Model Based Demand Order Estimation by Using Optimal Architecture Artificial Neural Network with Metaheuristic Optimizations
Journal of the Institute of Science and Technology(2022)
摘要
With the increase of e-commerce volumes in recent years, it is useful to estimate daily demand order numbers in order to improve the demand forecasts, production-distribution planning and sales services. In this manner, data-driven modeling and machine learning tools have been preferred to enhance demand order predictions, timely delivery, incomes and customer satisfaction in electronic trading because real-time data collection is possible in e-commerce platforms. Artificial Neural Networks (ANNs) are widely used for data-driven modeling and prediction problems. Since affecting the approximation performance of neural network function, the modeling performance of ANNs strongly depends on the architecture of neural networks, and architectural optimization of ANN models has become a main topic in the neuroevolution field. This study presents an architecture optimization method that implements Particle Swarm Optimization (PSO) and Differential Evolution (DE) algorithms to optimize ANN model architecture for the estimation of total demand order numbers from the sparse demand order data. In this approach, PSO and DE algorithm only optimizes neural model architecture according to an effective network search policy and the training of ANN models is carried out by using backpropagation algorithm. This neural architecture model optimization approach considers generalization of data, reducing neuron and training epoch numbers and it can yield an optimal architecture data-driven neural model for estimation of the daily total orders. In the experimental study, optimal architecture ANN models are obtained according to the daily order forecasting dataset.
更多查看译文
关键词
neural network,order,model
AI 理解论文
溯源树
样例
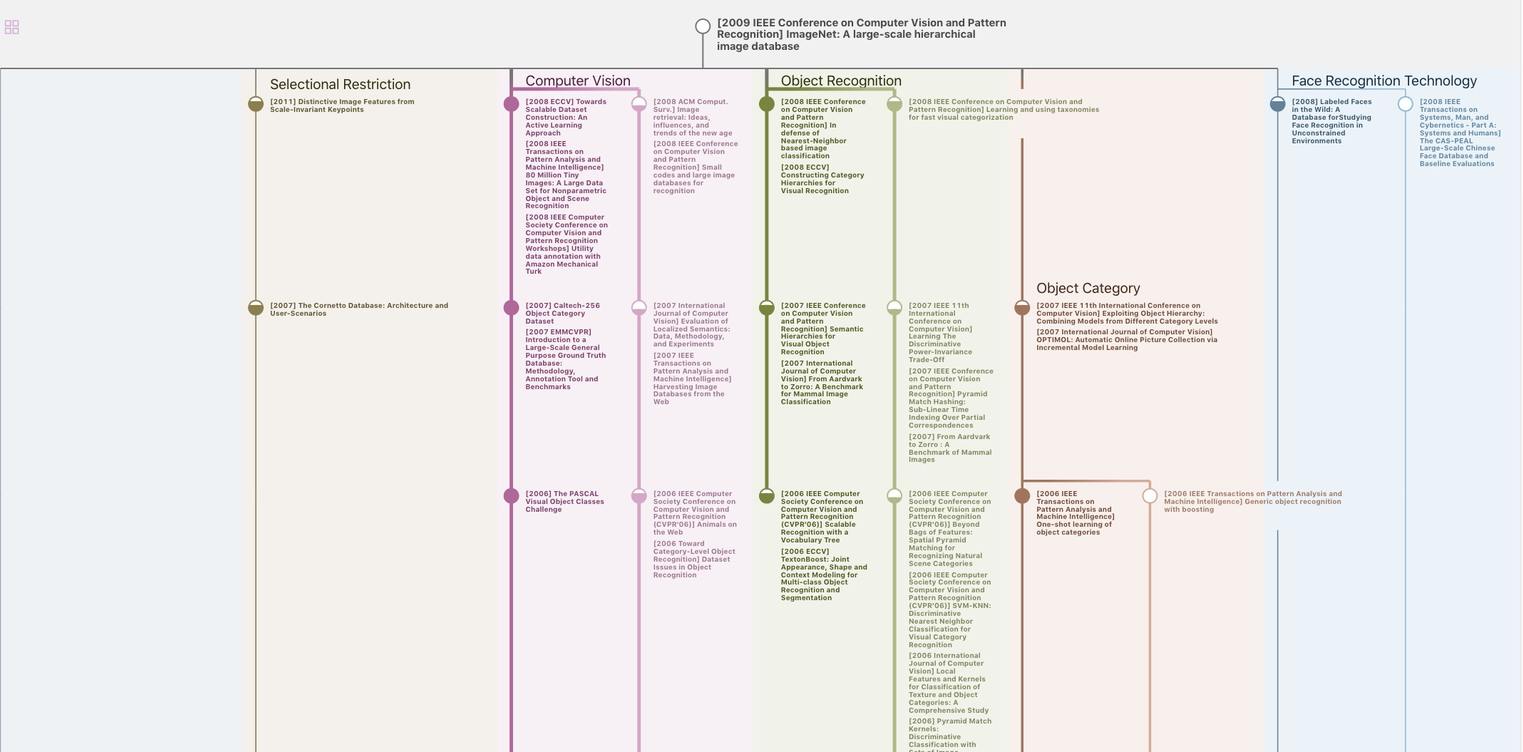
生成溯源树,研究论文发展脉络
Chat Paper
正在生成论文摘要