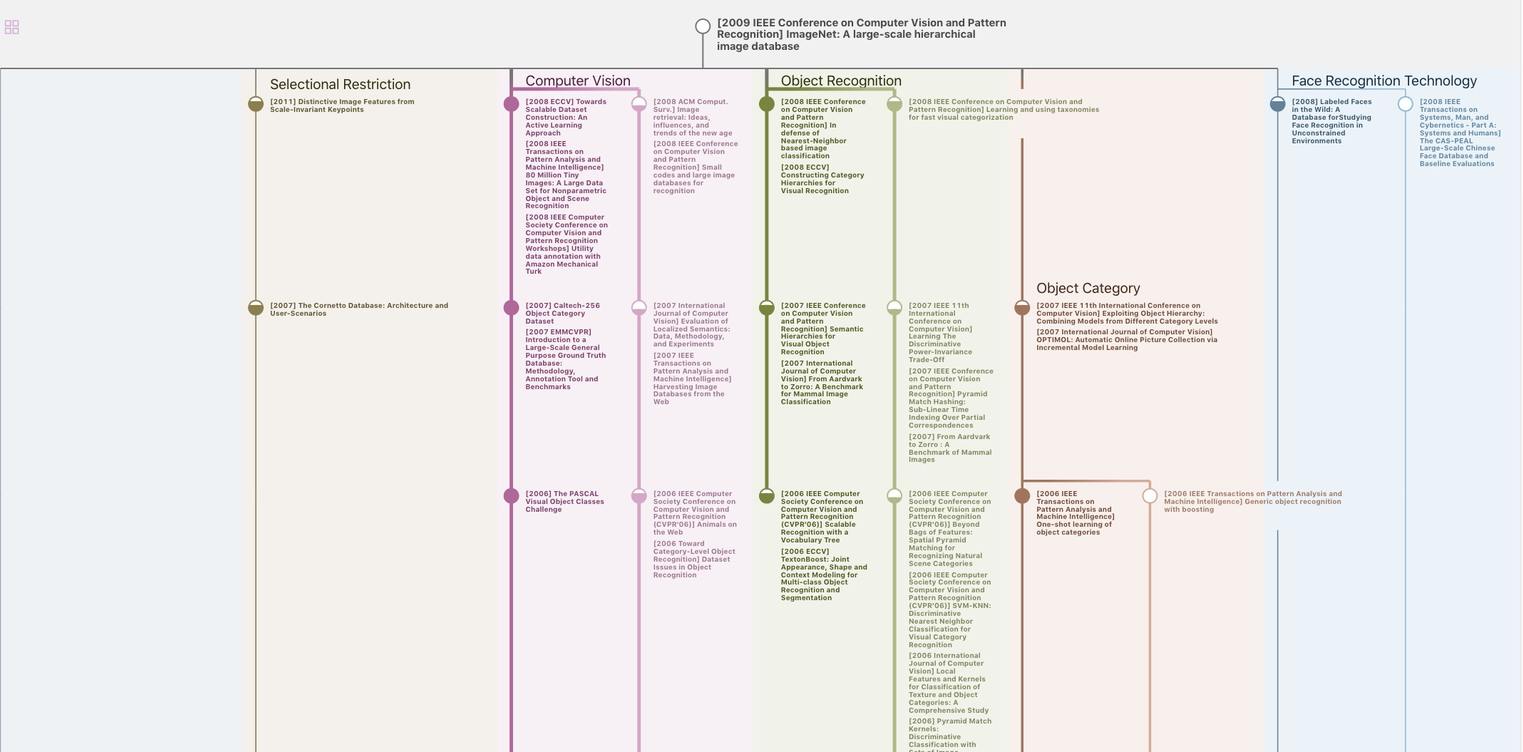
生成溯源树,研究论文发展脉络
Chat Paper
正在生成论文摘要