Blurring cluster randomized trials and observational studies using Two-Stage TMLE to address sub-sampling, missingness, and minimal independent units
arXiv (Cornell University)(2022)
摘要
Cluster randomized trials (CRTs) often enroll large numbers of participants, but due to logistical and fiscal challenges, only a subset of participants may be selected for measurement of certain outcomes, and those sampled may, purposely or not, be unrepresentative of all participants. Missing data also present a challenge: if sampled individuals with measured outcomes are dissimilar from those with missing outcomes, unadjusted estimates of arm-specific outcomes and the intervention effect may be biased. Further, CRTs often enroll and randomize few clusters by necessity, limiting statistical power and raising concerns about finite sample performance. Motivated by a sub-study of the SEARCH community randomized trial on the incidence of TB infection, we demonstrate interlocking methods to handle these challenges. First, we extend Two-Stage targeted minimum loss-based estimation (TMLE) to account for three sources of missingness: (1) sampling for the sub-study; (2) measurement of baseline status among those sampled, and (3) measurement of final status among those in the incidence cohort (i.e., persons known to be at risk at baseline). Second, we critically evaluate the assumptions under which sub-units of the cluster can be considered the conditionally independent unit, improving precision and statistical power but also causing the CRT to behave more like an observational study. Our application to the SEARCH highlights the impact of different assumptions on measurement and dependence as well as the real-life gains of our approach for bias reduction and efficiency improvement.
更多查看译文
关键词
observational studies,trials,cluster,two-stage,sub-sampling
AI 理解论文
溯源树
样例
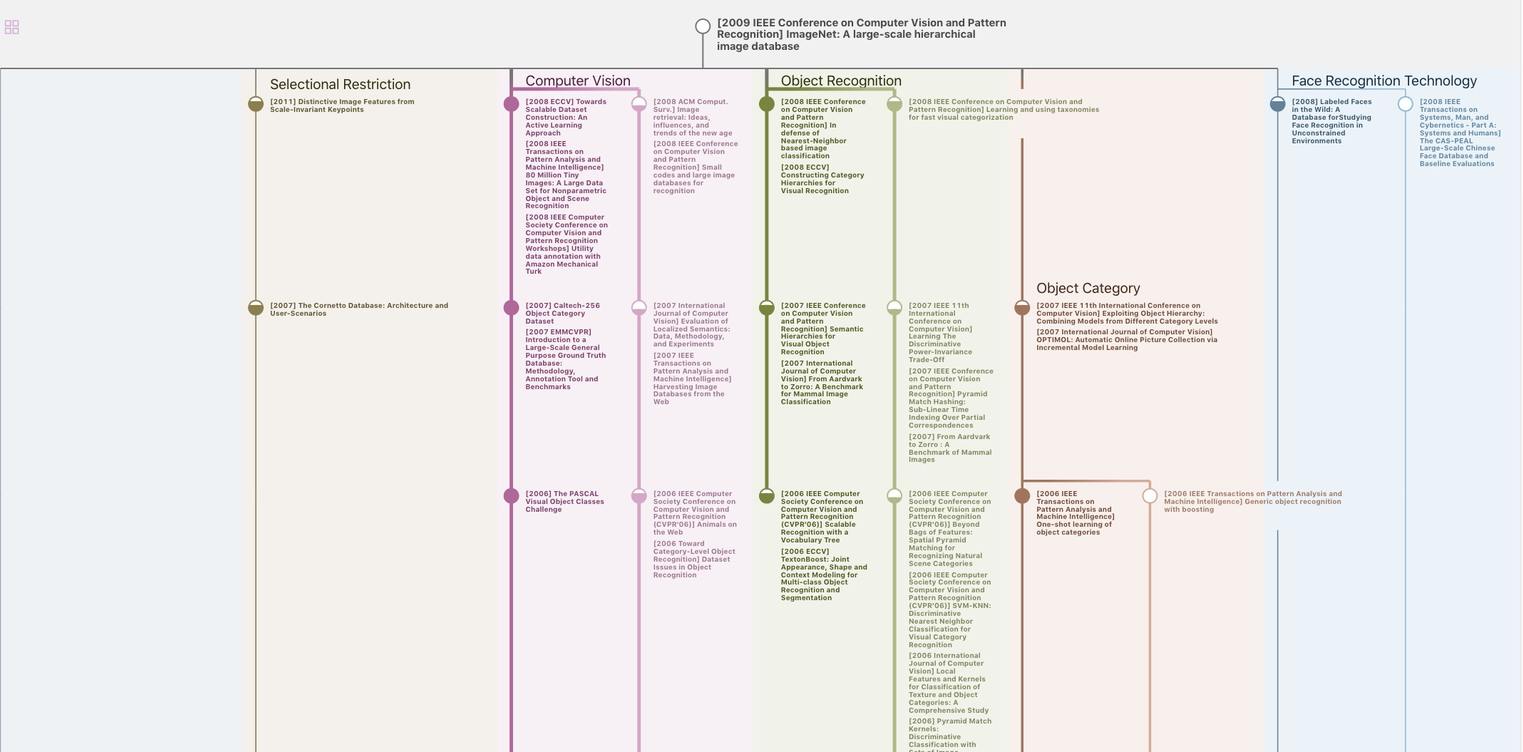
生成溯源树,研究论文发展脉络
Chat Paper
正在生成论文摘要