RGVisNet: A Hybrid Retrieval-Generation Neural Framework Towards Automatic Data Visualization Generation
KDD '22: Proceedings of the 28th ACM SIGKDD Conference on Knowledge Discovery and Data Mining(2022)
摘要
Recent years have witnessed the burgeoning of data visualization (DV) systems in both the research and the industrial communities since they provide vivid and powerful tools to convey the insights behind the massive data. A necessary step to visualize data is through creating suitable specifications in some declarative visualization languages (DVLs, e.g., Vega-Lite, ECharts). Due to the steep learning curve of mastering DVLs, automatically generating DVs via natural language questions, or text-to-vis, has been proposed and received great attention. However, existing neural network-based text-to-vis models, such as Seq2Vis or ncNet, usually generate DVs from scratch, limiting their performance due to the complex nature of this problem. Inspired by how developers reuse previously validated source code snippets from code search engines or a large-scale codebase when they conduct software development, we provide a novel hybrid retrieval-generation framework named RGVisNet for text-to-vis. It retrieves the most relevant DV query candidate as a prototype from the DV query codebase, and then revises the prototype to generate the desired DV query. Specifically, the DV query retrieval model is a neural ranking model which employs a schema-aware encoder for the NL question, and a GNN-based DV query encoder to capture the structure information of a DV query. At the same time, the DV query revision model shares the same structure and parameters of the encoders, and employs a DV grammar-aware decoder to reuse the retrieved prototype. Experimental evaluation on the public NVBench dataset validates that RGVisNet can significantly outperform existing generative text-to-vis models such as ncNet, by up to 74.28% relative improvement in terms of overall accuracy. To the best of our knowledge, RGVisNet is the first framework that seamlessly integrates the retrieval- with the generative-based approach for the text-to-vis task.
更多查看译文
AI 理解论文
溯源树
样例
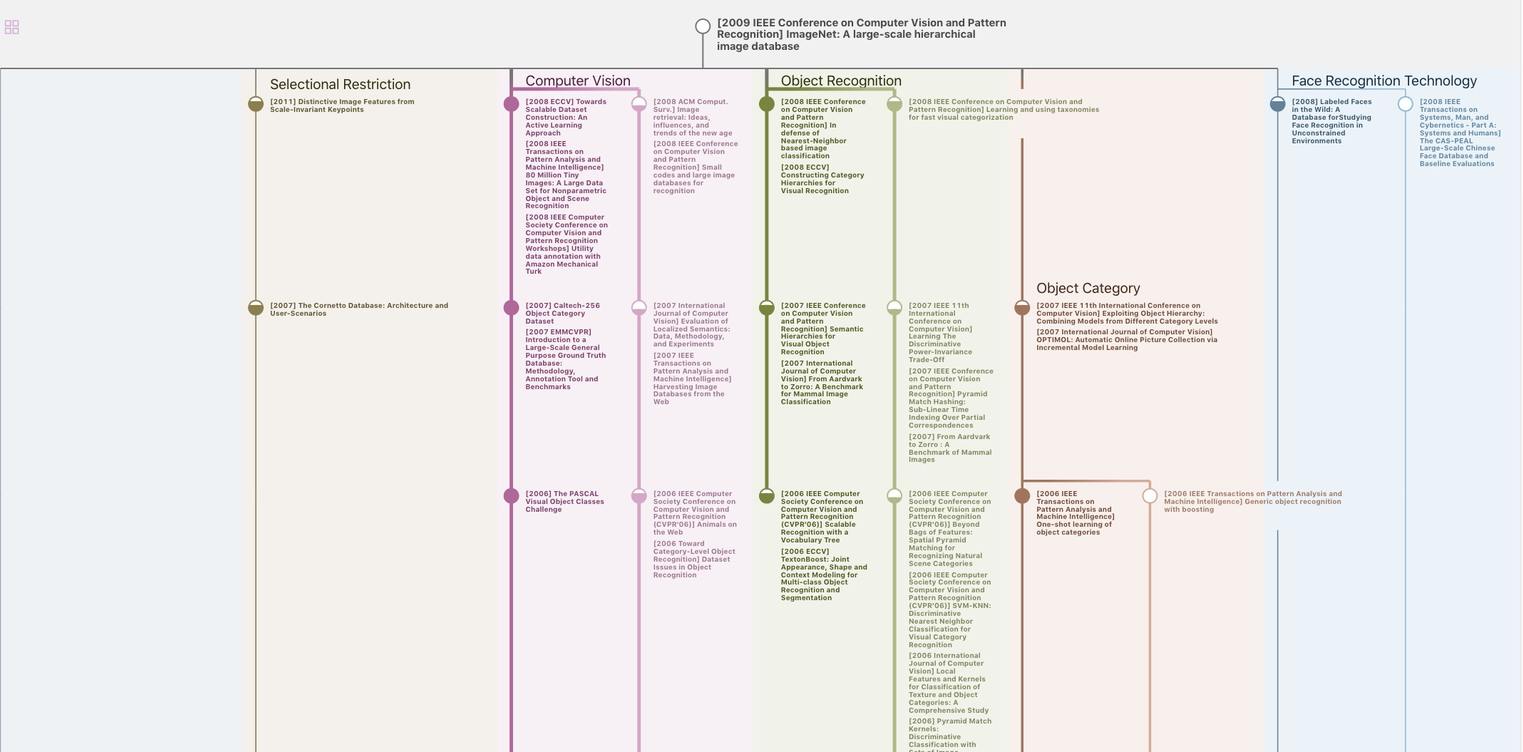
生成溯源树,研究论文发展脉络
Chat Paper
正在生成论文摘要