Data-Driven Causal Effect Estimation Based on Graphical Causal Modelling: A Survey
ACM COMPUTING SURVEYS(2024)
摘要
In many fields of scientific research and real-world applications, unbiased estimation of causal effects from non-experimental data is crucial for understanding the mechanism underlying the data and for decision-making on effective responses or interventions. A great deal of research has been conducted to address this challenging problem from different angles. For estimating causal effect in observational data, assumptions such as Markov condition, faithfulness, and causal sufficiency are always made. Under the assumptions, full knowledge, such as a set of covariates or an underlying causal graph, is typically required. A practical challenge is that in many applications, no such full knowledge or only some partial knowledge is available. In recent years, research has emerged to use search strategies based on graphical causal modelling to discover useful knowledge from data for causal effect estimation, with some mild assumptions, and has shown promise in tackling the practical challenge. In this survey, we reviewthese data-drivenmethods on causal effect estimation for a single treatment with a single outcome of interest and focus on the challenges faced by data-driven causal effect estimation. We concisely summarise the basic concepts and theories that are essential for data-driven causal effect estimation using graphical causal modelling but are scattered around the literature. We identify and discuss the challenges faced by data-driven causal effect estimation and characterise the existing methods by their assumptions and the approaches to tackling the challenges. We analyse the strengths and limitations of the different types of methods and present an empirical evaluation to support the discussions. We hope this review will motivate more researchers to design better data-driven methods based on graphical causal modelling for the challenging problem of causal effect estimation.
更多查看译文
关键词
Causal inference,causality,graphical causal model,causal effect estimation,latent confounders,instrumental variable
AI 理解论文
溯源树
样例
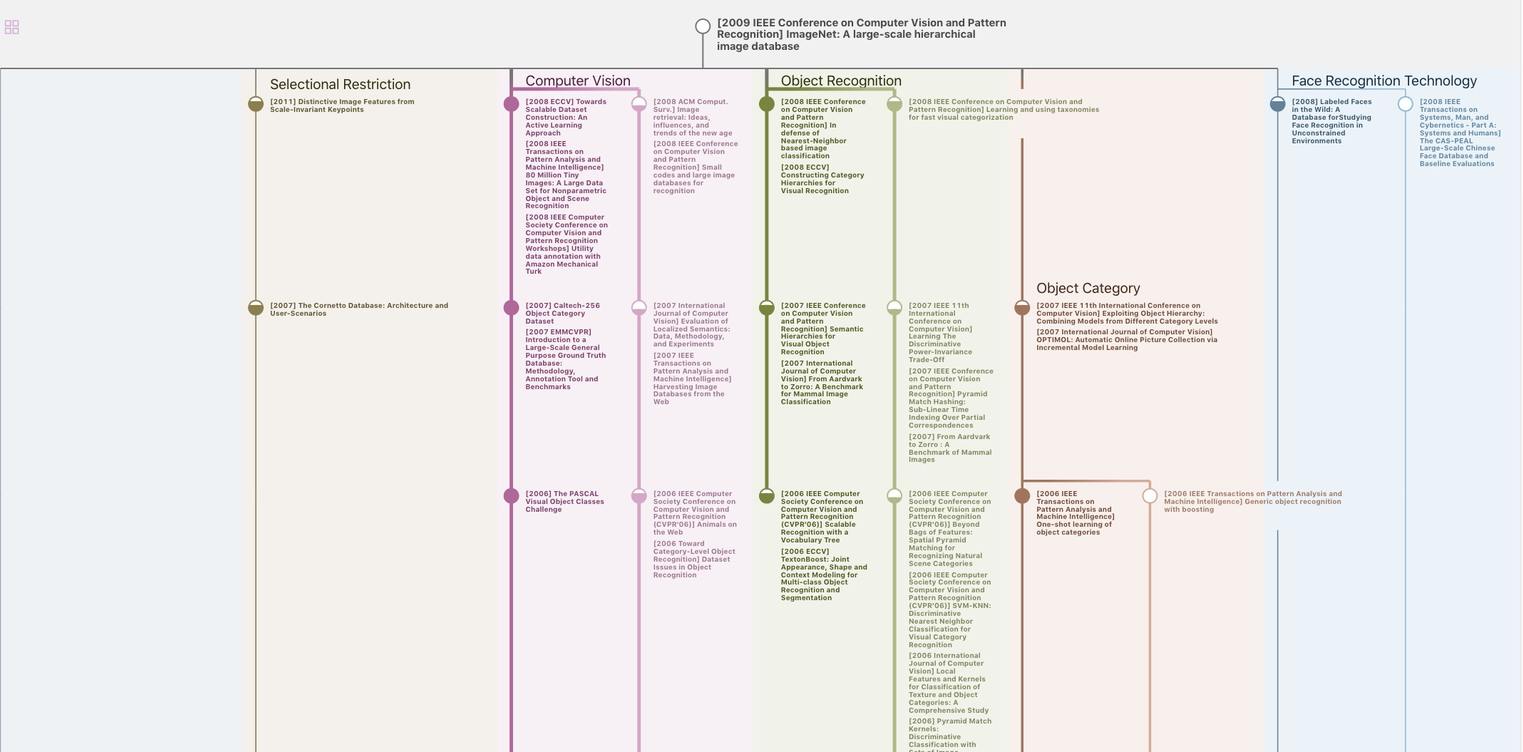
生成溯源树,研究论文发展脉络
Chat Paper
正在生成论文摘要