Joint Intent Detection Model for Task-oriented Human-computer Dialogue System using Asynchronous Training
ACM Transactions on Asian and Low-Resource Language Information Processing(2022)
摘要
How to accurately understand low-resource languages is the core of the task-oriented human-computer dialogue system. Language understanding consists of two sub-tasks, i.e., intent detection and slot filling. Intent detection still faces challenges due to semantic ambiguity and implicit intentions with users’ input. Moreover, separately modeling intent detection and slot filling significantly decrease the correctness and relevance between questions and answers. To address these issues, we propose a joint intent detection method using asynchronous training strategy. The proposed method firstly encodes local text information extracted by CNN and relationship information among words emphasized by attention structure. Later, a joint intent detection model with asynchronous training strategy is proposed by either fusing hidden states of intent detection and slot filling layers, or adopting the key information to fine-tune the whole network, greatly increasing the relevance of intent detection and slot filling subtasks. The accuracy achieved by the proposed method tested on an open-source airline travel dataset and a self-collected electricity service dataset, i.e., ATIS and ECSF, are 97.49% and 89.68% respectively, which proves the effectiveness of joint learning and asynchronous training.
更多查看译文
关键词
joint intent detection model,dialogue,asynchronous training,task-oriented,human-computer
AI 理解论文
溯源树
样例
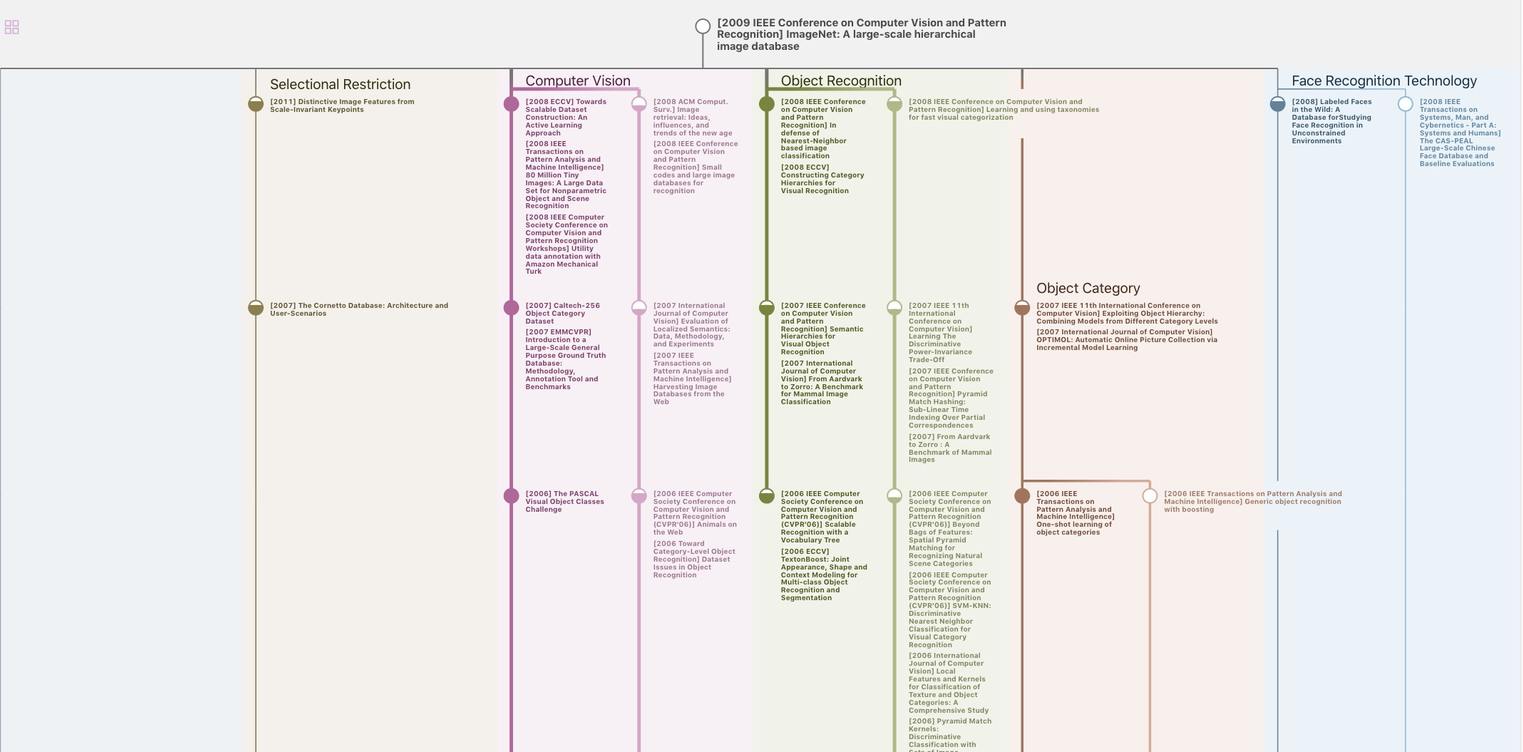
生成溯源树,研究论文发展脉络
Chat Paper
正在生成论文摘要