Fix-A-Step: Semi-supervised Learning from Uncurated Unlabeled Data
arXiv (Cornell University)(2023)
摘要
Semi-supervised learning (SSL) promises improved accuracy compared to training classifiers on small labeled datasets by also training on many unlabeled images. In real applications like medical imaging, unlabeled data will be collected for expediency and thus uncurated: possibly different from the labeled set in classes or features. Unfortunately, modern deep SSL often makes accuracy worse when given uncurated unlabeled data. Recent complex remedies try to detect out-of-distribution unlabeled images and then discard or downweight them. Instead, we introduce Fix-A-Step, a simpler procedure that views all uncurated unlabeled images as potentially helpful. Our first insight is that even uncurated images can yield useful augmentations of labeled data. Second, we modify gradient descent updates to prevent optimizing a multi-task SSL loss from hurting labeled-set accuracy. Fix-A-Step can repair many common deep SSL methods, improving accuracy on CIFAR benchmarks across all tested methods and levels of artificial class mismatch. On a new medical SSL benchmark called Heart2Heart, Fix-A-Step can learn from 353,500 truly uncurated ultrasound images to deliver gains that generalize across hospitals.
更多查看译文
关键词
uncurated unlabeled data,learning,fix-a-step,semi-supervised
AI 理解论文
溯源树
样例
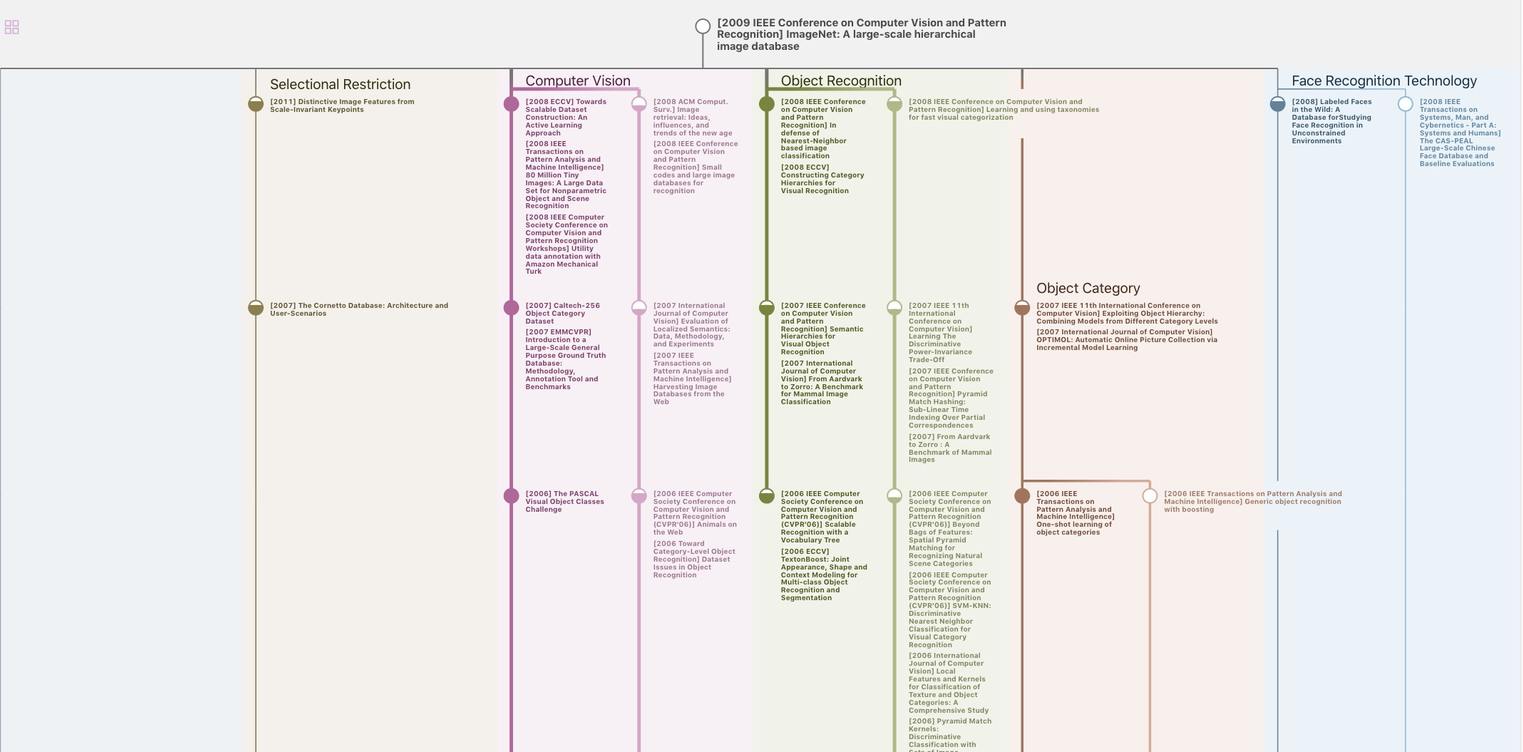
生成溯源树,研究论文发展脉络
Chat Paper
正在生成论文摘要