The Saddle-Point Accountant for Differential Privacy
arxiv(2022)
摘要
We introduce a new differential privacy (DP) accountant called the saddle-point accountant (SPA). SPA approximates privacy guarantees for the composition of DP mechanisms in an accurate and fast manner. Our approach is inspired by the saddle-point method -- a ubiquitous numerical technique in statistics. We prove rigorous performance guarantees by deriving upper and lower bounds for the approximation error offered by SPA. The crux of SPA is a combination of large-deviation methods with central limit theorems, which we derive via exponentially tilting the privacy loss random variables corresponding to the DP mechanisms. One key advantage of SPA is that it runs in constant time for the $n$-fold composition of a privacy mechanism. Numerical experiments demonstrate that SPA achieves comparable accuracy to state-of-the-art accounting methods with a faster runtime.
更多查看译文
关键词
differential privacy,saddle-point
AI 理解论文
溯源树
样例
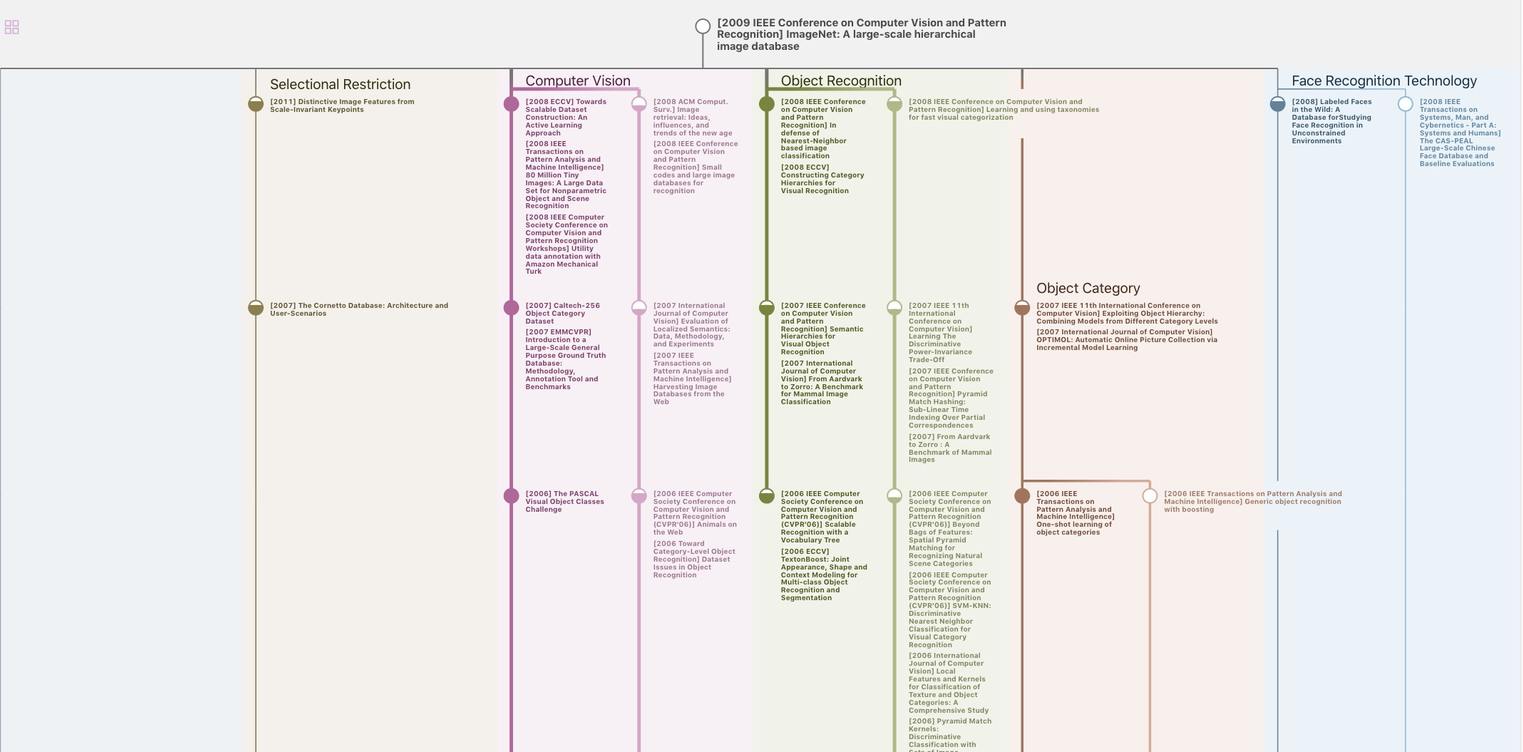
生成溯源树,研究论文发展脉络
Chat Paper
正在生成论文摘要