A Graphical Model for Fusing Diverse Microbiome Data
IEEE TRANSACTIONS ON SIGNAL PROCESSING(2023)
摘要
This paper develops a Bayesian graphical model for fusing disparate types of count data. The motivating application is the study of bacterial communities from diverse high-dimensional features, in this case, transcripts, collected from different treatments. In such datasets, there are no explicit correspondences between the communities and each corresponds to different factors, making data fusion challenging. We introduce a flexible multinomial-Gaussian generative model for jointly modeling such count data. This latent variable model jointly characterizes the observed data through a common multivariate Gaussian latent space that parameterizes the set of multinomial probabilities of the transcriptome counts. The covariance matrix of the latent variables induces a covariance matrix of co-dependencies between all the transcripts, effectively fusing multiple data sources. We present a computationally scalable variational Expectation-Maximization (EM) algorithm for inferring the latent variables and the parameters of the model. The inferred latent variables provide a common dimensionality reduction for visualizing the data and the inferred parameters provide a predictive posterior distribution. In addition to simulation studies that demonstrate the variational EM procedure, we apply our model to a bacterial microbiome dataset.
更多查看译文
关键词
Bayesian probabilistic graphical model,data fusion,microbial data analysis,variational optimization
AI 理解论文
溯源树
样例
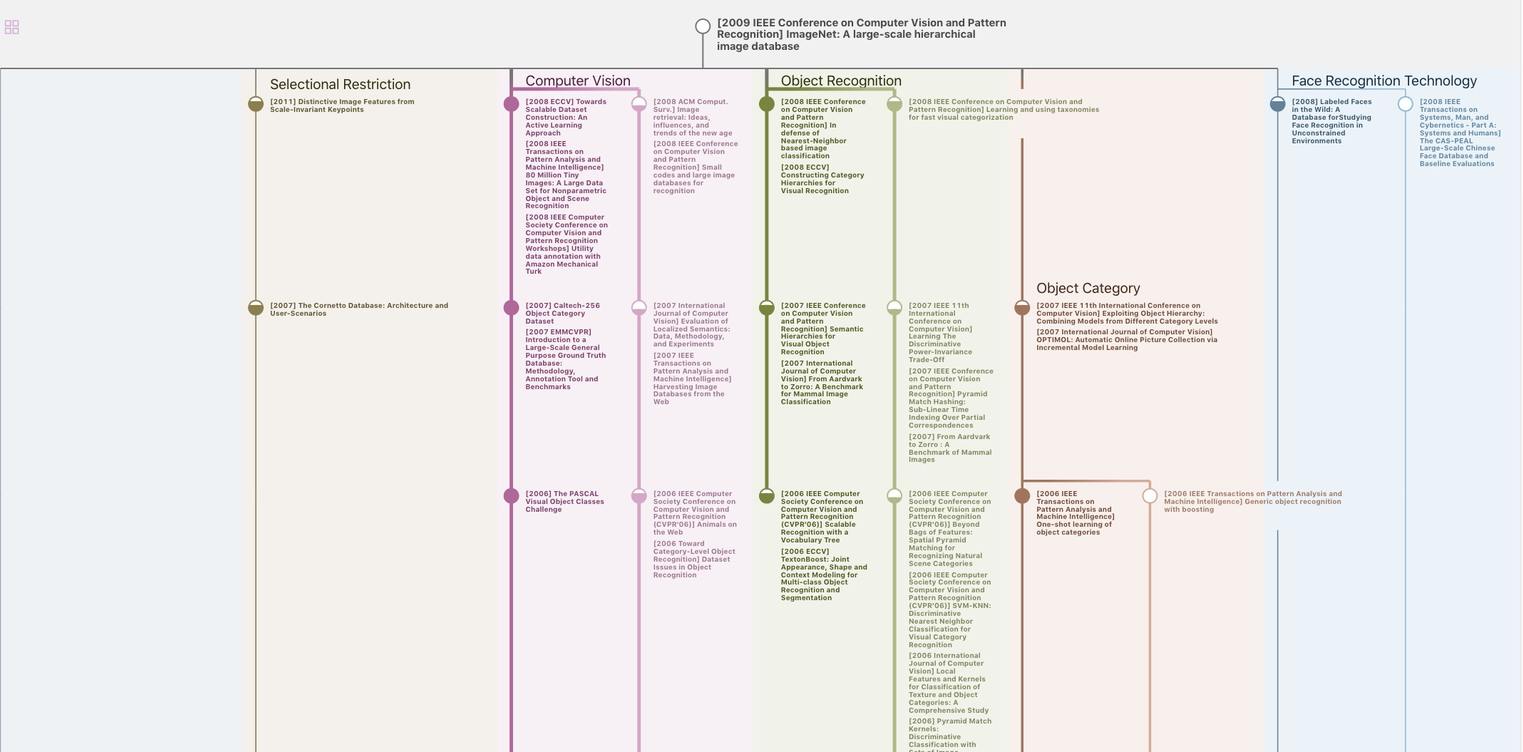
生成溯源树,研究论文发展脉络
Chat Paper
正在生成论文摘要