Accelerating and pruning CNNs for semantic segmentation on FPGA
Proceedings of the 59th ACM/IEEE Design Automation Conference(2022)
摘要
Semantic segmentation is one of the popular tasks in computer vision, providing pixel-wise annotations for scene understanding. However, segmentation-based convolutional neural networks require tremendous computational power. In this work, a fullypipelined hardware accelerator with support for dilated convolution is introduced, which cuts down the redundant zero multiplications. Furthermore, we propose a genetic algorithm based automated channel pruning technique to jointly optimize computational complexity and model accuracy. Finally, hardware heuristics and an accurate model of the custom accelerator design enable a hardware-aware pruning framework. We achieve 2.44x lower latency with minimal degradation in semantic prediction quality (-1.98 pp lower mean intersection over union) compared to the baseline DeepLabV3+ model, evaluated on an Arria-10 FPGA. The binary files of the FPGA design, baseline and pruned models can be found in github.com/pierpaolomori/SemanticSegmentationFPGA
更多查看译文
关键词
semantic segmentation,cnns
AI 理解论文
溯源树
样例
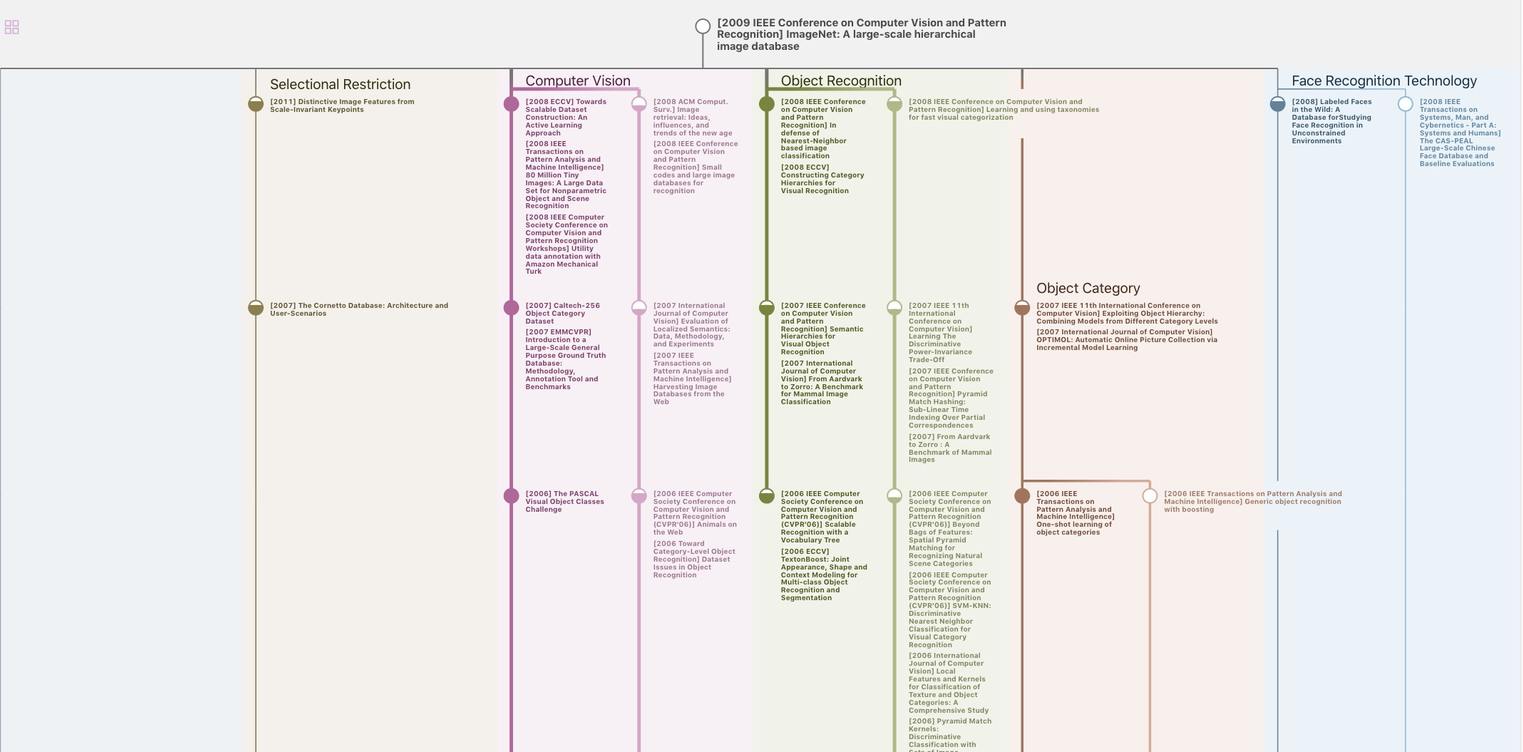
生成溯源树,研究论文发展脉络
Chat Paper
正在生成论文摘要