Deep Hyperspectral and Multispectral Image Fusion with Inter-image Variability
IEEE TRANSACTIONS ON GEOSCIENCE AND REMOTE SENSING(2023)
摘要
Hyperspectral and multispectral image fusion allows us to overcome the hardware limitations of hyperspectral imaging systems inherent to their lower spatial resolution. Nevertheless, existing algorithms usually fail to consider realistic image acquisition conditions. This paper presents a general imaging model that considers inter-image variability of data from heterogeneous sources and flexible image priors. The fusion problem is stated as an optimization problem in the maximum a posteriori framework. We introduce an original image fusion method that, on the one hand, solves the optimization problem accounting for inter-image variability with an iteratively reweighted scheme and, on the other hand, that leverages light-weight CNN-based networks to learn realistic image priors from data. In addition, we propose a zero-shot strategy to directly learn the image-specific prior of the latent images in an unsupervised manner. The performance of the algorithm is illustrated with real data subject to inter-image variability.
更多查看译文
关键词
Human-robot interaction, Image fusion, Hyperspectral imaging, Tensors, Spatial resolution, Optimization, Neural networks, Deep learning, hyperspectral data, image fusion, inter-image variability, multispectral data, zero-shot
AI 理解论文
溯源树
样例
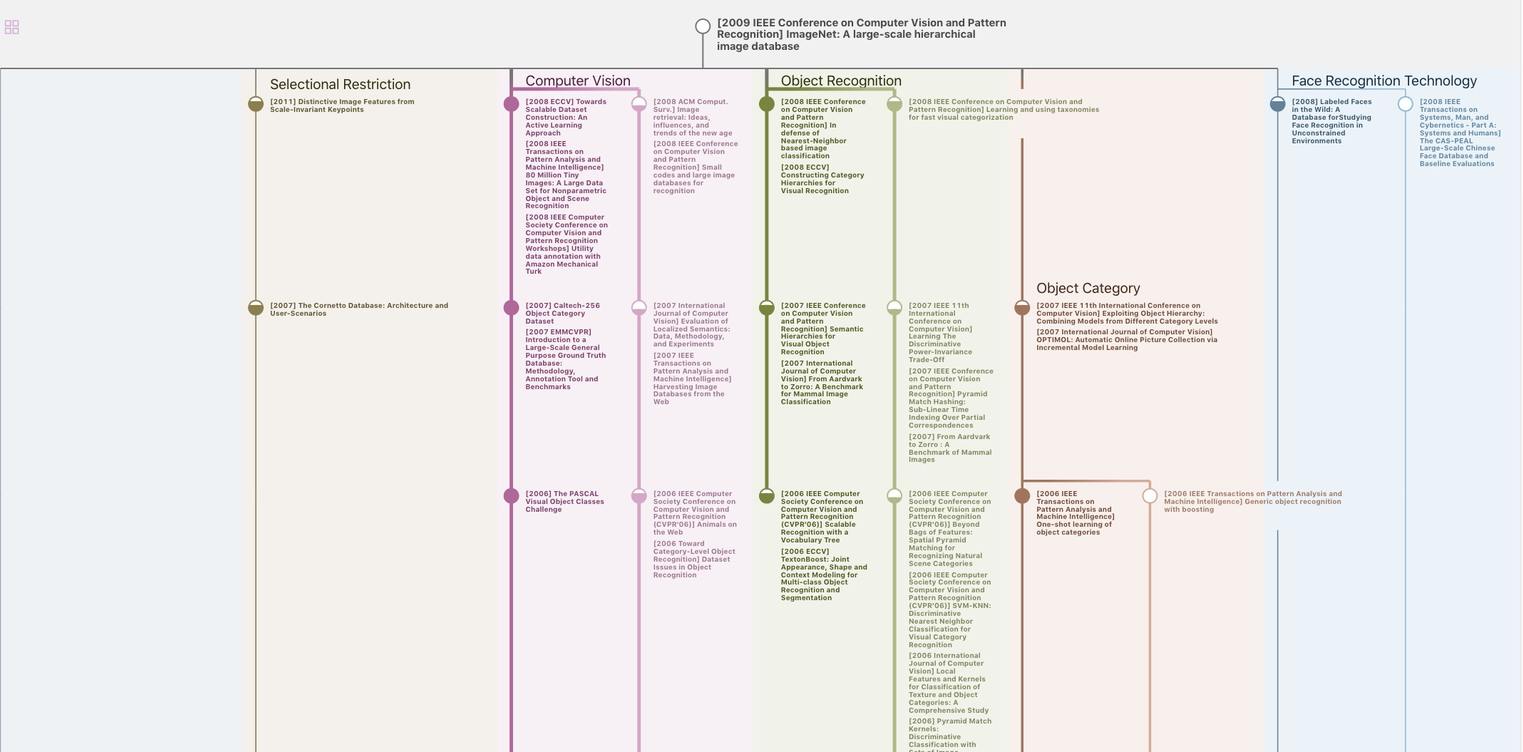
生成溯源树,研究论文发展脉络
Chat Paper
正在生成论文摘要