Difficulty and Contribution-Based Cooperative Coevolution for Large-Scale Optimization
IEEE TRANSACTIONS ON EVOLUTIONARY COMPUTATION(2023)
摘要
Cooperative coevolution (CC) is a paradigm equipped with the divide-and-conquer strategy for solving large-scale optimization problems (LSOPs). Currently, the computational resource allocation schemes of most CC could be divided into two categories, namely, equal allocation to all subproblems and preference allocation to the subproblems with a large contribution. However, the difficult subproblems are not carefully considered by the existing computational resource allocation schemes. For these subproblems, the investment of computational resources cannot quickly improve the fitness value, which leads to their small early contribution and being neglected. In this article, we comprehensively analyze the imbalanced nature of the subproblems from their difficulty and contribution in LSOPs. First, we propose a method to quantify the optimization difficulty of the problems during the evolution process, which considers both the difficulty of the fitness landscape and the behaviors of the optimization algorithm. Then, we propose a novel both difficulty and contribution-based CC framework, called DCCC, which encourages the allocation of the computational resources to more contributing and more difficult subproblems. DCCC is tested on the CEC'2010 and CEC'2013 large-scale optimization benchmarks, and is compared with several typical CC frameworks and state-of-the-art large-scale optimization algorithms. The experimental results demonstrate that DCCC is very competitive.
更多查看译文
关键词
Cooperative coevolution (CC),fitness-distance correlation (FDC),large-scale optimization
AI 理解论文
溯源树
样例
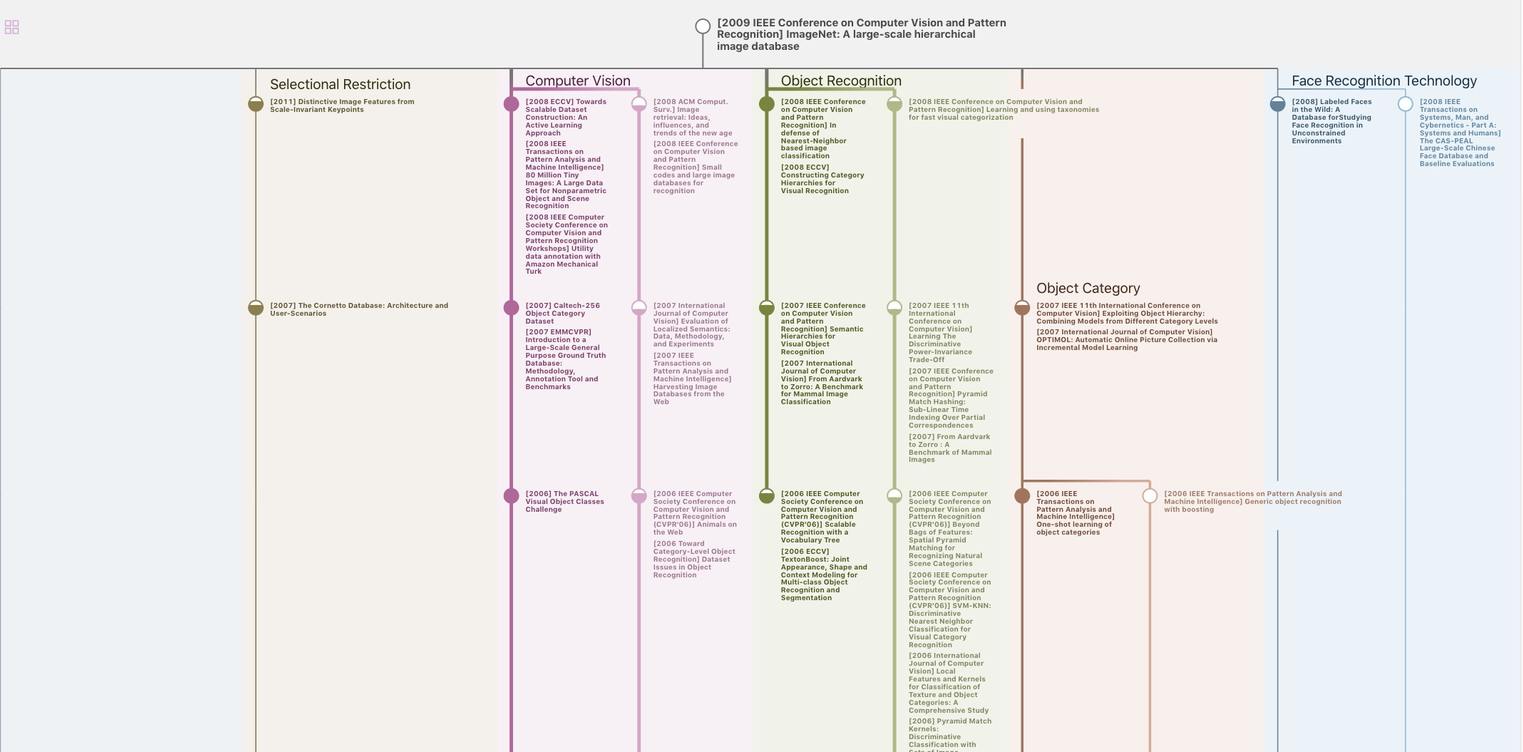
生成溯源树,研究论文发展脉络
Chat Paper
正在生成论文摘要