A soft-target difference scaling network via relational knowledge distillation for fault detection of liquid rocket engine under multi-source trouble-free samples
RELIABILITY ENGINEERING & SYSTEM SAFETY(2022)
摘要
The detection of anomalies in liquid rocket motors is a challenge at this stage. On the one hand, numerous key components and extreme working environment easily leads to multi-source, strong nonlinear and non-stationary characteristics of monitoring data. On the other hand, the difficulties of fault detection are further aggravated by the few fault monitoring data during equipment acceptance period. In view of the above problems of engine working state identification, this paper takes the hot commissioning data as the research object to carry out the study about the intelligent fault detection of liquid rocket engine. Firstly, the original data is reconstructed by hierarchical task training. Then the soft target of rocket engine samples is constructed, which is used to define the sample distribution range. The soft target difference scaling method is specially designed to assist relevant knowledge extraction. Combining with metric learning, the fault prototype features are constructed to calculate the engine state discrimination threshold. Finally, the state identification without fault samples is realized by integrating the above methods. Multiple sets of measured data of liquid rocket engines are analyzed and dis-cussed to verify the feasibility and effectiveness of the proposed method.
更多查看译文
关键词
Fault detection,Liquid rocket engine,Soft target,Metric learning,Trouble -free Samples
AI 理解论文
溯源树
样例
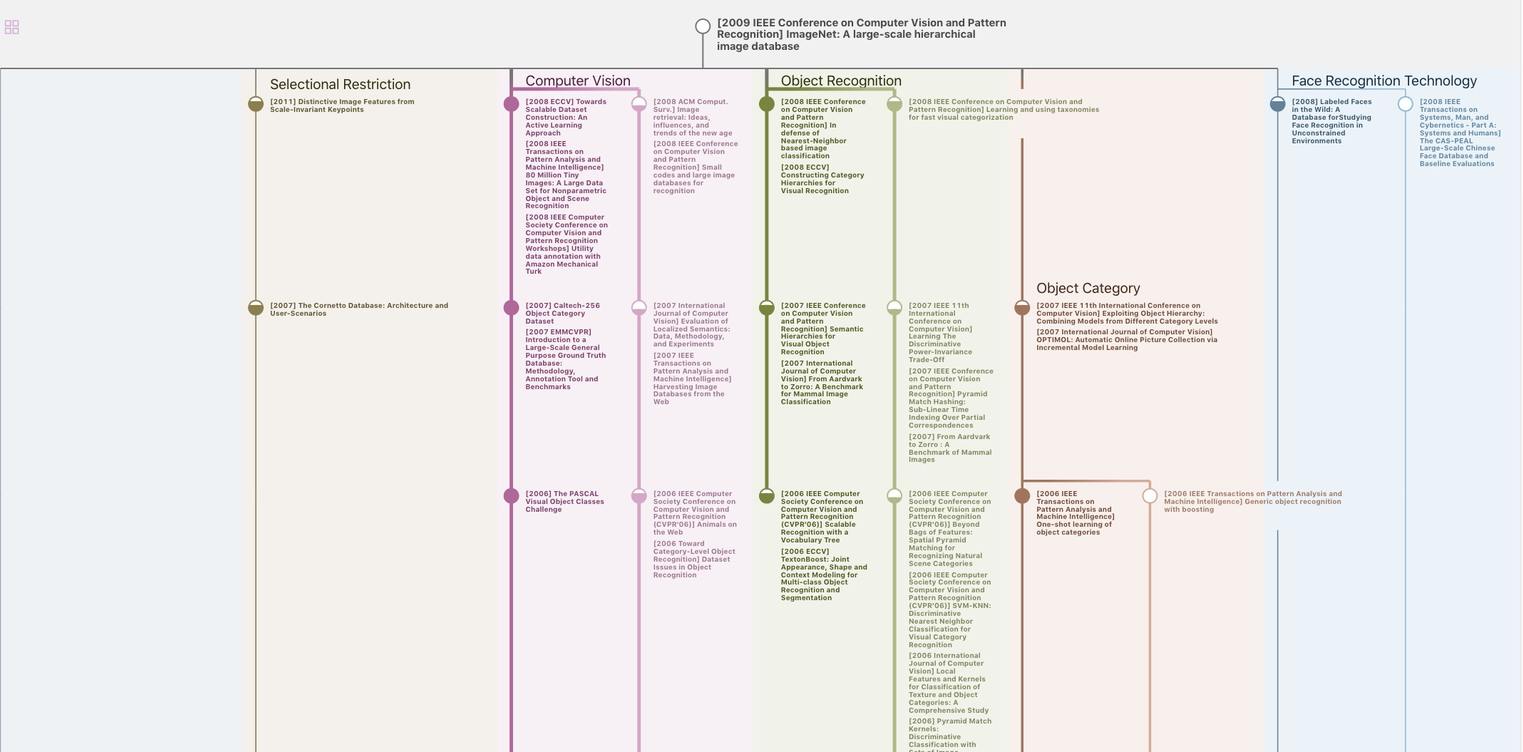
生成溯源树,研究论文发展脉络
Chat Paper
正在生成论文摘要