SW-GAN: Road Extraction from Remote Sensing Imagery Using Semi-Weakly Supervised Adversarial Learning
REMOTE SENSING(2022)
Abstract
Road networks play a fundamental role in our daily life. It is of importance to extract the road structure in a timely and precise manner with the rapid evolution of urban road structure. Recently, road network extraction using deep learning has become an effective and popular method. The main shortcoming of the road extraction using deep learning methods lies in the fact that there is a need for a large amount of training datasets. Additionally, the datasets need to be elaborately annotated, which is usually labor-intensive and time-consuming; thus, lots of weak annotations (such as the centerline from OpenStreetMap) have accumulated over the past a few decades. To make full use of the weak annotations, we propose a novel semi-weakly supervised method based on adversarial learning to extract road networks from remote sensing imagery. Our method uses a small set of pixel-wise annotated data and a large amount of weakly annotated data for training. The experimental results show that the proposed approach can achieve a maintained performance compared with the methods that use a large number of full pixel-wise annotations while using less fully annotated data.
MoreTranslated text
Key words
road extraction,semi-weakly supervised learning,Generative Adversarial Network,OpenStreetMap
AI Read Science
Must-Reading Tree
Example
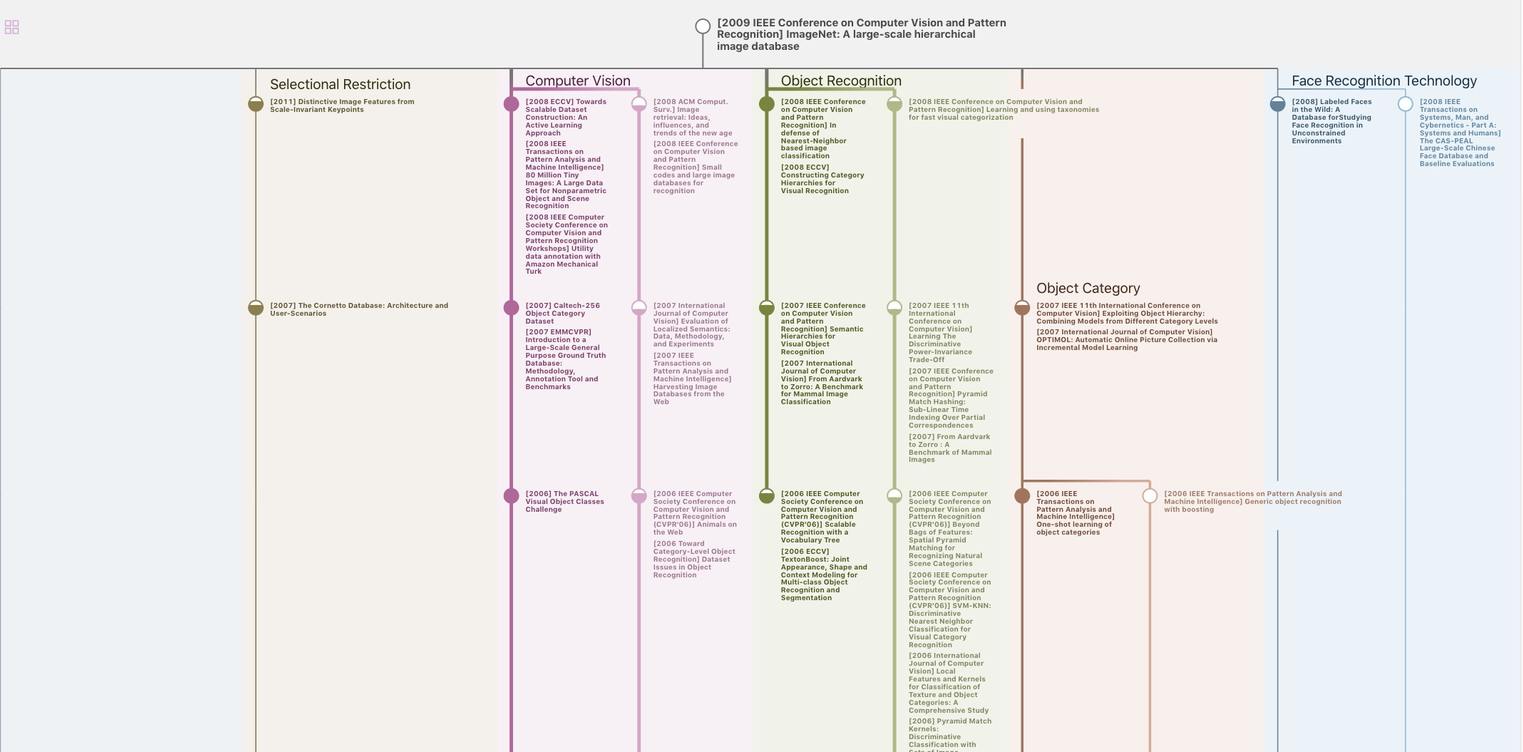
Generate MRT to find the research sequence of this paper
Chat Paper
Summary is being generated by the instructions you defined