Multi-task convolutional neural networks for predicting in vitro clearance endpoints from molecular images
Journal of Computer-Aided Molecular Design(2022)
Abstract
Optimization of compound metabolic stability is a highly topical issue in pharmaceutical research. Accordingly, application of predictive in silico models can potentially reduce the number of design-make-test-analyze iterations and consequently speed up the progression of novel candidate molecules. Herein, we have investigated the question if multiple in vitro clearance endpoints could be accurately predicted from image-based molecular representations. Thus, compound measurements for four commonly investigated clearance endpoints were curated from AstraZeneca internal sources, providing a sound basis for building multi-task convolutional neural network models. Application of several increasingly challenging data splitting strategies confirmed that convolutional neural network models were successful at capturing implicit chemical relationships contained in training and test data, similar to what is commonly observed for structural fingerprints. Furthermore, model benchmarking against state-of-the-art machine learning methods, including deep neural networks and graph convolutional neural networks, trained with structure- and graph-based representations, respectively, revealed on par or increased accuracy of convolutional neural networks with clear benefit of multi-task learning across all clearance endpoints. Our findings indicate that image-based molecular representations can be applied to predict multiple clearance endpoints, suggesting a potential follow-up to investigate model interpretability from molecular images.
MoreTranslated text
Key words
Metabolic stability, Compound clearance, Molecular images, Convolutional neural networks, Deep learning, Pharmacokinetics
AI Read Science
Must-Reading Tree
Example
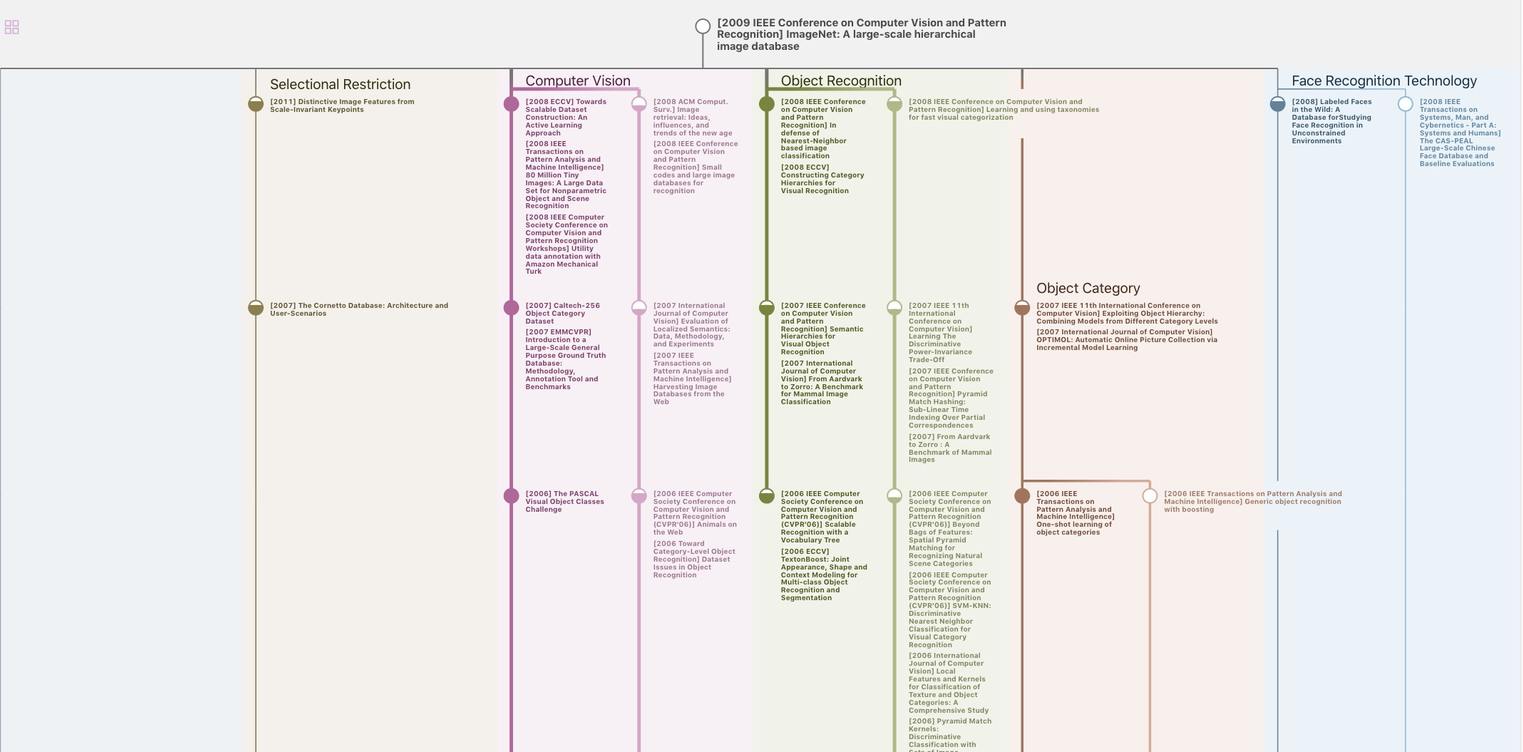
Generate MRT to find the research sequence of this paper
Chat Paper
Summary is being generated by the instructions you defined