Fast Nearest Convolution for Real-Time Efficient Image Super-Resolution.
CoRR(2022)
摘要
Deep learning-based single image super-resolution (SISR) approaches have drawn much attention and achieved remarkable success on modern advanced GPUs. However, most state-of-the-art methods require a huge number of parameters, memories, and computational resources, which usually show inferior inference times when applying them to current mobile device CPUs/NPUs. In this paper, we propose a simple plain convolution network with a fast nearest convolution module (NCNet), which is NPU-friendly and can perform a reliable super-resolution in real-time. The proposed nearest convolution has the same performance as the nearest upsampling but is much faster and more suitable for Android NNAPI. Our model can be easily deployed on mobile devices with 8-bit quantization and is fully compatible with all major mobile AI accelerators. Moreover, we conduct comprehensive experiments on different tensor operations on a mobile device to illustrate the efficiency of our network architecture. Our NCNet is trained and validated on the DIV2K 3x dataset, and the comparison with other efficient SR methods demonstrated that the NCNet can achieve high fidelity SR results while using fewer inference times. Our codes and pretrained models are publicly available at \url{https://github.com/Algolzw/NCNet}.
更多查看译文
AI 理解论文
溯源树
样例
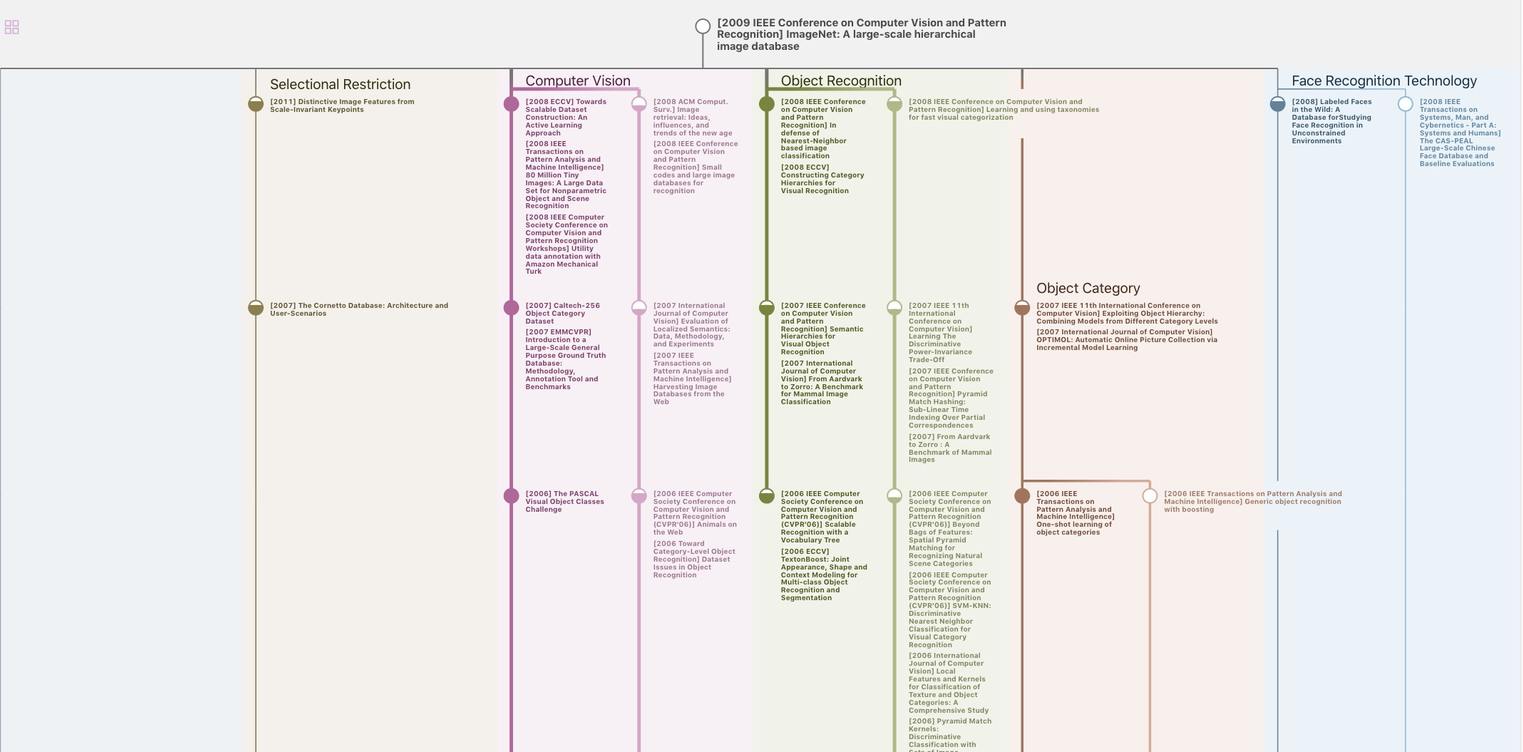
生成溯源树,研究论文发展脉络
Chat Paper
正在生成论文摘要