Multinomial Cluster-Weighted Models for High-Dimensional Data
arXiv (Cornell University)(2022)
Abstract
Modeling of high-dimensional data is very important to categorize different classes. We develop a new mixture model called Multinomial cluster-weighted model (MCWM). We derive the identifiability of a general class of MCWM. We estimate the proposed model through Expectation-Maximization (EM) algorithm via an iteratively reweighted least squares (EM-IRLS) and Stochastic Gradient Descent (EM-SGD). Model selection is carried out using different information criteria. Various Adjusted Rand Indices are considered as a different measure of accuracy. The clustering performance of the proposed model is investigated using simulated and real datasets. MCWM shows excellent clustering results via performance measures such as Accuracy and Area under the ROC curve.
MoreTranslated text
Key words
models,cluster-weighted,high-dimensional
AI Read Science
Must-Reading Tree
Example
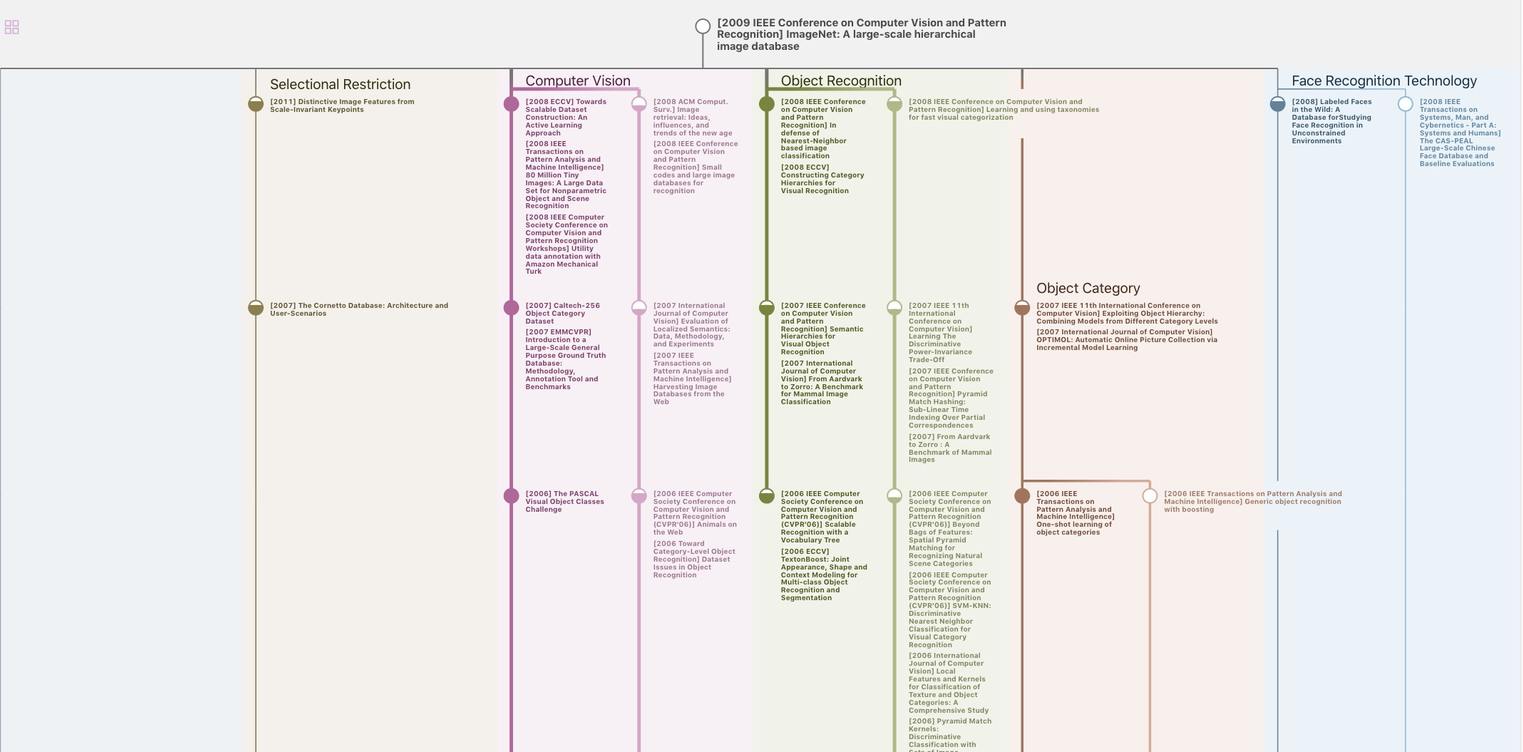
Generate MRT to find the research sequence of this paper
Chat Paper
Summary is being generated by the instructions you defined