Selection Collider Bias in Large Language Models
ICLR 2023(2022)
摘要
In this paper we motivate the causal mechanisms behind sample selection induced collider bias (selection collider bias) that can cause Large Language Models (LLMs) to learn unconditional dependence between entities that are unconditionally independent in the real world. We show that selection collider bias can become amplified in underspecified learning tasks, and although difficult to overcome, we describe a method to exploit the resulting spurious correlations for determination of when a model may be uncertain about its prediction. We demonstrate an uncertainty metric that matches human uncertainty in tasks with gender pronoun underspecification on an extended version of the Winogender Schemas evaluation set, and we provide an online demo where users can apply our uncertainty metric to their own texts and models.
更多查看译文
关键词
large language models,causal inference,selection bias
AI 理解论文
溯源树
样例
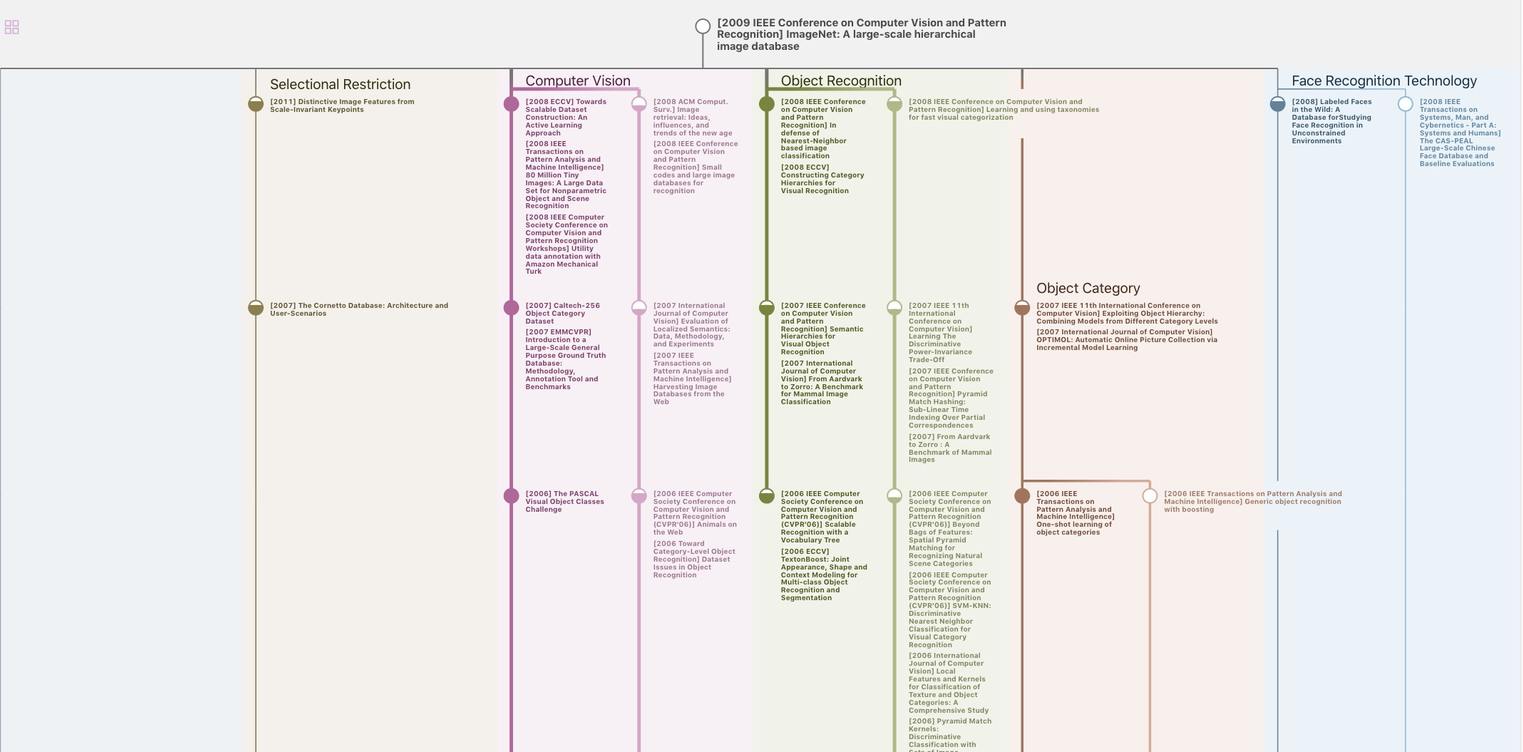
生成溯源树,研究论文发展脉络
Chat Paper
正在生成论文摘要