Development of a Model Predicting the Outcome of In Vitro Fertilization Cycles by a Robust Decision Tree Method
FRONTIERS IN ENDOCRINOLOGY(2022)
摘要
IntroductionInfertility is a worldwide problem. To evaluate the outcome of in vitro fertilization (IVF) treatment for infertility, many indicators need to be considered and the relation among indicators need to be studied. ObjectivesTo construct an IVF predicting model by a robust decision tree method and find important factors and their interrelation. MethodsIVF and intracytoplasmic sperm injection (ICSI) cycles between January 2010 and December 2020 in a women's hospital were collected. Comprehensive evaluation and examination of patients, specific therapy strategy and the outcome of treatment were recorded. Variables were selected through the significance of 1-way analysis between the clinical pregnant group and the nonpregnant group and then were discretized. Then, gradient boosting decision tree (GBDT) was used to construct the model to compute the score for predicting the rate of clinical pregnancy. ResultThirty-eight variables with significant difference were selected for binning and thirty of them in which the pregnancy rate varied in different categories were chosen to construct the model. The final score computed by model predicted the clinical pregnancy rate well with the Area Under Curve (AUC) value achieving 0.704 and the consistency reaching 98.1%. Number of two-pronuclear embryo (2PN), age of women, AMH level, number of oocytes retrieved and endometrial thickness were important factors related to IVF outcome. Moreover, some interrelations among factors were found from model, which may assist clinicians in making decisions. ConclusionThis study constructed a model predicting the outcome of IVF cycles through a robust decision tree method and achieved satisfactory prediction performance. Important factors related to IVF outcome and some interrelations among factors were found.
更多查看译文
关键词
in-vitro fertilization,artificial intelligence,prediction model,feature discretization,clinical pregnancy
AI 理解论文
溯源树
样例
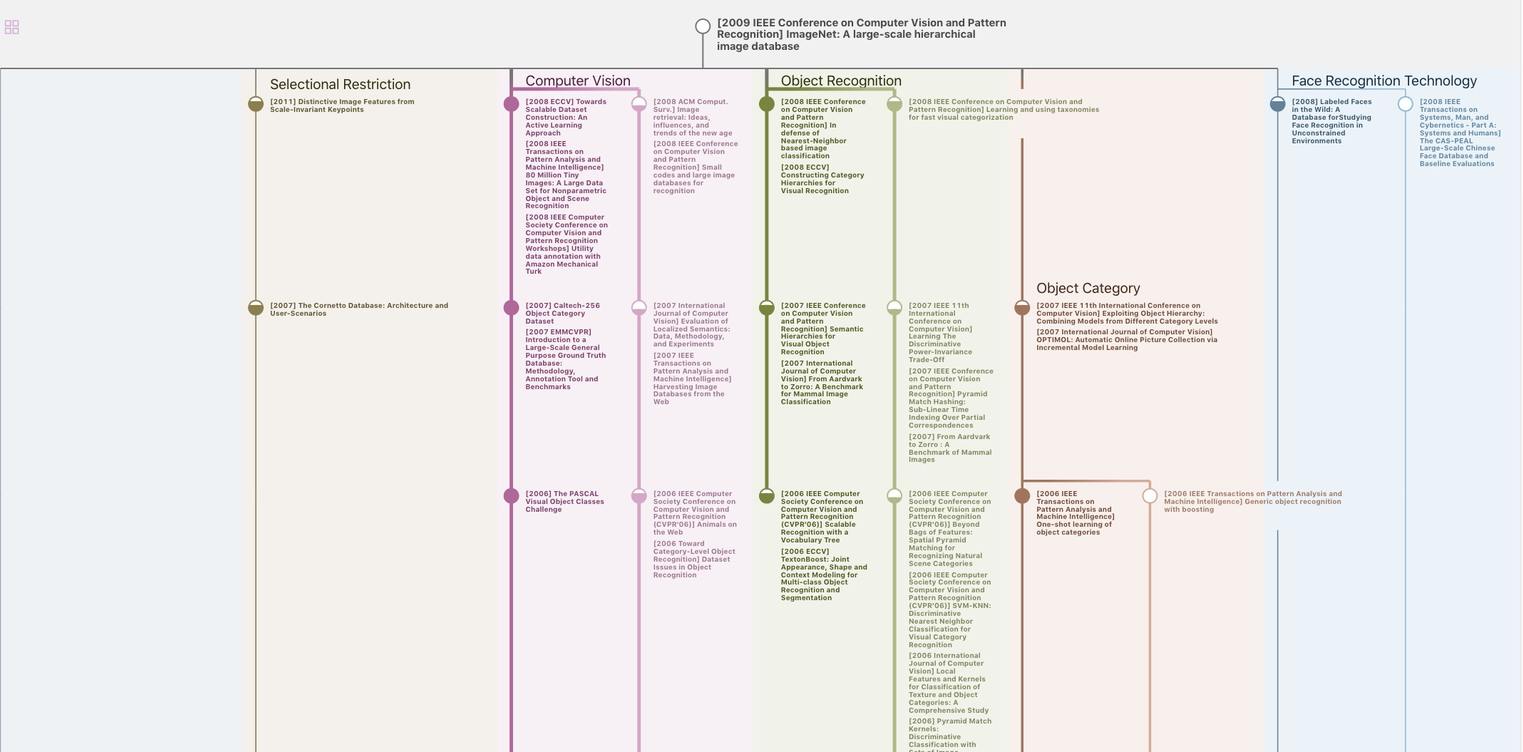
生成溯源树,研究论文发展脉络
Chat Paper
正在生成论文摘要